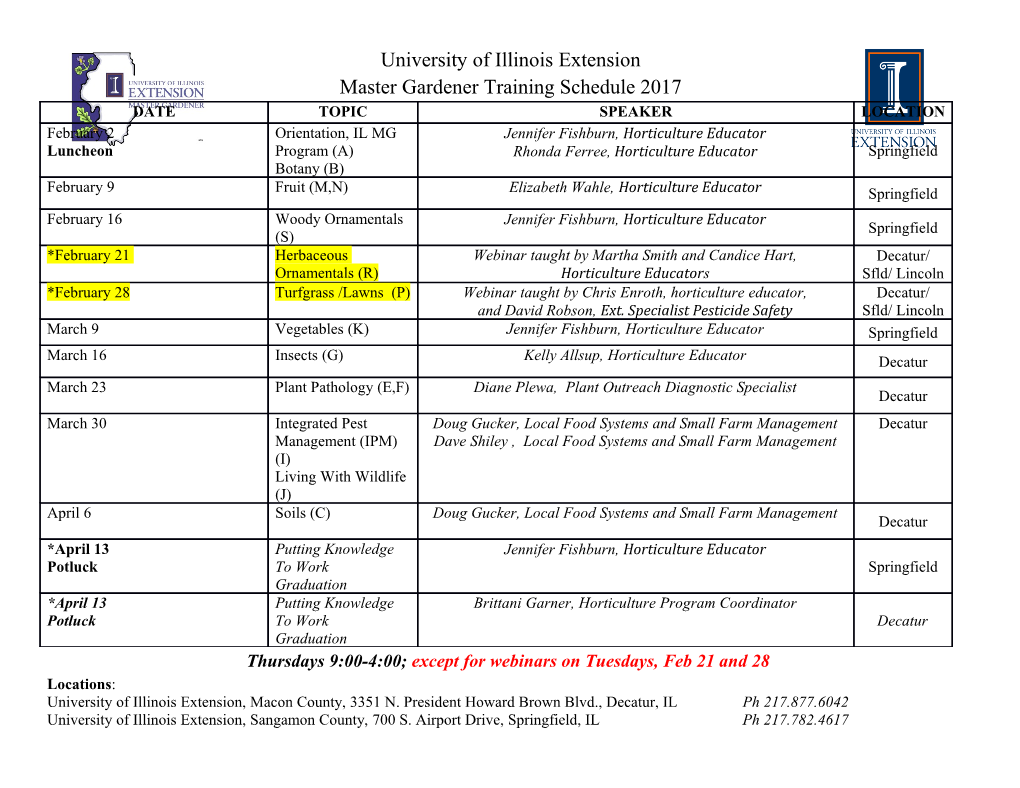
Statistical Science 2006, Vol. 21, No. 4, 501–513 DOI: 10.1214/088342306000000330 c Institute of Mathematical Statistics, 2006 Threshold Regression for Survival Analysis: Modeling Event Times by a Stochastic Process Reaching a Boundary Mei-Ling Ting Lee and G. A. Whitmore Abstract. Many researchers have investigated first hitting times as models for survival data. First hitting times arise naturally in many types of stochastic processes, ranging from Wiener processes to Markov chains. In a survival context, the state of the underlying process repre- sents the strength of an item or the health of an individual. The item fails or the individual experiences a clinical endpoint when the process reaches an adverse threshold state for the first time. The time scale can be calendar time or some other operational measure of degradation or disease progression. In many applications, the process is latent (i.e., unobservable). Threshold regression refers to first-hitting-time models with regression structures that accommodate covariate data. The pa- rameters of the process, threshold state and time scale may depend on the covariates. This paper reviews aspects of this topic and discusses fruitful avenues for future research. Key words and phrases: Accelerated testing, calendar time, compet- ing risk, cure rate, duration, environmental studies, first hitting time, gamma process, lifetime, latent variable models, maximum likelihood, operational time, occupational exposure, Ornstein–Uhlenbeck process, Poisson process, running time, stochastic process, stopping time, sur- vival analysis, threshold regression, time-to-event, Wiener diffusion pro- cess. 1. INTRODUCTION a stochastic process, which may be latent or observ- arXiv:0708.0346v1 [stat.ME] 2 Aug 2007 able. FHT models have a long history of application Many types of lifetime, duration or time-to-event in diverse fields, including medicine, environmental data may be interpreted as first hitting times (FHT’s) science, engineering, business, economics and soci- of a boundary or threshold state by sample paths of ology. FHT models may describe the length of a hospital stay, the survival time of a transplant pa- Mei-Ling Ting Lee is Professor and Chair, Division of tient, the onset time for a cancer induced by occu- Biostatistics, School of Public Health, Ohio State University, Columbus, Ohio 43210, USA e-mail: pational exposure, the failure time of an engineering [email protected]. G. A. Whitmore is Professor, system, the depletion time of an inventory, the sur- Management Science, Desautels Faculty of vival time of a new business, the transition time for Management, McGill University, Montreal, Quebec, a stock price change and the length of a marriage. Canada H3A 165 e-mail: [email protected]. Relevant articles, covering theory and application, appear in both the lifetime data and reliability lit- This is an electronic reprint of the original article published by the Institute of Mathematical Statistics in eratures. These FHT models are gradually becom- Statistical Science, 2006, Vol. 21, No. 4, 501–513. This ing widely adopted because of their conceptual ap- reprint differs from the original in pagination and peal, realism and applicability. Recently, interest in typographic detail. them has deepened and spread, and exciting new 1 2 M.-L. T. LEE AND G. A. WHITMORE areas of application are being encountered. The po- for the process and, therefore, the FHT is usually tential applications require new conceptual view- a stopping time in the formal sense of that term in points, theoretical advances, analytical techniques stochastic process theory. Note that when the parent and methodological extensions to which the discus- process is latent, there is no direct way of observing sion returns later. the FHT event in the state space of the process. To make FHT models truly valuable in applica- In some versions of the FHT model, there is no tions, they must be capable of extension to include guarantee that process X(t) will reach the bound- regression structures. Regression structures allow the ary set , so P (S< ){< 1.} We will let S = de- effects of covariates to explain the inherent disper- note theB absence of a∞ finite hitting time with P∞(S = sion of the data, thereby taking account of vari- ) = 1 P (S< ). The later discussion will show ability and sharpening inferences. Regression struc- situations∞ − where∞ this condition is plausible and a tures also provide scientific insights into potential desirable model feature. The basic FHT model (1) causal roles of covariates in the underlying processes, assumes that is fixed in time. In some applica- boundary sets and time scales. This article reviews tions, however,B it varies with time, that is, (t). This aspects of FHT models and is concerned especially variation may be deterministic or follow aB stochastic with regression