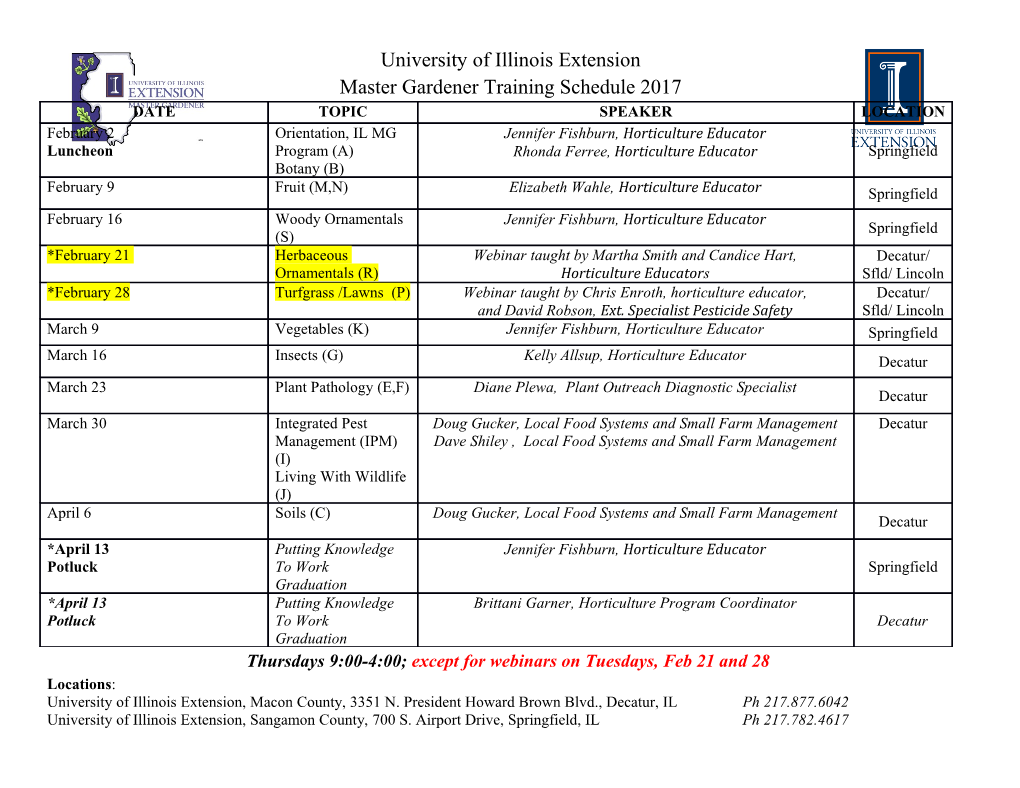
PubH 7470: STATISTICS FOR TRANSLATIONAL & CLINICAL RESEARCH Instructor: Chap T. Le, Ph.D. Distinguished Professor of Biostatistics Basic Issues: COURSE INTRODUCTION BIOSTATISTICS BIOSTATISTICS is the Biomedical Version of the TRIAL BY JURY. It can be defined as “the science of dealing with uncertainties using incomplete information.” Obviously, it is an essential component of Biomedical Research; we have to face uncertainties and, most of the times, we have to rely on incomplete information. AREAS OF BIOSTATISTICS Research using Biostatistics is a three-step process: (1) Sampling/design: Find a way or ways to collect data (going from population to sample). (2) Descriptive statistics: Learn to organize, summarize and present data which can shed light on the research question (investigating sample). (3) Inferential statistics: Generalize what we learn from the sample or samples to the target population and answer the research question (going from sample to population). External Validity Internal Validity Truth in Truth in Findings in The Universe The Study The Study Research Question Study Plan Study Data INFERENCES & VALIDITIES • Two major levels of inferences are involved in interpreting the results/findings of a study: The first level concerns Internal validity; the degree to which the investigator draws the correct conclusions about what actually happened in the study. The second level concerns External Validity (also referred to as generalizability or inference); the degree to which these conclusions could be appropriately applied to people and events outside the study. Validity is an important concept/component in research. It involves the assessment against accepted absolute standards which are often not available; or in a milder form, to see if the evaluation appears to cover its intended target or targets. Statistical contributions involve both Internal Validity and External Validity of any research project. STATISTICAL ISSUES • Statistics is a way of thinking, thinking about ways to gather and analyze data. • The gathering part (i.e. data collection) comes before the analyzing part; the first thing a statistician or a learner of statistics does when faced with a biomedical project is data collection (followed by data management and data analysis). • Studies may be inconclusive because they were poorly planned or not enough data were collected to accomplished the goals and support the hypotheses. THE IMPORTANT PHASE Just as in the case of “Trial by Jury”, the most important stage of the “Research Process” is the DESIGN: How & How Much data are collected! Also, It dictates how data should be analyzed. May be it’s not the question of “how” to collect your data but the decision on “when to do what”! Most training programs in Biostatistics offer a course in “Clinical Trials”; some may have a more advanced course, “Advanced Clinical Trials”. But not all studies are clinical trials; there are studies of other forms. Clinical Research Population Research T1 T2 Laboratory Research Studies can be grouped into there areas: Population, Laboratory, and Clinical; plus Translational Research, the component of basic science that interacts with clinical (T1) or with population research (T2). In addition, what you would learn in a typical clinical trial course are all about “randomized, controlled phase III clinical trials”. It’s about “serious, large-scale efforts” in later stage of clinical research. How often, or how likely, you’re going to see these trials in your practice as a consulting statistician or practitioner? As in the case of courses in Biostatistics, we do cover some topics in Data Analysis – especially topics not covered in other biostatistics courses but we also put equal – even more - emphasis on “Study Design” which I consider the “more important phase” of research and of Biostatistics. A BASIC ISSUE IN RESEARCH Most of the times, inexperienced researchers mistakenly act like there is an identifiable, existent parent population or populations of subjects. We act as if the sample or samples is/are obtained from the parent population or populations according to a carefully defined technical procedure called “random sampling”. This is not true in real-life biomedical studies. The laboratory investigator uses animals in his projects but the animals are not randomly selected from any large population of animals. The clinician, who is attempting to describe the results he has obtained with a particular therapy, cannot say that his patients is a random sample from a parent population of patients. ONE-SAMPLE CASE A surgeon might attempt to convince readers that the results on his 25 patients typify the results expected from his procedure. On the one hand, he carefully explain/describe his report as “pure description”. On the other hand, he goes to some lengths