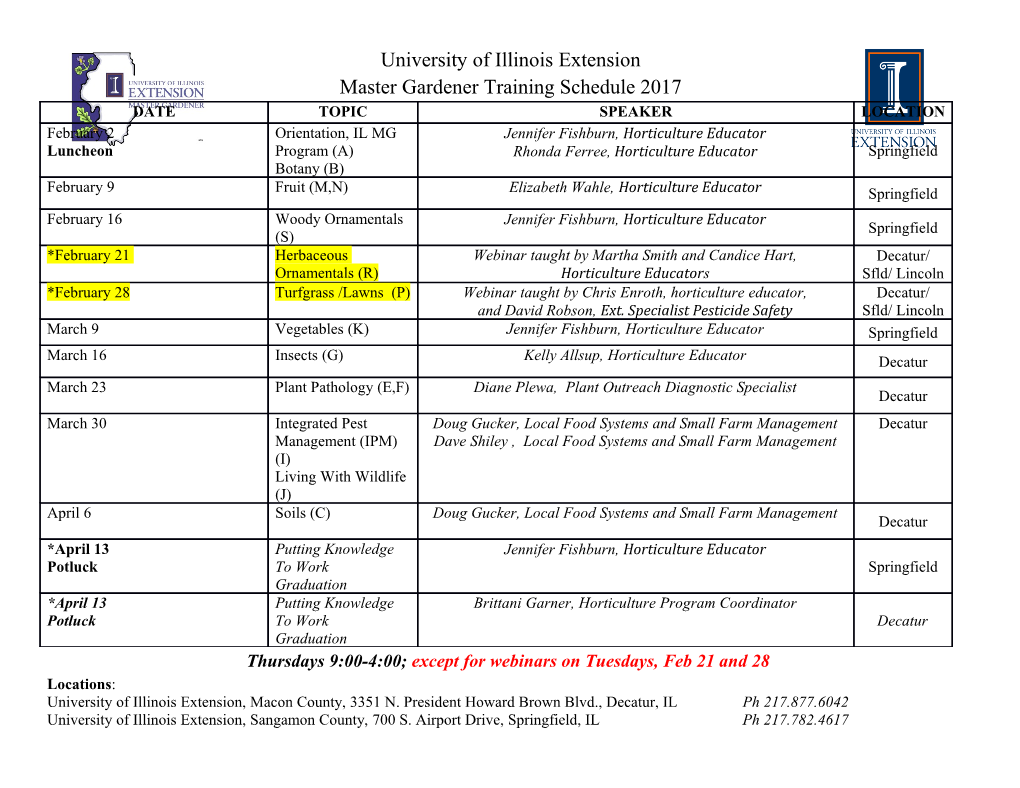
Norms and Inner Products Garrett Thomas July 21, 2018 1 About This document is part of a series of notes about math and machine learning. You are free to distribute it as you wish. The latest version can be found at http://gwthomas.github.io/notes. Please report any errors to [email protected]. 2 Norms Norms generalize the notion of length from Euclidean space. A norm on a vector space V is a function k · k : V ! R that satisfies (i) kvk ≥ 0, with equality if and only if v = 0 (ii) kαvk = jαjkvk (iii) ku + vk ≤ kuk + kvk (the triangle inequality) for all u; v 2 V and all α 2 F. A vector space endowed with a norm is called a normed vector space, or simply a normed space. An important fact about norms is that they induce metrics, giving a notion of convergence in vector spaces. Proposition 1. If a vector space V is equipped with a norm k · k : V ! R, then d(u; v) , ku − vk is a metric on V . Proof. The axioms for metrics follow directly from those for norms. If u; v; w 2 V , then (i) d(u; v) = ku − vk ≥ 0, with equality if and only if u − v = 0, i.e. u = v (ii) d(u; v) = ku − vk = k(−1)(v − u)k = j − 1jkv − uk = d(v; u) (iii) d(u; v) = ku − vk = ku − w + w − vk ≤ ku − wk + kw − vk = d(u; w) + d(w; v) so d is a metric as claimed. 1 Therefore any normed space is also a metric space. If a normed space is complete with respect to the distance metric induced by its norm, it is said to be a Banach space. The metric induced by a norm automatically has the property of translation invariance, meaning that d(u + w; v + w) = d(u; v) for any u; v; w 2 V : d(u + w; v + w) = k(u + w) − (v + w)k = ku + w − v − wk = ku − vk = d(u; v) 3 Inner products An inner product on a vector space V over F is a function h·; ·i : V × V ! F satisfying (i) hv; vi ≥ 0, with equality if and only if v = 0 (ii) Linearity in the first slot: hu + v; wi = hu; wi + hv; wi and hαu; vi = αhu; vi (iii) Conjugate symmetry: hu; vi = hv; ui for all u; v; w 2 V and all α 2 F. A vector space endowed with an inner product is called an inner product space. If F = R, the conjugate symmetry condition reduces to symmetry: hu; vi = hv; ui. Additionally, the combination of linearity and conjugate symmetry imply sesquilinearity: hu; αvi = hαv; ui = αhv; ui = αhv; ui = αhu; vi It is also worth noting that h0; vi = 0 for any v because for any u 2 V hu; vi = hu + 0; vi = hu; vi + h0; vi Proposition 2. The function hx; yi , xy is an inner product on C (considered as a vector space over C). Proof. If x; y; z 2 C and α 2 C, then 1. hz; zi = zz = jzj2 ≥ 0, with equality if and only if z = 0 2. hx + y; zi = (x + y)z = xz + yz = hx; zi + hy; zi, and hαx; yi = (αx)y = α(xy) = αhx; yi 3. hx; yi = xy = yx = hy; xi so the function is an inner product as claimed. 