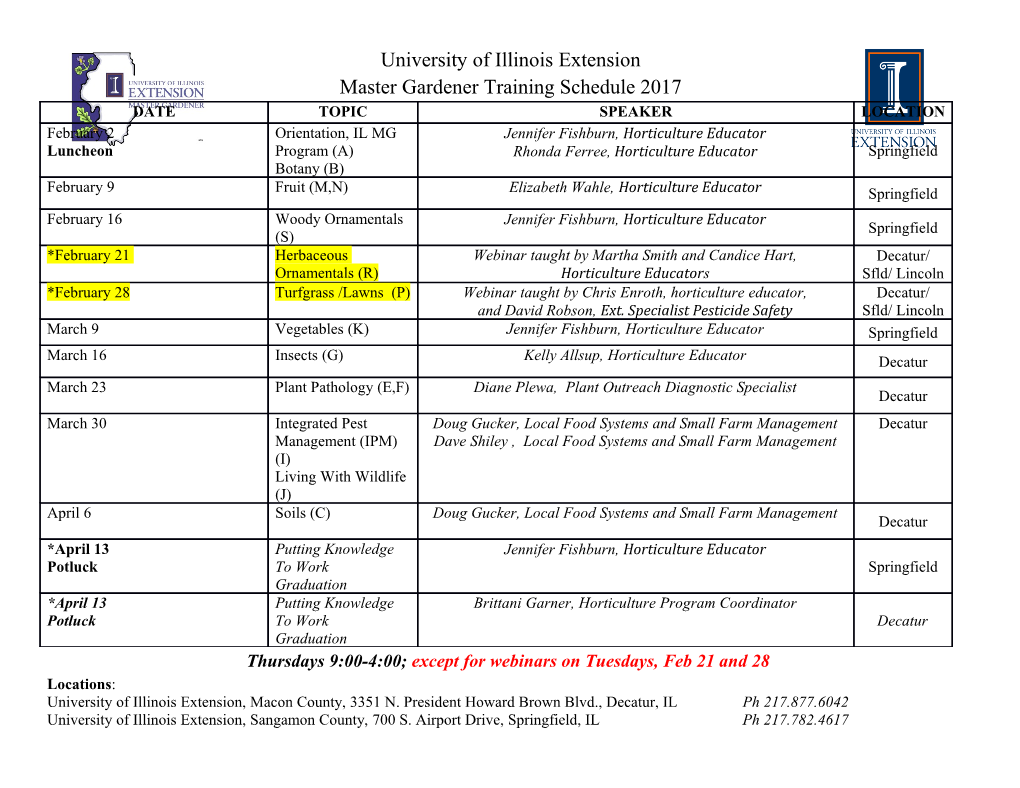
Molecular Systems Biology 7; Article number 543; doi:10.1038/msb.2011.77 Citation: Molecular Systems Biology 7: 543 & 2011 EMBO and Macmillan Publishers Limited All rights reserved 1744-4292/11 www.molecularsystemsbiology.com PERSPECTIVE Controlled vocabularies and semantics in systems biology Me´lanie Courtot1,19, Nick Juty2,19, Christian Knu¨pfer3,19, provides semantic information about the model compo- Dagmar Waltemath4,19, Anna Zhukova2,19, Andreas Dra¨ger5, nents. The Kinetic Simulation Algorithm Ontology (KiSAO) Michel Dumontier6, Andrew Finney7, Martin Golebiewski8, supplies information about existing algorithms available Janna Hastings2, Stefan Hoops9, Sarah Keating2, Douglas B for the simulation of systems biology models, their characterization and interrelationships. The Terminology Kell10,11, Samuel Kerrien2, James Lawson12, Allyson Lister13,14, for the Description of Dynamics (TEDDY) categorizes James Lu15, Rainer Machne16, Pedro Mendes10,17, 14 2 10,17 dynamical features of the simulation results and general Matthew Pocock , Nicolas Rodriguez , Alice Villeger , systems behavior. The provision of semantic information 13 2 2 Darren J Wilkinson , Sarala Wimalaratne , Camille Laibe , extends a model’s longevity and facilitates its reuse. It 18 2, Michael Hucka and Nicolas Le Nove`re * provides useful insight into the biology of modeled processes, and may be used to make informed decisions 1 Terry Fox Laboratory, Vancouver, Canada, on subsequent simulation experiments. 2 Department of Computational Neurobiology, EMBL European Bioinformatics Molecular Systems Biology 7: 543; published online 25 October Institute, Wellcome-Trust Genome Campus, Hinxton, UK, 2011; doi:10.1038/msb.2011.77 3 Institute of Computer Science, Friedrich-Schiller University, Jena, Germany, 4 Bioinformatics and Systems Biology, Rostock University, Rostock, Germany, Subject Categories: bioinformatics; simulation and data analysis 5 Center for Bioinformatics Tuebingen, University of Tuebingen, Tu¨bingen, Keywords: dynamics; kinetics; model; ontology; simulation Germany, 6 Department of Biology, Carleton University, Ottawa, Ontario, Canada, 7 Ansys, Abingdon, UK, 8 HITS gGmbH, Heidelberg, Germany, 9 Virginia Bioinformatics Institute, Blacksburg, VA, USA, 10 Manchester Interdisciplinary Biocentre, Manchester, UK, Introduction: semantics in computational 11 School of Chemistry, University of Manchester, Manchester, UK, systems biology 12 Auckland Bioengineering Institute, University of Auckland, Auckland, New Zealand, Models as abstract representations of observed or hypothe- 13 Centre for Integrated Systems Biology of Ageing and Nutrition, Institute for sized phenomena are not new to the life sciences. They have Ageing and Health, Newcastle upon Tyne, UK, 14 long been used as tools for organizing and communicating School of Computing Science, Newcastle University, Newcastle upon Tyne, information. However, the form those models take in systems UK, 15 Biomolecular Signaling and Control Group, Automatic Control Laboratory, biology has changed dramatically. Traditional representations Swiss Federal Institute of Technology Zurich, Zurich, Switzerland, of biomolecular networks have used natural language 16 Theoretical Biochemistry Group, University of Vienna, Vienna, Austria, narratives augmented with block-and-arrow diagrams. While 17 School of Computer Science, University of Manchester, Manchester, UK and useful for describing hypotheses about a system’s components 18 Division of Engineering and Applied Science, California Institute of and their interactions, those representations are increasingly Technology, Pasadena, CA, USA recognized as inadequate vehicles for understanding complex 19 These authors contributed equally to this work systems (Bialek and Botstein, 2004). Instead, formal, quanti- * Corresponding author. Department of Computational Neurobiology, EMBL European Bioinformatics Institute, Wellcome-Trust Genome Campus, Hinxton tative models replace these static diagrams as integrators of CB10 1SD, UK. Tel.: þ 44 (0)1223 494521; Fax: þ 44 (0)1223 494468; knowledge, and serve as the centerpiece of the scientific E-mail: [email protected] modeling and simulation cycle. By systematically describing how biological entities and processes interrelate and unfold, Received 28.3.11; accepted 7.9.11 and by the adoption of standards for how these are defined, represented, manipulated and interpreted, quantitative models can enable ‘meaningful comparison between the consequences of basic assumptions and the empirical facts’ The use of computational modeling to describe and analyze (May, 2004). biological systems is at the heart of systems biology. Model The ease with which modern computational and theoretical structures, simulation descriptions and numerical results tools can be applied to modeling