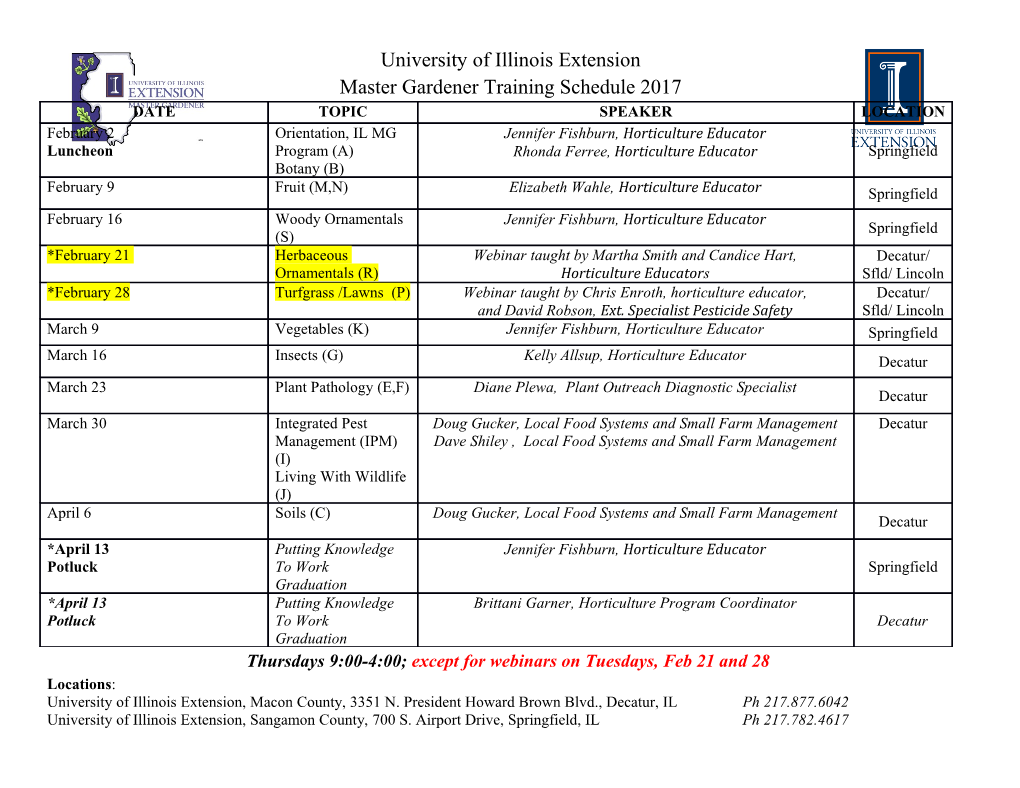
Categorical Distributions in Natural Language Processing Version 0.1 MURAWAKI Yugo 12 May 2016 MURAWAKI Yugo Categorical Distributions in NLP 1 / 34 Categorical distribution Suppose random variable x takes one of K values. x is generated according to categorical distribution Cat(θ), where θ = (0:1; 0:6; 0:3): RYG In many task settings, we do not know the true θ and need to infer it from observed data x = (x1; ··· ; xN). Once we infer θ, we often want to predict new variable x0. NOTE: In Bayesian settings, θ is usually integrated out and x0 is predicted directly from x. MURAWAKI Yugo Categorical Distributions in NLP 2 / 34 Categorical distributions are a building block of natural language models N-gram language model (predicting the next word) POS tagging based on a Hidden Markov Model (HMM) Probabilistic context-free grammar (PCFG) Topic model (Latent Dirichlet Allocation (LDA)) MURAWAKI Yugo Categorical Distributions in NLP 3 / 34 Example: HMM-based POS tagging BOS DT NN VBZ VBN EOS the sun has risen Let K be the number of POS tags and V be the vocabulary size (ignore BOS and EOS for simplicity). The transition probabilities can be computed using K categorical θTRANS; θTRANS; ··· distributions ( DT NN ), with the dimension K. θTRANS = : ; : ; : ; ··· DT (0 21 0 27 0 09 ) NN NNS ADJ Similarly, the emission probabilities can be computed using K θEMIT; θEMIT; ··· categorical distributions ( DT NN ), with the dimension V. θEMIT = : ; : ; : ; ··· NN (0 012 0 002 0 005 ) sun rose cat MURAWAKI Yugo Categorical Distributions in NLP 4 / 34 Outline Categorical and multinomial distributions Conjugacy and posterior predictive distribution LDA (Latent Dirichlet Applocation) as an application Gibbs sampling for inference MURAWAKI Yugo Categorical Distributions in NLP 5 / 34 Categorical distribution: 1 observation Suppose θ is known. Th probability of generating random variable x 2 f1; ··· ; Kg is p(x = kjθ) = θk: P ≤ θ ≤ K θ = 0 k 1 and k=1 k 1. For example, if θ = (0:1; 0:6; 0:3), then p(x = 2jθ) = θ2 = 0:6. MURAWAKI Yugo Categorical Distributions in NLP 6 / 34 Categorical distribution: N observations The probability of generating a sequence of random variables with length N, x = (x1; ··· ; xN), is YN YK jθ = θ = θnk ; p(x ) xi k i=1 k=1 whereP nk is the number of times value k is observed in x K = ( k=1 nk N). NOTE: p(xjθ) does not depend on the ordering of x but only on the total number of observed values in the sequence (sufficient statistics). MURAWAKI Yugo Categorical Distributions in NLP 7 / 34 Multinomial distribution: the probability of counts Replacing x = (x1; ··· ; xN) with the counts n = (n1; ··· ; nK), we obtain the multinomial distribution: ! YK N Multi(njθ) = θnk n ··· n k 1 k k=1 YK N! = θnk n ! ··· n ! k 1 k k=1 YK Γ(N + 1) = Q θnk : K Γ + k k=1 (nk 1) k=1 Note Γ(n) = (n − 1)!. The second term is the same as p(xjθ). The first term is the combinatorial number of x mapped to n. NOTE: In NLP, the categorical distribution is often called a multinomial distribution. MURAWAKI Yugo Categorical Distributions in NLP 8 / 34 Estimating θ from N observations If θ is unknown, we may want to infer it from x. First, the likelihood function is defined as follows: L(θ; x) = Cat(xjθ): In maximum likelihood estimation (MLE), we estimate θML that satisfies: θML = argmax L(θ; x) = argmax Cat(xjθ): θ θ It has the following analytical solution (use the method of Lagrange multipliers): θML = nk : k N MURAWAKI Yugo Categorical Distributions in NLP 9 / 34 A problem with MLE = θML = If nk 0, then k 0 (zero frequency problem). Would larger observed data fix the problem? Not necessarily. Natural language symbols usually follow a power low (cf. Zipf’s law). There are always low-frequency symbols. MURAWAKI Yugo Categorical Distributions in NLP 10 / 34 Bayes’ theorem Now we introduce p(θ), a prior distribution over θ. A prior distribution is an (arbitrary) distribution that expresses our beliefs about θ. Bayes’ theorem: p(xjθ)p(θ) p(θjx) = p(x) / p(xjθ)p(θ): p(θjx) is a posterior distribution, p(xjθ) is a likelihood, and p(θ) is a prior distribution. p(θjx) can be interpreted as the distribution of θ after observing data. MURAWAKI Yugo Categorical Distributions in NLP 11 / 34 A prior for categorical distributions For a categorical distribution, we usually set the Dirichlet distribution Dir(θjα) as its prior becaues it has nice analytical properties. p(θjx; α) / Cat(xjθ)Dir(θjα); where YK Γ(A) α − Dir(θjα) = θ k 1; Γ(α ) ··· Γ(α ) k 1 K k=1 P α = α ; ··· ; α α > = K α α where ( 1 K), k 0, and A k=1 k. is a parameter given a priori (hyperparameter). We usually set αi = α j. αk can be a real number (e.g. 1:5). Note that the Dirichlet distribution resembles a categorical distribution: YK jθ = θnk : Cat(x ) k k=1 MURAWAKI Yugo Categorical Distributions in NLP 12 / 34 Posterior distribution Posterior distribution p(θjx; α) / Cat(xjθ)Dir(θjα) is (proof omitted): YK Γ(N + A) +α − p(θjx; α) = θnk k 1 Γ(n + α ) ··· Γ(n + α ) k 1 1 K K k=1 = Dir(θjn + α): The posterior has the same form as the prior. The parameter α is replaced with n + α. This property is called conjugacy. MURAWAKI Yugo Categorical Distributions in NLP 13 / 34 Maximum a posteriori (MAP) estimatoin Maximum a posteriori (MAP) estimation employs θMAP that maximizes the posterior probability: θMAP = argmax p(θjx) θ = argmax p(xjθ)p(θ): θ MAP For Dirichlet-categorical parameter θ = argmaxθ Dir(θjn + α), the solution is n + α − 1 n + α − 1 θMAP = P k k = k k : k K + α − N + A − K k=1(nk k 1) θML = nk α − Compare it with ML estimate k N . The additional term k 1 is used to smooth the