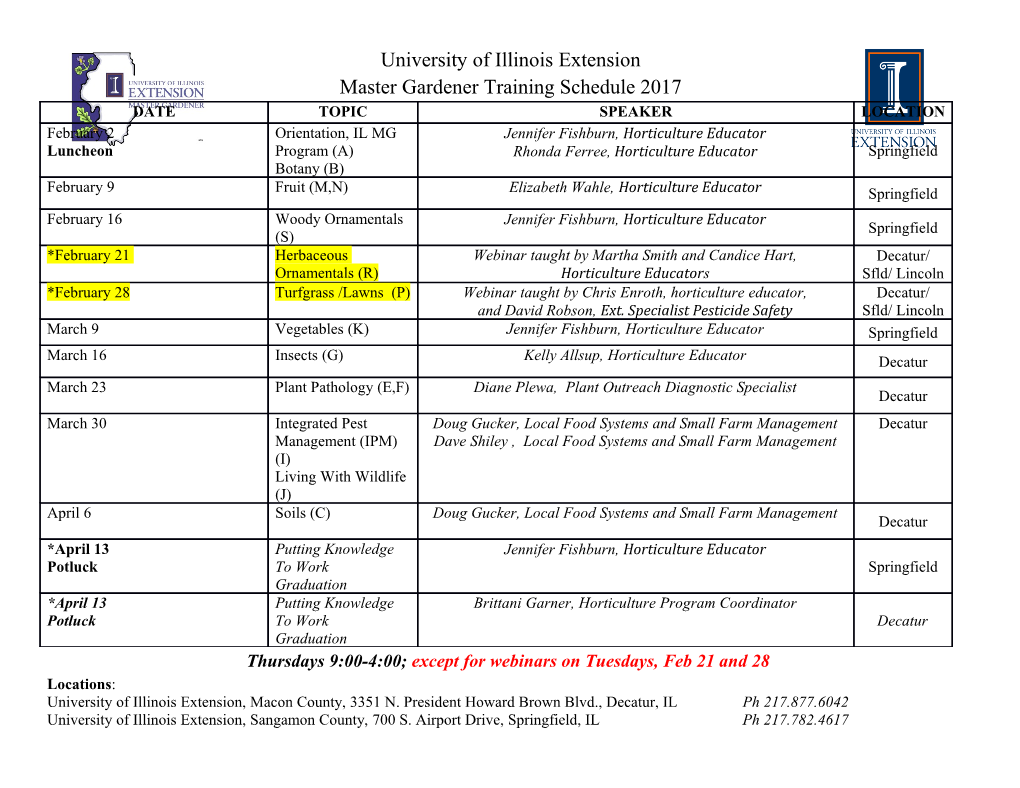
6.3 Orthogonal Projections Math 2331 { Linear Algebra 6.3 Orthogonal Projections Jiwen He Department of Mathematics, University of Houston [email protected] math.uh.edu/∼jiwenhe/math2331 Jiwen He, University of Houston Math 2331, Linear Algebra 1 / 16 6.3 Orthogonal Projections Orthogonal Projection Decomposition Best Approximation 6.3 Orthogonal Projections Orthogonal Projection: Review Orthogonal Projection: Examples The Orthogonal Decomposition Theorem The Orthogonal Decomposition: Example Geometric Interpretation of Orthogonal Projections The Best Approximation Theorem The Best Approximation Theorem: Example New View of Matrix Multiplication Orthogonal Projection: Theorem Jiwen He, University of Houston Math 2331, Linear Algebra 2 / 16 6.3 Orthogonal Projections Orthogonal Projection Decomposition Best Approximation Orthogonal Projection: Review y·u by = u·u u is the orthogonal projection of onto . n Suppose fu1;:::; upg is an orthogonal basis for W in R . For each y in W , y · u1 y · up y = u1 + ··· + up u1 · u1 up · up Jiwen He, University of Houston Math 2331, Linear Algebra 3 / 16 6.3 Orthogonal Projections Orthogonal Projection Decomposition Best Approximation Orthogonal Projection: Example Example 3 Suppose fu1; u2; u3g is an orthogonal basis for R and let 3 W =Spanfu1; u2g. Write y in R as the sum of a vector by in W and a vector z in W ?. Jiwen He, University of Houston Math 2331, Linear Algebra 4 / 16 6.3 Orthogonal Projections Orthogonal Projection Decomposition Best Approximation Orthogonal Projection: Example (cont.) Solution: Write y · u1 y · u2 y · u3 y = u + u2 + u3 u1 · u1 1 u2 · u2 u3 · u3 where y · u1 y · u2 y · u3 by= u + u2; z = u : u1 · u1 1 u2 · u2 u3 · u3 3 To show that z is orthogonal to every vector in W , show that z is orthogonal to the vectors in fu1; u2g : Since z · u1 = = = 0 z · u2 = = = 0 Jiwen He, University of Houston Math 2331, Linear Algebra 5 / 16 6.3 Orthogonal Projections Orthogonal Projection Decomposition Best Approximation The Orthogonal Decomposition Theorem Theorem (8) Let W be a subspace of Rn. Then each y in Rn can be uniquely represented in the form y =by + z ? where by is in W and z is in W . In fact, if fu1;:::; upg is any orthogonal basis of W , then y · u1 y · up by = u1 + ··· + up u1 · u1 up · up and z = y−by: The vector by is called the orthogonal projection of y onto W : Jiwen He, University of Houston Math 2331, Linear Algebra 6 / 16 6.3 Orthogonal Projections Orthogonal Projection Decomposition Best Approximation The Orthogonal Decomposition Theorem Jiwen He, University of Houston Math 2331, Linear Algebra 7 / 16 6.3 Orthogonal Projections Orthogonal Projection Decomposition Best Approximation The Orthogonal Decomposition: Example Example 2 3 3 2 0 3 2 0 3 Let u1 = 4 0 5, u2 = 4 1 5 ; and y = 4 3 5. Observe that 1 0 10 fu1; u2g is an orthogonal basis for W =Spanfu1; u2g. Write y as the sum of a vector in W and a vector orthogonal to W . Solution: proj y = y = y·u1 u + y·u2 u W b u1·u1 1 u2·u2 2 2 3 3 2 0 3 2 3 3 = ( ) 4 0 5 + ( ) 4 1 5 = 4 3 5 1 0 1 2 0 3 2 3 3 2 −3 3 z = y−by = 4 3 5 − 4 3 5 = 4 0 5 10 1 9 Jiwen He, University of Houston Math 2331, Linear Algebra 8 / 16 6.3 Orthogonal Projections Orthogonal Projection