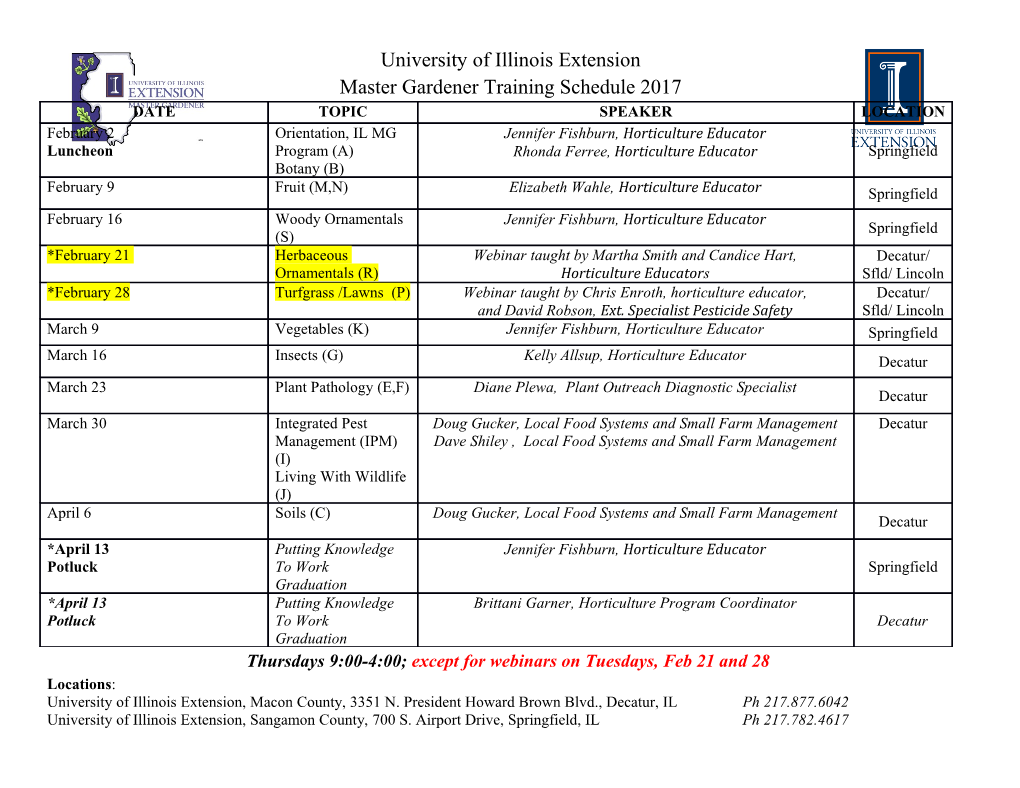
Consequences Typical processes Stationarity Diagnostics Spatial autocorrelation Advanced Quantitative Methods: Autocorrelation Jos Elkink University College Dublin February 23, 2011 Jos Elkink autocorrelation Consequences Typical processes Stationarity Diagnostics Spatial autocorrelation 1 Consequences 2 Typical processes 3 Stationarity 4 Diagnostics Plots Tests: Autocorrelation Tests: Stationarity 5 Spatial autocorrelation Jos Elkink autocorrelation Consequences Typical processes Stationarity Diagnostics Spatial autocorrelation Notation: lagged variables Instead of yi to indicate each of n observations, we will use yt to refer to each of T observations on a time-series. Jos Elkink autocorrelation Consequences Typical processes Stationarity Diagnostics Spatial autocorrelation Notation: lagged variables Instead of yi to indicate each of n observations, we will use yt to refer to each of T observations on a time-series. yt−1 refers to the lagged value, i.e. the value of variable y at time t 1, the observation just one time period before time t. − Jos Elkink autocorrelation Consequences Typical processes Stationarity Diagnostics Spatial autocorrelation Notation: lagged variables Instead of yi to indicate each of n observations, we will use yt to refer to each of T observations on a time-series. yt−1 refers to the lagged value, i.e. the value of variable y at time t 1, the observation just one time period before time t. − A lag can have any length k (k > 0), yt−k . Jos Elkink autocorrelation Consequences Typical processes Stationarity Diagnostics Spatial autocorrelation Notation: first differences The difference between yt and yt−1, or the change in variable y at time t, is called the first difference, ∆yt = yt yt− . − 1 Jos Elkink autocorrelation Consequences Typical processes Stationarity Diagnostics Spatial autocorrelation Notation: first differences The difference between yt and yt−1, or the change in variable y at time t, is called the first difference, ∆yt = yt yt− . − 1 Again, differences can have different lag lengths: ∆yt−k = yt−k yt−k− . − 1 Jos Elkink autocorrelation Consequences Typical processes Stationarity Diagnostics Spatial autocorrelation Outline 1 Consequences 2 Typical processes 3 Stationarity 4 Diagnostics Plots Tests: Autocorrelation Tests: Stationarity 5 Spatial autocorrelation Jos Elkink autocorrelation Consequences Typical processes Stationarity Diagnostics Spatial autocorrelation The problem A key assumption of (linear) regression is that observations are independent. Jos Elkink autocorrelation Consequences Typical processes Stationarity Diagnostics Spatial autocorrelation The problem A key assumption of (linear) regression is that observations are independent. Generally, in time-series or observations in space, the observations depend on each other. If GDP is high in 1999, it is likely to be high in 2000. If GDP is high in Germany, it is likely to be high in The Netherlands. Jos Elkink autocorrelation Consequences Typical processes Stationarity Diagnostics Spatial autocorrelation The problem A key assumption of (linear) regression is that observations are independent. Generally, in time-series or observations in space, the observations depend on each