is leading not only to a large can be encoded in structured formats, but there is an increase in the number of computational models in biology, increasing need to provide an additional semantic layer. but also to a dramatic increase in their size and complexity. As Semantic information adds meaning to components of an example, the number of models deposited in BioModels structured descriptions to help identify and interpret them Database (Le Nove`re et al, 2006; Li et al, 2010a) is doubling unambiguously. Ontologies are one of the tools frequently roughly every 22 months while the average number of used for this purpose. We describe here three ontologies relationships between variables per model is doubling every created specifically to address the needs of the systems 13 months. The models published with the first release of biology community. The Systems Biology Ontology (SBO) BioModels Database contained on average 30 relationships per & 2011 EMBO and Macmillan Publishers Limited Molecular Systems Biology 2011 1 Ontologies for modeling and simulation M Courtot et al model, and this number rose to around 100 in the 17th release. examples include the Gene Ontology (Ashburner et al, 2000), Standardization of the encoding formats is required to search, the Foundational Model of Anatomy (Rosse and Mejino, 2003) compare or integrate such a large amount of models. We have and BioPAX (Demir et al, 2010). Ontologies used in conjunc- argued that the standards used in descriptions of knowledge in tion with standard formats provide a rich, flexible, fast- life sciences can be divided into three broad categories: evolving semantic layer on top of the stable and robust content standards, syntax standards and semantic standards standard formats. (see for instance the matrix in Le Nove`re, 2008). Content While existing ontologies adequately cover the biology standards provide checklists or guidelines as to what encoded in models, we extend the idea to model-related information should be stored for a particular data type or information. We describe three ontology efforts to standardize subject area. Examples of such Minimum Information check- the encoding of semantics for models and simulations in lists are hosted on the MIBBI portal (Taylor et al, 2008). Syntax systems biology. These publicly available, free consensus standards provide structures for formatting the information ontologies are the Systems Biology Ontology (SBO), the Kinetic requested in a content standard. Frequent examples are Simulation Algorithm Ontology (KiSAO) and the Terminology representation formats, for instance using an XML language. for the Description of Dynamics (TEDDY). Together, they Semantic standards provide a unified, common definition for provide stable and perennial identifiers, referencing machine- all words, phrases or vocabulary used to describe a particular readable, software-interpretable, regulated terms. These data type or subject area. By using standards from these three ontologies define semantics for the aspects of models, which categories in concert, model descriptions can achieve both correspond to the three steps of the modeling and simulation human and computational usability, reusability and interoper- process as shown in Figure 1. The efforts we introduce here are ability, and it has even been claimed that ‘the markup is the at different stages of development and have different levels of model’ (Kell and Mendes, 2008). community support; SBO is a well-established software tool, Computational models, expressed in representation formats KiSAO gathers increasing community support and TEDDY such as the Systems Biology Markup Language (SBML; Hucka is as yet in its infancy, being primarily a research project. et al, 2003), CellML (Lloyd et al, 2004) and NeuroML (Gleeson The purpose of our work is to provide practical tools for et al, 2010), still require much human interpretation. While computational systems biology and as such, the development syntax standards define the format for expressing the of the ontologies presented here is largely driven by the needs mathematical structure of models (i.e. the variables and their of the projects using them. However, their focus and coverage mathematical relationships), they define neither what the is not voluntarily restricted and any community requirements variables and the mathematical expressions represent, nor will, in general, be accommodated. All three ontologies aim to how they were generated. Where this critical information is fill specific niches in the concept space covered by the Open communicated through free-text descriptions or non-standard Biomedical Ontology (OBO) foundry (Smith et al, 2007). The annotations, it can only—if at all—be
Details
-
File Typepdf
-
Upload Time-
-
Content LanguagesEnglish
-
Upload UserAnonymous/Not logged-in
-
File Pages12 Page
-
File Size-