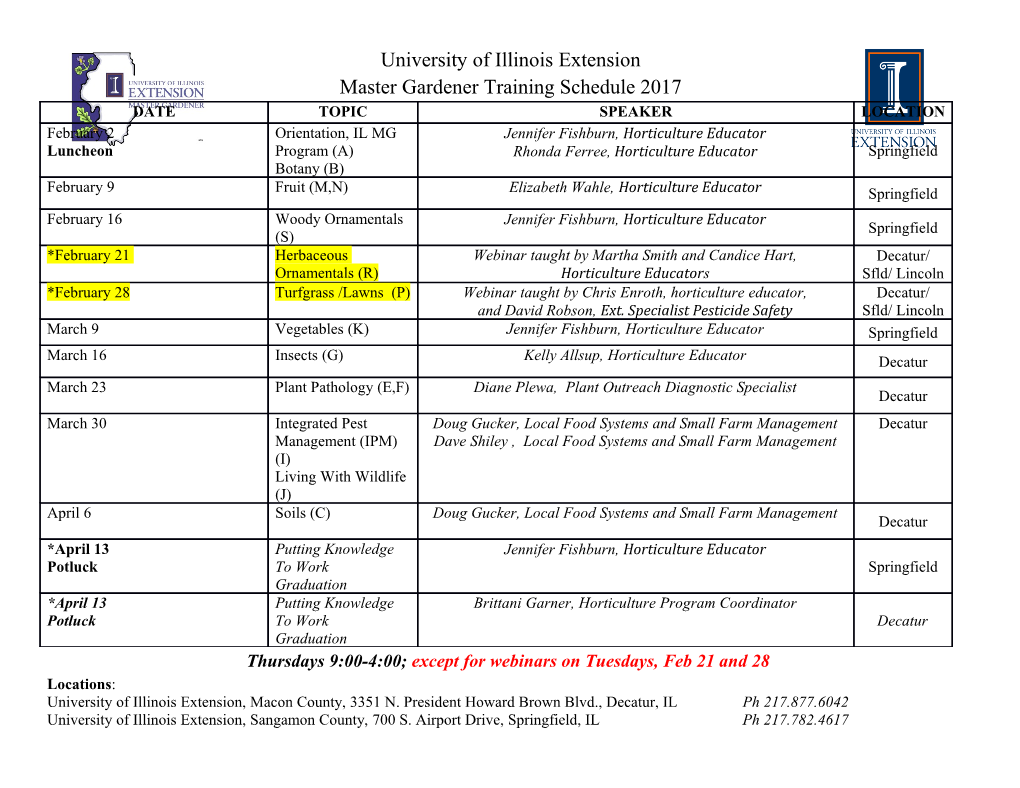
PREDICTABILITY AND CHAOS 1777 Crowley G (1991) Dynamics of the Earth’s thermosphere. Rishbeth H (2001) The centenary of solar-terrestrial physics. US National Report to International Union of Geodosy Journal of Atmospheric and Solar–Terrestrial Physics 63: and Geophysics 1987–1990, Review of Geophysics, 1883–1890. Supplement, pp. 1143–1165. Rishbeth H and Garriott OK (1969) Introduction to Hedin AE and Carignan GR (1985) Morphology of thermo- Ionospheric Physics. London: Academic Press. spheric composition variations in the quiet polar meso- Roble RG (1986) Chemistry in the thermosphere and sphere from Dynamics Explorer measurements. Journal ionosphere. Chemical Engineering News. 64(24): 23–38. of Geophysical Research 90: 5269–5277. Roble RG (1992) The polar lower thermosphere. Planetary Killeen TL (1987) Energetics of the Earth’s thermosphere. Space Science 40: 271–297. Reviews of Geophysics 25: 433–454. Rocket P (1952) Pressure, densities and temperatures Killeen TL and Roble RG (1988) Thermospheric dynamics: in the upper atmosphere. Physics Review 88: contributions from the first 5 years of the Dynamics 1027–1032. Explorer program. Reviews of Geophysics 26: 329–367. Schunk RW and Nagy AF (2000) Ionospheres, Physics, Rees MH (1989) Physics and Chemistry of the Upper Plasma Physics and Chemistry. Cambridge: Cambridge Atmosphere. Cambridge: Cambridge University Press. University Press. PREDICTABILITY AND CHAOS L A Smith, London School of Economics, London, UK will limit our ability to make probability (PDF) Copyright 2003 Elsevier Science Ltd. All Rights Reserved. forecasts, just as uncertainty in the initial condition limits the utility of single forecasts even if the model is perfect. Introduction Initially, it appears that chaotic systems will be unpredictable, and this is true in that it is not possible Chaos is difficult to quantify. The nonlinear dynamic to make extremely accurate forecasts in the very that gives rise to chaos links forecasting on the shortest distant future. Yet chaos per se does not imply one time scales of interest with behavior over the longest cannot sometimes make accurate forecasts well into time scales. In addition, the statistics which quantify the medium range. And perhaps just as importantly, chaos forbid an appeal to most of the traditional with a perfect model one can determine which of these simplifications employed in statistical estimation. forecasts will be informative and which will not at the Nevertheless, both the concept of chaos in physical time they are made. As we shall see below, both the systems and its technical relative in mathematics have American and the European weather forecast centers had a significant impact on meteorological aims and have adopted this strategy operationally, with the aim methods. This is particularly true in forecasting. of quantifying day-to-day variations in the likely range Chaos implies sensitivity to initial conditions: small of future meteorological variables. Quantifying this uncertainties in the current state of the system will range can be of significant value even without a perfect grow exponentially-on-average. Yet, as discussed model. Although accurate probability forecasts are below, neither this exponential-on-average growth likely to require a perfect model, current operational nor the Lyapunov exponents that quantify it reflect ensemble prediction systems already provide econom- macroscopic predictability. The limits chaos places on ically valuable information on the uncertainty of predictability are much less severe than generally numerical weather prediction (NWP) well into ‘week imagined. Predictability is more clearly quantified two’, and research programs on seasonal time scales through traditional statistics, like uncertainty dou- are underway. bling times. These statistics will vary from day to day, Why is perfect foresight of the future state of the depending on the current state of the atmosphere. atmosphere impossible? First, it should be no surprise Maintaining the uncertainty in the initial state within that if our knowledge of the present is uncertain then the forecast is a central goal of ensemble forecasting our knowledge of the future will also be uncertain; the (see Weather Prediction: Ensemble Prediction). question of prediction then turns to how to best Achieving the ultimate goal of meaningful probability quantify the dynamics of uncertainty. Here the full forecasts for meteorological variables would be of implications of chaos, or more properly of nonlinear- great societal and economic value. Fundamental ity, mix what are often operationally distinct tasks: limitations in the realism of models of the atmosphere observing strategy, data assimilation, state estimation, 1778 PREDICTABILITY AND CHAOS ensemble formation, forecast evaluation, and model realization of the Lorenz model is chaotic, and even improvement. This complicates the forecasting prob- more so modern NWP models. lem beyond the limits in which classical prediction theory was developed. First the