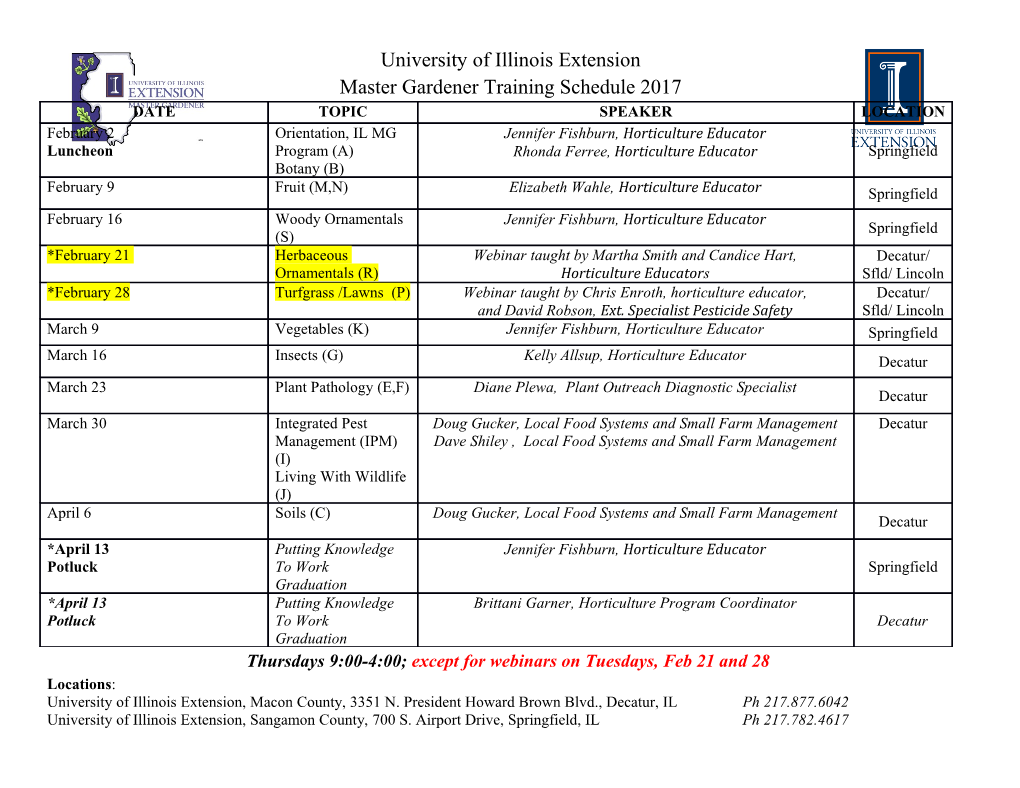
Building Representative Corpora from Illiterate Communities: A Review of Challenges and Mitigation Strategies for Developing Countries Stephanie Hirmer1, Alycia Leonard1, Josephine Tumwesige2, Costanza Conforti2;3 1Energy and Power Group, University of Oxford 2Rural Senses Ltd. 3Language Technology Lab, University of Cambridge [email protected] Abstract areas where the bulk of the population lives (Roser Most well-established data collection methods and Ortiz-Ospina(2016), Figure1). As a conse- currently adopted in NLP depend on the as- quence, common data collection techniques – de- sumption of speaker literacy. Consequently, signed for use in HICs – fail to capture data from a the collected corpora largely fail to repre- vast portion of the population when applied to LICs. sent swathes of the global population, which Such techniques include, for example, crowdsourc- tend to be some of the most vulnerable and ing (Packham, 2016), scraping social media (Le marginalised people in society, and often live et al., 2016) or other websites (Roy et al., 2020), in rural developing areas. Such underrepre- sented groups are thus not only ignored when collecting articles from local newspapers (Mari- making modeling and system design decisions, vate et al., 2020), or interviewing experts from in- but also prevented from benefiting from de- ternational organizations (Friedman et al., 2017). velopment outcomes achieved through data- While these techniques are important to easily build driven NLP. This paper aims to address the large corpora, they implicitly rely on the above- under-representation of illiterate communities mentioned assumptions (i.e. internet access and in NLP corpora: we identify potential biases literacy), and might result in demographic misrep- and ethical issues that might arise when col- resentation (Hovy and Spruit, 2016). In this pa- lecting data from rural communities with high illiteracy rates in Low-Income Countries, and per, we make a first step towards addressing how propose a set of practical mitigation strategies to build representative corpora in LICs from il- to help future work. literate speakers. We believe that this is a cur- rently unaddressed topic within NLP research. It 1 Introduction aligns with previous work investigating sources The exponentially increasing popularity of super- of bias resulting from the under-representation vised Machine Learning (ML) in the past decade of specific demographic groups in NLP corpora has made the availability of data crucial to the (such as women (Hovy, 2015), youth (Hovy and development of the Natural Language Processing Søgaard, 2015), or ethnic minorities (Groenwold (NLP) field. As a result, much NLP research has et al., 2020)). In this paper, we make the follow- focused on developing rigorous processes for col- ing contributions: (i) we introduce the challenges lecting large corpora suitable for training ML sys- of collecting data from illiterate speakers in x2; arXiv:2102.02841v1 [cs.CL] 4 Feb 2021 tems. We observe, however, that many best prac- (ii) we define various possible sources of biases tices for quality data collection make two implicit and ethical issues which can contribute to low data assumptions: that speakers have internet access quality we define various possible sources of bi- and that they are literate (i.e. able to read and ases and ethical issues which can contribute to low often write text effortlessly1). Such assumptions data quality we define various possible sources of might be reasonable in the context of most High- biases and ethical issues which can contribute to Income Countries (HICs) (UNESCO, 2018). How- low data quality x3; finally, (iii) drawing on years ever, in Low-Income Countries (LICs), and espe- of experience in data collection in LICs, we outline cially in sub-Saharan Africa (SSA), such assump- practical countermeasures to address these issues tions may not hold, particularly in rural developing in x4. 1For example, input from speakers is often taken in writing, in response to a written stimulus which must be read. (a) Adult literacy (% ages 15+, UN- (b) Urban population (% total, UN- (c) Internet usage (% of total, ITU ESCO(2018)) DESA(2018)) (2019)) Figure 1: Literacy, urban population, and internet usage in African countries. Note that countries with more rural populations tend to have less literacy and less internet users. These countries are likely to be under-represented in corpora generated using common data collection methods that assume literacy and internet access (Grey: no data). 