other. If GDP is high in 1999, it is likely to be high in 2000. If GDP is high in Germany, it is likely to be high in The Netherlands. Treating them as independent observations suggest that you have far more information than you do. Jos Elkink autocorrelation Consequences Typical processes Stationarity Diagnostics Spatial autocorrelation The problem Ignoring this autocorrelation leads to: βˆOLS unbiased but inefficient (as long as E(ε X)=0) | Jos Elkink autocorrelation Consequences Typical processes Stationarity Diagnostics Spatial autocorrelation The problem Ignoring this autocorrelation leads to: βˆOLS unbiased but inefficient (as long as E(ε X)=0) | V (βˆOLS ) may be an under- or overestimate - the F - and t-tests cannot be trusted. If the autocorrelation is positive, V (βˆOLS ) will be an underestimate. Jos Elkink autocorrelation Consequences Typical processes Stationarity Diagnostics Spatial autocorrelation The problem Ignoring this autocorrelation leads to: βˆOLS unbiased but inefficient (as long as E(ε X)=0) | V (βˆOLS ) may be an under- or overestimate - the F - and t-tests cannot be trusted. If the autocorrelation is positive, V (βˆOLS ) will be an underestimate. The residual variance is likely to be underestimated and R2 overestimated. Jos Elkink autocorrelation Consequences Typical processes Stationarity Diagnostics Spatial autocorrelation The problem Ignoring this autocorrelation leads to: βˆOLS unbiased but inefficient (as long as E(ε X)=0) | V (βˆOLS ) may be an under- or overestimate - the F - and t-tests cannot be trusted. If the autocorrelation is positive, V (βˆOLS ) will be an underestimate. The residual variance is likely to be underestimated and R2 overestimated. Risk of spurious regressions Jos Elkink autocorrelation Consequences Typical processes Stationarity Diagnostics Spatial autocorrelation Spurious regressions When two variables are uncorrelated, but nonstationary, they often lead to highly significant estimates of their correlation in“naive”linear regression. Assume: Jos Elkink autocorrelation Consequences Typical processes Stationarity Diagnostics Spatial autocorrelation Spurious regressions When two variables are uncorrelated, but nonstationary, they often lead to highly significant estimates of their correlation in“naive”linear regression. Assume: yt = yt−1 + ε1,t xt = xt−1 + ε2,t . Jos Elkink autocorrelation Consequences Typical processes Stationarity Diagnostics Spatial autocorrelation Spurious regressions When two variables are uncorrelated, but nonstationary, they often lead to highly significant estimates of their correlation in“naive”linear regression. Assume: yt = yt−1 + ε1,t xt = xt−1 + ε2,t . Then OLS estimation of: yt = α + βxt + εt will lead to a significant t-test on β. Jos Elkink autocorrelation Consequences Typical processes Stationarity Diagnostics Spatial autocorrelation Spurious regressions Sample data −10 −5 0 5 0 20 40 60 80 100 Jos Elkink autocorrelation Consequences Typical processes Stationarity Diagnostics Spatial autocorrelation Spurious regression lm(formula = y ~ x) Estimate Std. Error t value Pr(>|t|) (Intercept) -0.9646 0.3626 -2.660 0.00911 ** x -0.9207 0.1002 -9.185 6.54e-15 *** Residual standard error: 3.021 on 99 degrees of freedom Multiple R-Squared: 