traditional goal of a single best first guess (BFG) forecast with a minimal Chaos least-squares error is not a viable aim in this scenario, Mathematically, a chaotic system is a deterministic nor is a least squares solution desired. Indeed (as system in which (infinitesimally) small uncertainties in discussed below) this approach would reject the the initial condition will grow, on average, exponenti- perfect model which generated the observations! ally fast. The average is taken over (infinitely) long Second, current models are not perfect. The term periods of time. Of course, finite uncertainties often ‘model inadequacy’ is used to summarize imperfec- grow rapidly as well, in which case any uncertainty in tions in a given model and the entire model class from the initial condition will limit predictability in terms of which that model is a member. Model improvement a single BFG of the future state, even with a perfect and the search for a superior model class, along with model. But inasmuch as it is defined by the behaviour the investigation of more relevant measures of model of (infinitesimally) small uncertainties of (infinitely) skill, are areas of active research. long periods of time, chaos per se places no limits of the growth of finite uncertainty over a finite period of time. Chaotic systems are often said to display sensitive dependence on initial condition (SDIC), a A Mathematical Framework for technical term for systems in which states initially very Modelling Dynamical Systems close together tend to end up very far apart, eventually. Suppose the true state of the system is x~: what is the Chaos is a phenomenon found in many nonlinear behaviour of a near-by solution x^ where x^ ¼ x~ þ e?In mathematical models. While one should never forget the pendulum, a small initial e grows slowly, if at all. In the distinction between the model and the system a chaotic system, the magnitude of e will grow being modeled, precise mathematical definitions are exponentially-on-average; yet this does not imply more easily made within the perfect model scenario that the actual magnitude of e ever grows exponenti- (PMS). Within this useful fiction, one assumes that the ally in time. Indeed, since e is a distance and jej >0 for model in-hand is itself the physical system of interest. any given value of t, one can always define a value Before moving back to forecasts of the real world, of course, one must recover from this self-deception. 1 l ¼ log ½jeðtÞj=jeð0Þj Given a model, an initial condition is simply an t assignment of values to all model variables at a particular starting time. Thus the initial condition for any system, chaotic or not! In this case, observing a reflects the current state of the model: it is a vector xðtÞ value of l >0 for finite t does not even suggest which specifies the value of every variable in the model exponential growth. Statistics like l become interest- at time t. For the classical model of a pendulum, the ing only when they approach a constant as t !1;by state consists of two variables (the angle and the definition, chaos reflects properties only in this limit. angular velocity). These two numbers completely Clearly, chaos includes special cases where magni- define the current state of the model, and so the model tude of e is growing uniformly, say doubling every is called two-dimensional, or, equivalently, said to second; but it also allows the more common case have a two-dimensional state space. The famous where the growth of e is a function of the state x and Lorenz model of 1963 is three-dimensional, as there hence changes with time. In general, the growth will are three variables: x ¼ðx; y; zÞ. These low-dimen- not be uniform in time. In fact in some chaotic systems, sional systems should be contrasted with the state of an including the Lorenz 1963 model, there are regions of operational NWP model, which may consist of over 10 the state space in which every e will decrease! Such million variables. regions are said to represent ‘return of skill’ as The sequence of states a dynamical system passes forecasts become more accurate in the least-squares through defines the history of the system; this sequence sense as time passes. is called a trajectory. For deterministic systems, any For instance, consider the case where, on average, single state x along a trajectory defines all future states half the time e is constant and the other half of the time of the system. For the classical pendulum, these it grows by a factor of four. This will yield in the same solutions can be written down analytically; but for exponential-on-average growth as doubling every all but the simplest nonlinear systems only numerical time step, yet there will be times when prediction is solutions exist. Thus it is difficult to prove that a given easy. Or for a more extreme case, consider where e PREDICTABILITY AND CHAOS 1779 shrinks by a factor of two 9 times out of 10, but once in geometry.
Details
-
File Typepdf
-
Upload Time-
-
Content LanguagesEnglish
-
Upload UserAnonymous/Not logged-in
-
File Pages9 Page
-
File Size-