2 Listening to the Illiterate: What Makes description of best practices for data collection re- it Challenging? mains a notable research gap. In recent years, developing corpora that encom- 3 Definitions and Challenges passes as many human languages as possible has been recognised as important in the NLP commu- Guided by research in medicine (Pannucci and nity. In this context, widely translated texts (such Wilkins, 2010), sociology (Berk, 1983), and psy- as the Bible (Mueller et al., 2020) or the Human chology (Gilovich et al., 2002), NLP has experi- Rights declaration (King, 2015)) are often used as enced increasing interest in ethics and bias mitiga- a source of data. However, these texts tend to be tion to minimise unintentional demographic mis- quite short and domain-specific. Moreover, while representation and harm (Hovy and Spruit, 2016). the Internet constitutes a powerful data collection While there are many stages where bias may enter tool which is more representative of real language the NLP pipeline (Shah et al., 2019), we focus on use than the previously-mentioned texts, it excludes those pertinent to data collection from rural illit- illiterate communities, as well as speakers which erate communities in LICs, leaving the study of lack reliable internet access (as is often the case in biases in model development for future work2. rural developing settings, Figure1). Given the obstacles to using these common lan- 3.1 Data Collection Biases guage data collection methods in LIC contexts, the NLP community can learn from methodologies Biases in data collection are inevitable (Marshall, adopted in other fields. Researchers from fields 1996) but can be minimised when known to the such as sustainable development (SD, Gleitsmann researcher (Trembley, 1957). We identify various et al.(2007)), African studies (Adams, 2014), and biases that can emerge when collecting language ethnology (Skinner et al., 2013), tend to rely heav- data in rural developing contexts, which fall under ily on qualitative data from oral interviews, tran- three broad categories: sampling, observer, and re- scribed verbatim. Collecting such data in rural de- sponse bias. Sampling determines who is studied, veloping areas is considerably more difficult than the interviewer (or observer) determines what in- in developed or urban contexts. In addition to high formation is sought and how it is interpreted, and illiteracy levels, researchers face challenges such the interviewee (or respondent) determines which as seasonal roads and low population densities. To information is revealed (Woodhouse, 1998). These our knowledge, there are very few NLP works categories span the entire data collection process which explicitly focus on building corpora from and can affect the quality and quantity of language rural and illiterate communities: of those works data obtained. that exist, some present clear priming effect is- 2Note, this paper does not focus on a particular NLP ap- sues (Abraham et al., 2020), while others focus plication, as once the data has been collected from illiterate on application (Conforti et al., 2020). A detailed communities it can be annotated for virtually any specific task. 3.2 Sampling or selection bias unrelated data as electricity-motivated (Hirmer and Guthrie, 2017), or omit data which contradicts their Sampling bias occurs when observations are drawn hypothesis (Peters, 2020). Using such data to train from an unrepresentative subset of the population NLP models may introduce unintentional bias to- being studied (Marshall, 1996) and applied more wards the original expectations of the researchers widely. In our context, this might arise when select- instead of accurately representing the community. ing communities from which to collect language Secondly, the interviewer’s understanding and data, or specific individuals within each commu- interpretation of the speaker’s utterances might be nity. When sampling communities, bias can be influenced by their class, culture and language. introduced if convenience is prioritized. Commu- Note that, particularly in countries without strong nities which are easier to access may not produce language standardisation policies, consistent se- language data representative of a larger area or mantic shifts can happen even between varieties group. This can be illustrated through Uganda’s spoken in neighboring regions (Gordon, 2019), refugee response, which consists of 13 settlements which may result in systematic misunderstand- (including the 2nd largest in the world) hosted in 12 ing (Sayer, 2013). For example, in the neighboring districts (UNHCR, 2020). Data collection may be Ugandan tribes of Toro and Bunyoro, the same easier in one of the older, established settlements; word omunyoro means respectively husband and however, such data cannot be generalised over the a member of the tribe. Language data collected in entire refugee response due to different cultural such contexts, if not properly handled, may contain
Details
-
File Typepdf
-
Upload Time-
-
Content LanguagesEnglish
-
Upload UserAnonymous/Not logged-in
-
File Pages14 Page
-
File Size-