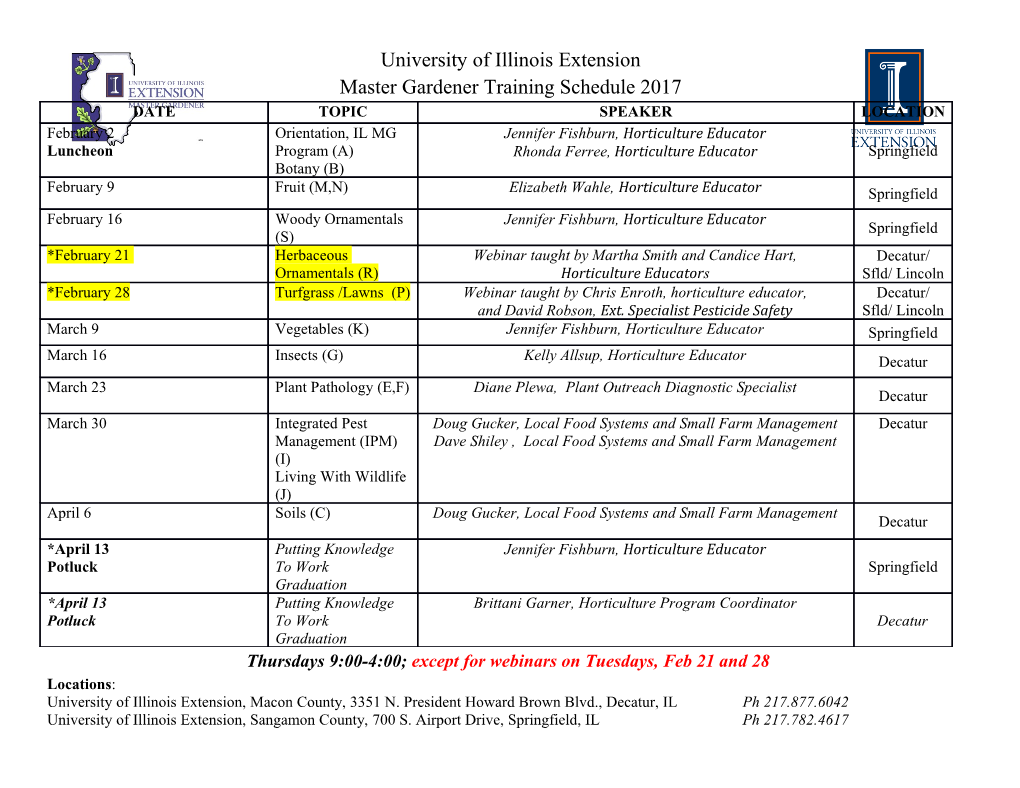
Introduction to Biosystematics - Zool 575 Introduction to Biosystematics Final Review Lecture 37 - Final Review Outline 1. Four steps 2. Hennigian Terminology 3. Saturation, correction, models of evolution, ASRV 4. Maximum Likelihood 5. Computation time is too long Derek S. Sikes University of Calgary Zool 575 Four steps - each should be explained in methods Polarity Hennigian terminology 1. Character (data) selection (not too fast, not too slow) “Why did you choose these data?” nouns apomorph - derived character state 2. Alignment of Data (hypotheses of primary autapomorph - derived character state not shared homology) “How did you align your data?” synapomorph - shared derived character state plesiomorph - ancestral character state 3. Analysis selection (choose the best model / synplesiomorph - shared ancestral character method(s)) - data exploration “Why did you state chose your analysis method?” adjectives apomorphic, autapomorphic, etc. 4. Conduct analysis Lack of a reproducible method resulted in three major approaches: (Explosion in 1960s) 1. Phenetics - similarity / distances only, not evolution, not phylogeny 2. Cladistics - phylogeny inferred using characters & parsimony 3. Statistical Phylogenetics - phylogeny inferred using corrected data & “best fitting model” [both distance & character data] 1 Introduction to Biosystematics - Zool 575 Saturation Models of Sequence Evolution For distance data: Saturation graph as time proceeds DNA distances also - distances are the branch lengths increase, to a point of saturation In extreme cases - correction to observed (D) to yield estimate of the data become actual (d) DNA essentially Distances randomized & all - simplest correction: Jukes and Cantor 1969 phylogenetically actual valuable (JC69) observed information is gone d = -3/4ln (1- 4/3 D) time eg D = 0.119, d = 0.130 eg D = 0.00482, d = 0.00483 Models of Sequence Evolution Maximum Likelihood - variable branch length Some features many models deal with: As the branch length increases the probability of a base changing increases (and the probability of it staying the 1. The tendency of one state to change to same decreases - of course, they can’t both increase!) another (rate) For a branch 3 times longer than our initial branch: 2. The frequency of the different states A C G T (base composition) P3 = A 0.93 0.029 0.019 0.022 C 0.007 0.949 0.015 0.029 3. Variation in rates of change among G 0.01 0.029 0.939 0.022 characters (ASRV) T 0.007 0.038 0.015 0.94 - some sites change a lot, others change little or not at all Models of Sequence Evolution The shape of the gamma distribution for Among Site Rate Variation (ASRV) different values of alpha. - if present in data and not accounted for If alpha = infinity, analysis can be mislead - inconsistent, rate is equal for all erroneous sites (no ASRV) - can be accommodated by adding extra parameters to any model - pInvar - proportion of invariable sites [ eg JC+I] - gamma - distribution of ASRV controlled by one parameter, alpha Low alpha (eg 0.5 and lower) = high ASRV [ eg GTR+I+G] High alpha (eg > 2) = low ASRV 2 Introduction to Biosystematics - Zool 575 Models of Sequence Evolution Correction doesn’t always work… - Huge dataset of 124,026 characters More complex models usually fit the data better - Data corrected with GTR and LogDet - 100% branch support - STILL WRONG! Optimality Criteria - Given 2+ trees Parsimony & Maximum Likelihood