3.1 Orthogonality Orthogonality generalizes the notion of perpendicularity from Euclidean space. Two vectors u and v are said to be orthogonal if hu; vi = 0; we write u ? v for shorthand. A set of vectors v1; : : : ; vn is described as pairwise orthogonal (or just orthogonal for short) if vj ? vk for all j 6= k. Proposition 3. If v1; : : : ; vn are nonzero and pairwise orthogonal, then they are linearly indepen- dent. 2 Proof. Assume v1; : : : ; vn are nonzero and pairwise orthogonal, and suppose α1v1 + : : : αnvn = 0. Then for each j = 1; : : : ; n, 0 = h0; vji = hα1v1 + : : : αnvn; vji = α1hv1; vji + ··· + αnhvn; vji = αjhvj; vji Since vj 6= 0, hvj; vji > 0, so αj = 0. Hence v1; : : : ; vn are linearly independent. 4 Norms induced by inner products Any inner product induces a norm given by p kvk , hv; vi Moreover, these norms have certain special properties related to the inner product. The notation k · k is not yet justified as we have not yet shown that this is in fact a norm. We need first a couple of intermediate results, which are useful in their own right. 4.1 Pythagorean Theorem The well-known Pythagorean theorem generalizes naturally to arbitrary inner product spaces. Proposition 4. (Pythagorean theorem) If u ? v, then ku + vk2 = kuk2 + kvk2 Proof. Suppose u ? v, i.e. hu; vi = 0. Then ku + vk2 = hu + v; u + vi = hu; ui + hv; ui + hu; vi + hv; vi = kuk2 + kvk2 as claimed. It is clear that by repeatedly applying the Pythagorean theorem for two vectors, we can obtain a slight generalization: if v1; : : : ; vn are orthogonal, then 2 2 2 kv1 + ··· + vnk = kv1k + ··· + kvnk 4.2 Cauchy-Schwarz inequality The Cauchy-Schwarz inequality is one of the most widespread and useful inequalities in mathe- matics. Proposition 5. If V is an inner product space, then jhu; vij ≤ kukkvk for all u; v 2 V . Equality holds exactly when u and v are linearly dependent. Proof. If v = 0, then equality holds, for jhu; 0ij = 0 = kuk0 = kukk0k. So suppose v 6= 0. In this case we can define hu; vi w v , kvk2 3 which satisfies hu; vi hu; vi hw; u − wi = v; u − v kvk2 kvk2 ! hu; vi hu; vi = hv; ui − hv; vi kvk2 kvk2 hu; vi hv; ui = hv; ui − kvk2 kvk2 kvk2 = 0 Therefore, by the Pythagorean theorem, kuk2 = kw + u − wk2 = kwk2 + ku − wk2 2 hu; vi ≥ v kvk2 2 hu; vi 2 = kvk kvk2 jhu; vij2 = kvk2 which, after multiplying through by kvk2, yields jhu; vij2 ≤ kuk2kvk2 The claimed inequality follows by taking square roots, since both sides are nonnegative. Observe that the only inequality in the reasoning above comes from ku − wk2 ≥ 0, so equality holds if and only if hu; vi 0 = u − w = u − v kvk2 which implies that u and v are linearly dependent. Conversely if u and v are linearly dependent, then u = αv for some α 2 F, and hαv; vi αhv; vi w = v = v = αv kvk2 kvk2 giving u − w = 0, so that equality holds. We now have all the tools needed to prove that k · k is in fact a norm. Proposition 6. If V is an inner product space, then p kvk , hv; vi is a norm on V . Proof. The axioms for norms mostly follow directly from those for inner products, but the triangle inequality requires a bit of work. If u; v 2 V and α 2 F, then (i) kvk = phv; vi ≥ 0 since hv; vi ≥ 0, with equality if and only if v = 0. 