esimate (additive smoothing). MURAWAKI Yugo Categorical Distributions in NLP 14 / 34 Marginal likelihood / posterior predictive distribution Instead of using one estimate of θ, we now consider all possible values of θ. To do so, we integrate out θ to obtain marginal likelihood: Z p(x) = p(xjθ)p(θ) dθ: θ The probability of generating new variable x0 given x (predictive probability) is: Z p(x0jx) = p(x0jθ)p(θjx) dθ Zθ p(xjθ)p(θ) = p(x0jθ) dθ θ p(x) Thanks to conjugacy, we have analytical solutions for Dirichlet-categorical (next slide). MURAWAKI Yugo Categorical Distributions in NLP 15 / 34 Marginal likelihood for Dirichlet-categorical Z p(xjα) = Cat(xjθ)Dir(θjα) dθ θ 0 1 0 1 Z BYK C B YK C B C B Γ(A) α − C = B θnk C B θ k 1C θ @ k A @ k A d θ Γ(α ) ··· Γ(α ) k=1 1 K k=1 Z YK Γ(A) +α − = θnk k 1 dθ Γ α ··· Γ α k ( 1) ( K) θ = Q k 1 K Γ(A) Γ(nk + αk) = Q k=1 K Γ α Γ(N + A) k=1 ( k) Γ YK Γ + α = (A) (nk k) Γ(N + A) Γ(α ) k=1 k MURAWAKI Yugo Categorical Distributions in NLP 16 / 34 Posterior predictive for Dirichlet-categorical The probability of generating x0 after observing x is p(x0 = k0; xjα) p(x0 = k0jx; α) = p(xjα) Q 0 Γ(A) K Γ(nk+I(k=k )+αk) Γ(N+1+A) k=1 Γ(α ) = Q k Γ(A) K Γ(nk+αk) Γ(N+A) k=1 Γ(αk) Γ + Γ 0 + + α 0 = (N A) (nk 1 k ) Γ(N + 1 + A) Γ(nk0 + αk0 ) 0 + α 0 = nk k ; N + A where I(statement) is an indicator function. It gives 1 if statement is true; 0 otherwise. In analogy with nk (the count of observations), αk is called a pseudo-count. MURAWAKI Yugo Categorical Distributions in NLP 17 / 34 Sequential updates of x Let x1:N = (x1; ··· ; xN). p(x1:Njα) is the product of the probabilities of generating x one by one. p(x1:Njα) = p(xNjx1:N−1; α)p(x1:N−1jα) + α nxN (x1:N−1) xN = p(x − jα) N − 1 + A 1:N 1 + α nxN (x1:N−1) xN = p(x − jx − ; α)p(x − jα) N − 1 + A N 1 1:N 2 1:N 2 n (x − ) + α n (x − ) + α = xN 1:N 1 xN xN−1 1:N 2 xN−1 jα − + − + p(x1:N−2 ) Q NQ1 A N 2 A K nk(x1:N ) = i − 1 + αk = k 1Q i=1 N − + i=1 i 1 A Γ YK Γ + α = (A) (nk(x1:N) k); Γ(N + A) Γ(α ) k=1 k where nk(x) is the number of times k appears in x. MURAWAKI Yugo Categorical Distributions in NLP 18 / 34 Exchangeability As stated earlier, p(xjθ) does not depend on the ordering of x but on the total number of times each value is observed in the sequence (sufficient statistics). Let us create x0 by swapping an arbitrary pair of variables xi; x j in x. Since the ordering does not matter, the following is hold: p(x0jα) = p(xjα) This property is called exchangeability. As we will see later, exchangeability makes inference easy. MURAWAKI Yugo Categorical Distributions in NLP 19 / 34 Example: Sequential updates of x Suppose random variable x takes one of three values: R, Y, G.
Details
-
File Typepdf
-
Upload Time-
-
Content LanguagesEnglish
-
Upload UserAnonymous/Not logged-in
-
File Pages34 Page
-
File Size-