0.4601, Adjusted R-squared: 0.4547 F-statistic: 84.37 on 1 and 99 DF, p-value: 6.544e-15 Jos Elkink autocorrelation Consequences Typical processes Stationarity Diagnostics Spatial autocorrelation Outline 1 Consequences 2 Typical processes 3 Stationarity 4 Diagnostics Plots Tests: Autocorrelation Tests: Stationarity 5 Spatial autocorrelation Jos Elkink autocorrelation Consequences Typical processes Stationarity Diagnostics Spatial autocorrelation Time-series processes A time-series can have been generated by various different types of processes. Which process generated the data of course affects which econometric model is more appropriate to estimate its parameters. Jos Elkink autocorrelation Consequences Typical processes Stationarity Diagnostics Spatial autocorrelation Linear model The linear regression model looks like: y = µ + ε, where µ = Xβ, or, if we have no explanatory variables, µ is a constant. Jos Elkink autocorrelation Consequences Typical processes Stationarity Diagnostics Spatial autocorrelation Linear model The linear regression model looks like: y = µ + ε, where µ = Xβ, or, if we have no explanatory variables, µ is a constant. For now, we will look at the latter case, µt = µ. Jos Elkink autocorrelation Consequences Typical processes Stationarity Diagnostics Spatial autocorrelation Linear model The linear regression model looks like: y = µ + ε, where µ = Xβ, or, if we have no explanatory variables, µ is a constant. For now, we will look at the latter case, µt = µ. In the linear model, we assume ε to be an IID variable, ε N(0,σ2). ∼ Jos Elkink autocorrelation Consequences Typical processes Stationarity Diagnostics Spatial autocorrelation Moving average process In the moving average model, we replace the assumption of entirely independent residuals by assuming that the residual at time t is a weighted average between that residual and the one at t 1. − Jos Elkink autocorrelation Consequences Typical processes Stationarity Diagnostics Spatial autocorrelation Moving average process In the moving average model, we replace the assumption of entirely independent residuals by assuming that the residual at time t is a weighted average between that residual and the one at t 1. − yt = µ +(εt + φεt− ) 1 <φ< 1 1 − Jos Elkink autocorrelation Consequences Typical processes Stationarity Diagnostics Spatial autocorrelation Moving average process The above is a so-called MA(1) process, a moving average process with one lag. Jos Elkink autocorrelation Consequences Typical processes Stationarity Diagnostics Spatial autocorrelation Moving average process The above is a so-called MA(1) process, a moving average process with one lag. This model can be generalised to more lags, the MA(q) process: yt = µ +(εt + φ1εt−1 + φ2εt−2) q yt = µ +(εt + φl εt−l ) l =1 Jos Elkink autocorrelation Consequences Typical processes Stationarity Diagnostics Spatial autocorrelation Moving average process Theoretically this model can be generalised to infinitely many lags: ∞ yt = µ +(εt + φl εt−l ) l =1 Jos Elkink autocorrelation Consequences Typical processes Stationarity Diagnostics Spatial autocorrelation
Details
-
File Typepdf
-
Upload Time-
-
Content LanguagesEnglish
-
Upload UserAnonymous/Not logged-in
-
File Pages135 Page
-
File Size-