Parsimony & Cladistics - Strict cladists typically use only parsimony methods Maximum Parsimony & justify this choice on philosophical grounds The tree hypothesizing the fewest eg it provides the “least falsified hypothesis” number of character state changes is - Parsimony has also been interpreted as a fast the best approximation to maximum likelihood Cavalli-Sforza and Edwards (1967:555), stated that parsimony’s “… Maximum Likelihood success is probably due to the closeness of the solution it gives to the projection of the ‘maximum likelihood’ tree” and parsimony “certainly The tree maximizing the probability cannot be justified on the grounds that evolution proceeds according to of the observed data is best some minimum principle…”. Maximum Likelihood (ML) Maximum Likelihood (ML) Slow incorporation into systematics 1. Computationally complex - limited early uses to A statistical model-fitting exercise small molecular datasets (<12 OTUs) 2. Molecular only (until 2001) Which model (process + tree [topology+branch lengths]) 3. Slow development of good software is most likely to have generated the 4. Slow development of objective means to choose process models observed data? Now we have more sophisticated software, faster Maximizing the probability of the data given a computers, more realistic models, and means to model L=Pr(D|H) choose among models ML is becoming a widely used method Select process model using AIC etc. select Joseph Felsenstein and David Swofford instrumental in developing tree & model parameters using ML software to use ML 3 Introduction to Biosystematics - Zool 575 Maximum Likelihood (ML) Maximum Likelihood (ML) Goal: To estimate the probability that we We then can rank & choose among alternate would observe a particular dataset, given a trees by selecting the tree which is most phylogenetic tree and some notion of how likely to have given rise to our data the evolutionary process worked over time = an optimality criterion Probability of given ( ) Probability of given ( ) Maximum Likelihood Maximum Likelihood ML says nothing about the likelihood of Requires explicit process model of evolution the hypothesis being true it simply allows Strength: assumptions are spelled out unlike us to select among many hypotheses Parsimony - which also requires a model but the based on which makes the data most model is implicit & only recently understood likely statistically eg. Loud sounds coming from your attic (data) - The hypotheses (the model) includes: Hypothesis 1: gremlins are bowling upstairs 1. The process model (eg JC69, GTR+G) Hypothesis 2: gremlins are sleeping upstairs 2. The topology (tree) 3. The branch lengths of (2n-3) branches for n hypothesis 1 makes the data more likely than taxa hypothesis 2 - but itself is highly improbable! Maximum Likelihood - branch lengths ML comparison with Parsimony (MP) This is key… (1) ML estimates “corrected” branch lengths (corrected for unobserved changes) 1. The most parsimonious topology is often, but not And (2) uses these branch lengths to adjust the probabilities always, the same as the maximum likelihood of change topology Branch lengths are estimated in units of expected number of 2. Parsimony does not correct the data for unobserved changes per character changes and so parsimony branch lengths are typically underestimates of actual branch The probability of change is a product of mutation rate (µ) lengths and time (t) - Parsimony branch lengths = the