Decomposition Best Approximation Geometric Interpretation of Orthogonal Projections Jiwen He, University of Houston Math 2331, Linear Algebra 9 / 16 6.3 Orthogonal Projections Orthogonal Projection Decomposition Best Approximation The Best Approximation Theorem Theorem (9 The Best Approximation Theorem) n n Let W be a subspace of R , y any vector in R , and by the orthogonal projection of y onto W . Then by is the point in W closest to y, in the sense that ky−byk < ky − vk for all v in W distinct from by. Jiwen He, University of Houston Math 2331, Linear Algebra 10 / 16 6.3 Orthogonal Projections Orthogonal Projection Decomposition Best Approximation The Best Approximation Theorem (cont.) Outline of Proof: Let v in W distinct from by. Then v−by is also in W (why?) z = y−by is orthogonal to W ) y−by is orthogonal to v−by 2 2 2 y − v = (y−by) + (by−v) =) ky − vk = ky−byk + kby−vk . 2 2 ky − vk > ky−byk Hence, ky−byk < ky − vk. Jiwen He, University of Houston Math 2331, Linear Algebra 11 / 16 6.3 Orthogonal Projections Orthogonal Projection Decomposition Best Approximation The Best Approximation Theorem: Example Example Find the closest point to y in Spanfu1; u2g where 2 2 3 2 1 3 2 0 3 6 4 7 6 1 7 6 0 7 y = 6 7, u1= 6 7, and u2= 6 7. 4 0 5 4 0 5 4 1 5 −2 0 1 Solution: y · u1 y · u2 by= u1 + u2 u1 · u1 u2 · u2 2 1 3 2 0 3 6 1 7 6 0 7 = ( ) 6 7 + ( ) 6 7 = 4 0 5 4 1 5 0 1 Jiwen He, University of Houston Math 2331, Linear Algebra 12 / 16 6.3 Orthogonal Projections Orthogonal Projection Decomposition Best Approximation New View of Matrix Multiplication Part of Theorem 10 below is based upon another way to view matrix multiplication where A is m × p and B is p × n 2 3 row1B 6 row2B 7 AB = col A col A ··· col A 6 7 1 2 p 6 . 7 4 . 5 rowpB = (col1A) (row1B) + ··· + (colpA) (rowpB) Jiwen He, University of Houston Math 2331, Linear Algebra 13 / 16 6.3 Orthogonal Projections Orthogonal Projection Decomposition Best Approximation New View of Matrix Multiplication: Example Example 5 6 2 1 3 34 5 3 = 3 1 4 0 −2 10 3 7 5 6 2 1 3 3 1 4 0 −2 5 6 = 2 1 3 + 4 0 −2 3 1 = Jiwen He, University of Houston Math 2331, Linear Algebra 14 / 16 6.3 Orthogonal Projections Orthogonal Projection Decomposition Best Approximation Orthogonal Matrix 2 T 3 u1 6 uT 7 T 6 2 7 So if U = u1 u2 ··· up . Then U = 6 . 7. So 4 . 5 T up T T T T UU = u1u1 + u2u2 + ··· + upup T T T T UU y = u1u1 + u2u2 + ··· + upup y T T T = u1u1 y + u2u2 y + ··· + upup y T T T = u1 u1 y + u2 u2 y + ··· + up up y = (y · u1) u1 + (y · u2) u2 + ··· + (y · up) up T ) UU y = (y · u1) u1 + (y · u2) u2 + ··· + (y · up) up Jiwen He, University of Houston Math 2331, Linear Algebra 15 / 16 6.3 Orthogonal Projections Orthogonal Projection Decomposition Best Approximation Orthogonal Projection: Theorem Theorem (10) If fu1;:::; upg is an orthonormal basis for a subspace W of Rn, then projW y = (y · u1) u1 + ··· + y · up up If U = u1 u2 ··· up , then T n projW y =UU y for all y in R . Outline of Proof: y·u proj y = y·u1 u + ··· + p u W u1·u1 1 up·up p T = (y · u1) u1 + ··· + y · up up = UU y. Jiwen He, University of Houston Math 2331, Linear Algebra 16 / 16.
Details
-
File Typepdf
-
Upload Time-
-
Content LanguagesEnglish
-
Upload UserAnonymous/Not logged-in
-
File Pages16 Page
-
File Size-