4 (ii) kαvk = phαv; αvi = pααhv; vi = pjαj2hv; vi = jαjphv; vi = jαjkvk. (iii) Using the Cauchy-Schwarz inequality, ku + vk2 = hu + v; u + vi = hu; ui + hu; vi + hv; ui + hv; vi = kuk2 + hu; vi + hu; vi + kvk2 = kuk2 + 2 Rehu; vi + kvk2 ≤ kuk2 + 2jhu; vij + kvk2 ≤ kuk2 + 2kukkvk + kvk2 = (kuk + kvk)2 Taking square roots yields ku + vk ≤ kuk + kvk, since both sides are nonnegative. Hence k · k is a norm as claimed. Thus every inner product space is a normed space, and hence also a metric space. If an inner product space is complete with respect to the distance metric induced by its inner product, it is said to be a Hilbert space. 4.3 Orthonormality A set of vectors e1; : : : ; en are said to be orthonormal if they are orthogonal and have unit norm (i.e. kejk = 1 for each j). This pair of conditions can be concisely expressed as hej; eki = δjk, where δjk is the Kronecker delta. Observe that if v 6= 0, then v=kvk is a unit vector in the same \direction" as v (i.e. a positive scalar multiple of v), for v 1 = kvk = 1 kvk kvk Recall that an orthogonal set of nonzero vectors are linearly independent. Unit vectors are necessarily nonzero (since k0k = 0 6= 1), so an orthonormal set of vectors always forms a basis for its span. Not surprisingly, such a basis is referred to as an orthonormal basis. A nice property of orthonormal bases is that vectors' coefficients in terms of this basis can be computed via the inner product. Proposition 7. If e1; : : : ; en is an orthonormal basis for V , then any v 2 V can be written v = hv; e1ie1 + ··· + hv; enien Proof. Since e1; : : : ; en is a basis for V , any v 2 V has a unique expansion v = α1e1 + ··· + αnen where α1; : : : ; αn 2 F. Then for j = 1; : : : ; n, hv; eji = hα1e1 + ··· + αnen; eji = α1he1; eji + ··· + αnhen; eji = αj since hek; eji = 0 for k 6= j and hej; eji = 1. 5 The norm of vectors expressed in an orthonormal basis is also easily found, for 2 2 2 2 2 kα1e1 + ··· + αnenk = kα1e1k + ··· + kαnenk = jα1j + ··· + jαnj by application of the Pythagorean theorem. Combining this with the previous result, we see 2 2 2 kvk = jhv; e1ij + ··· + jhv; enij 4.4 The Gram-Schmidt process The Gram-Schmidt process produces an orthonormal basis for a vector space from an arbitrary basis for the space. Proposition 8. Let v1; : : : ; vn 2 V be linearly independent. Define wj , vj − hvj; e1ie1 − · · · − hvj; ej−1iej−1 wj ej , kwjk for j = 1; : : : ; n.
Details
-
File Typepdf
-
Upload Time-
-
Content LanguagesEnglish
-
Upload UserAnonymous/Not logged-in
-
File Pages11 Page
-
File Size-