estimated number of changes that occurred & are mapped onto Branches can be long due to large t, or higher µ, or both the tree after it has been found (not used during (hard to tease apart t from µ) if all branches have same tree searching - only total tree length is used to µ then the data fit a molecular clock (ultrametric) select the best tree) 4 Introduction to Biosystematics - Zool 575 ML comparison with Parsimony (MP) ML comparison with Parsimony (MP) 3. Because Parsimony ignores branch length 4. Parsimony minimizes homoplasy information during searches it is - ML will sometimes prefer a tree with more than the - much faster than ML minimum amount of homoplasy (if it makes the data - unable to use this information to help it find the more likely given the model) most probable tree (some strict cladists argue that they do not use parsimony to find the most - Again, it is branch lengths which indicate where probable tree, they claim there is no connection homoplasy may be common in the tree - longer between minimal tree length and probability of branches are more likely to be involved in homoplastic being correct, “truth is unknowable”) events than shorter ones - especially unable to use branch lengths (~ rates of change) to detect areas of the tree that are [Note: Parsimony is sometimes called Maximum Parsimony likely to experience higher rates of homoplasy and abbreviated MP in contrast to ML] than other regions ML comparison with Parsimony (MP) ML comparison with Parsimony (MP) Parsimony would never prefer the correct, but longer Thus we expect MP to yield the same tree as ML when tree on the left whereas ML would (more on this in next branch lengths are similar across the tree (when lecture) - Parsimony also ignores autapomorphies there is little branch-length heterogeneity) Contrary to some statements to the opposite, it doesn’t autapomorphies matter how much change has occurred (some have said that MP will only work if there has A B A B been little change - low rates of evolution) 3 This is wrong, MP can do fine with high rates of 1 1 1 2 change as long as there is little branch length 2 2 ML 3 MP heterogeneity - and conversely, even with TINY 3 amounts of change MP can fail if branches are C D C D of significantly different lengths 2 convergence events 1 convergence event Desirable Attributes of a method Desirable Attributes of a method 1. Consistency 1. Consistency 2. Efficiency 3. Robustness - a method is consistent if it converges on the correct tree as the data set becomes infinitely 4. Computational Speed large (ie there is no systematic error) 5. Discriminating ability 6. Versatility - all methods are consistent if their assumptions are met (ie their model is not violated) - Some can be assessed using simulations - Data are simulated and later analyzed - conversely, model misspecification can cause using a method to determine its attributes any method to be inconsistent 5 Introduction to Biosystematics - Zool 575 Desirable Attributes of a method Desirable Attributes of a method 1. Consistency 2. Efficiency (cont.) - inconsistency manifests as the preference for - some methods are consistent but not very the wrong tree as the data become infinitely efficient (require TONS of data to work) numerous - MP, when its assumptions are not violated, is far more efficient than ML 2.
Details
-
File Typepdf
-
Upload Time-
-
Content LanguagesEnglish
-
Upload UserAnonymous/Not logged-in
-
File Pages9 Page
-
File Size-