to assure that that these patients are like a sample – “unselected” . He makes an inference from sample mean to population mean; calculating the standard error which can help in assessing the reliability of the sample mean for this purpose. Then a 95% confidence interval is provided to complete the report. Many “one-sample studies” are still being conducted because there are no better other choices. A typical case are “Phase II Clinical Trials” for cancers. A group of patients take the same dose of an experimental drug; the result is a “response rate” (the proportion of patients respond to the new drug: size of tumor reduced in half lasting four weeks or longer). However, the broad inference to patients operated by other surgeons, in other years or other institutions is still … very dangerous, And the standard error of the mean cannot be trusted to measure all of these uncertainties because “random sampling” has not been done. So, what can investigators like this surgeon do in one-sample cases? First, the surgeon can describe his group of patients in some detail so that his readers can see the nature of the patients he operated, their age range, the severity of their disease, and so forth; the logic is the more similar patients the results are more likely similar. Second, in measuring the effects of treatment or operation, he can report measurements before and after surgery, so that each patient serves as his/her own control, so to speak. The focus on the mean/proportion and its standard error might be misleading; single sample studies remain difficult to evaluate, with or without statistics. MULTIPLE-SAMPLE CASE We have more than one group; in many clinical studies and in most laboratory studies a control group is included, so that comparisons can be made between “experimental effect” and “placebo effect” within the study itself. These are comparative experiments THE VALUE OF TRIALS • Because they are not population-based (there is not an identifiable, existent parent population of subjects for sample selection), biomedical studies – designed experiments are “comparative”. That is the validity of the conclusions is based on a comparison. • In a clinical trial, we compare the results from the “treatment group” versus the results from the “placebo group”. The validity of the comparison is backed by the “randomization”, a method proposed by Fisher in 1935 Randomization serves two purposes. First, the groups of study units or arms receiving the different treatments tend to be comparable on all variables, known and unknown. Second, such randomization provides a secure foundation on which statistical measures (standard error, p- value) can be justified. Biomedical studies are often conducted to “demonstrate” or confirm or establish a relationship between an exposure or explanatory factor and an outcome or response variable. The demonstration is accomplished by comparing the outcomes or responses from different levels of the explanatory factor or exposure. Different ways to show case the relationship form different “designs”. EXPERIMENTAL DESIGNS There are three different Designs (methods for data collection) depending on the timing (present, past, and future) and the focus (disease or exposure): • Cross-sectional, e.g. surveys • Case-Control (retrospective) • Cohort (prospective); clinical trials are of an important special form. Cross-sectional designs are one-sample studies but the focus is on the relationship which is less likely changed because of the lack of randomness than the group mean or proportion. Nevertheless, their validity still remain not as firmed. Case-Control Design Factor Factor Present Absent Disease Sample 1: Cases No Disease Sample 2: Controls Retrospective Studies gather past data from selected cases (with disease) and controls (without disease) to determine differences, if any, in exposure to a suspected risk factor. Advantages: Economical & Quick. Major Limitations: Accuracy of exposure histories & Appropriateness of controls The Cross-sectional and Case-control designs are often referred to as “observational”. Some call the first category exploratory observational studies and the second confirmatory observational studies. As opposed to “observational” studies, the others are “experimental”. A CLINICAL TRIAL Study Initiation Study Termination No subjects enrolled after 1 0 1 2 Enrollment Period, Follow-up Period, e.g. three (3) years e.g. two (2) years OPERATION: Patients come sequentially; each is enrolled and randomized to receive one of two or several treatments, and followed for varying amount of time- between 1 & 2 Some investigators further divides the experimental group into controlled experiments and controlled experiments with covariates.
Details
-
File Typepdf
-
Upload Time-
-
Content LanguagesEnglish
-
Upload UserAnonymous/Not logged-in
-
File Pages40 Page
-
File Size-