structures for FHT models, which process. will be referred to as threshold regression, or TR An exhaustive review of the first-hitting-time lit- for short. The word “threshold” refers to the fact erature is impossible within the confines of a single that the FHT is triggered by the underlying process article. Eaton and Whitmore (1977) discuss FHT’s reaching a threshold state within a boundary set, as as a general model for hospital stay. Aalen and described in more detail in the next section. Gjessing (2001) provide an excellent overview of much of this subject. Likewise, Lawless (2003) gives a com- 2. THE FIRST-HITTING-TIME (FHT) MODEL plete and compact summary of theory, models and methods (see Section 11.5, pages 518–523). Lee and A first-hitting-time (FHT) model has two basic Whitmore (2004) also provide an overview of first- components: (1) a parent stochastic process X(t),t hitting-time models for survival and time-to-event ,x with initial value X(0) = x , where{ is∈ 0 data. We will make numerous references to selected theT time∈ X } space and is the state space of theT pro- work as we proceed. There is a huge literature deal- cess and (2) a boundaryX set , where . We ing with theoretical and mathematical aspects of shall refer to the boundary setB as aB⊂X boundary, FHT models that we will not attempt to review or barrier or threshold, depending onB which is most de- incorporate. We also will not cover random growth scriptive or conventional in the context. The process curve models, such as those of Carey and Koenig X(t) may have a variety of properties, such as one (1991) and Lu and Meeker (1993), which have an or{ many} dimensions, the Markov property, continu- FHT interpretation but where the only randomness ous or discrete states, and monotonic sample paths. at the level of the individual parent process is con- Whether the sample path of the parent process is fined to a noise factor. The large literature on linear observable or latent (unobservable) is an important and nonlinear regression methods for survival data distinguishing characteristic of the FHT model. La- and reliability where the underlying models have no tent processes are the most common by far. The central FHT motivation (such as accelerated failure boundary set may also have different features as time and proportional hazards models) is also not will be illustratedB in later examples. covered. Taking the initial value X(0) = x0 of the process to lie outside the boundary set , the first hitting time of is the random variableBS defined as 3. EXAMPLES OF FIRST-HITTING-TIME B MODELS (1) S = inf t : X(t) . { ∈ B} The parent stochastic processes may take many Thus, the first hitting time is the time when the forms, from Wiener processes to Markov chains. Like- stochastic process first encounters set . We refer wise, the nature of the boundary state may vary to the state first encountered in the boundaryB set widely—for example, a fixed threshold in a Wiener by the process, that is, X(S) , as the threshold process or an absorbing state in a Markov chain. state. The boundary set defines∈B a stopping condition As the preceding description of a first-hitting-time THRESHOLD REGRESSION MODELS 3 model is quite abstract, we now list a few basic ex- (1995), Whitmore and Schenkelberg (1997) and Hor- amples to illustrate the variety encountered in ap- rocks and Thompson (2004), to name a few. Onar plications. and Padgett (2000) and Padgett and Tomlinson (2004) 1. Bernoulli process and negative binomial first extend the Wiener diffusion model to an acceler- hitting time. The number of trials S required to ated testing context. Pettit and Young (1999) set reach the mth success in a Bernoulli process Bt,t = the model in a Bayesian context. As illustrated later, 1, 2,... has a negative binomial distribution{ with the inverse Gaussian distribution has a closed-form parameters} m and p, where p is the success prob- probability density function (p.d.f.) and a compu- ability on each trial. To give this setup our stan- tationally simple cumulative distribution function dard representation, we consider a parent process (c.d.f.). Their formulas vary slightly depending on Xt,t = 0, 1, 2,... with initial value X = x = m whether the parent process is defined as rising or { } 0 0 and let Xt = x0 Bt,t = 1, 2,..., where Bt is the falling to hit the relevant boundary. preceding Bernoulli− process. The first hitting{ } time 4. Gamma process and inverse gamma first hitting time. Consider a parent process X(t),t 0 with is the first Bernoulli trial t = S for which Xt equals { ≥ } 0. The number of rocket launches required to get m initial value X(0) = x0 > 0. Let X(t)= x0 Z(t) where Z(t),t 0 is a gamma process with− scale satellites in orbit is a simple example of this FHT { ≥ } model.
Details
-
File Typepdf
-
Upload Time-
-
Content LanguagesEnglish
-
Upload UserAnonymous/Not logged-in
-
File Pages14 Page
-
File Size-