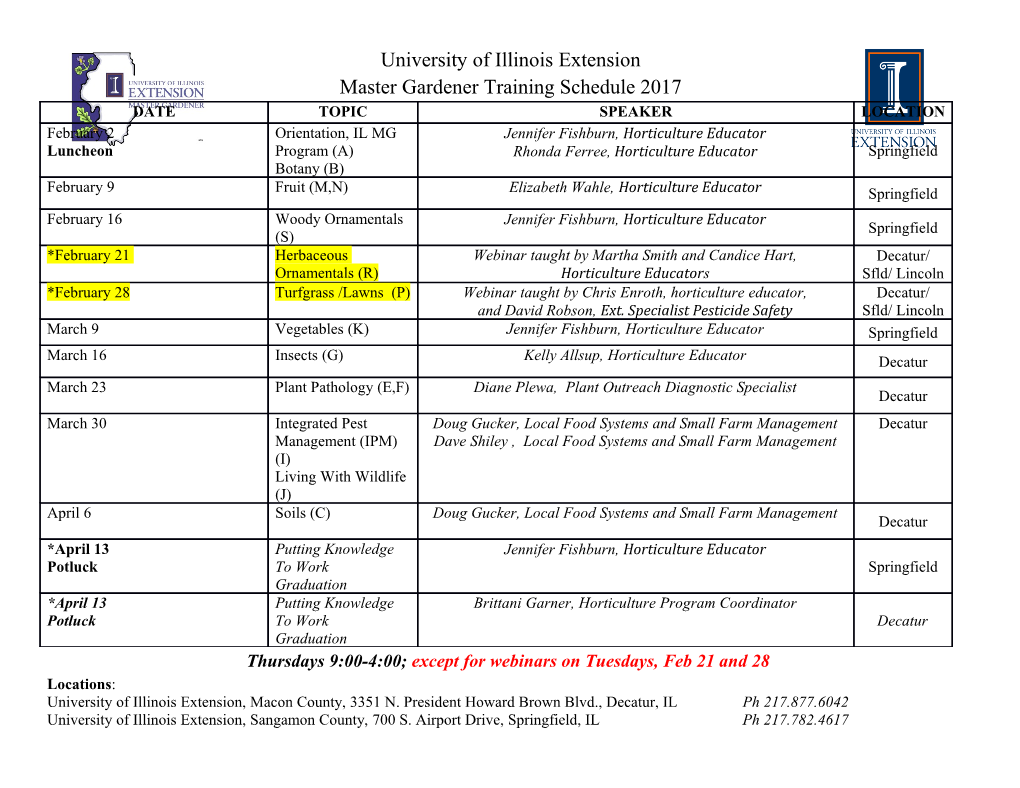
A&A 517, A57 (2010) Astronomy DOI: 10.1051/0004-6361/201014013 & c ESO 2010 Astrophysics Stellar atmosphere parameters with MAχ, a MAssive compression of χ2 for spectral fitting P. Jofré1,B.Panter2, C. J. Hansen3,andA.Weiss1 1 Max-Planck-Institut für Astrophysik, Karl-Schwarzschild-Str. 1, 85741 Garching, Germany e-mail: [email protected] 2 Institute for Astronomy, University of Edinburgh, Royal Observatory, Blackford Hill, Edinburgh EH9 3HJ, UK 3 European South Observatory (ESO), Karl-Schwarzschild-Str. 2, 85748 Garching, Germany Received 7 January 2010 / Accepted 19 April 2010 ABSTRACT MAχ is a new tool to estimate parameters from stellar spectra. It is based on the maximum likelihood method, with the likelihood compressed in a way that the information stored in the spectral fluxes is conserved. The compressed data are given by the size of the number of parameters, rather than by the number of flux points. The optimum speed-up reached by the compression is the ratio of the data set to the number of parameters. The method has been tested on a sample of low-resolution spectra from the Sloan Extension for Galactic Understanding and Exploration (SEGUE) survey for the estimate of metallicity, effective temperature and surface gravity, with accuracies of 0.24 dex, 130 K and 0.5 dex, respectively. Our stellar parameters and those recovered by the SEGUE Stellar Parameter Pipeline agree reasonably well. A small sample of high-resolution VLT-UVES spectra is also used to test the method and the results were compared to a more classical approach. The speed and multi-resolution capability of MAχ combined with its performance compared with other methods indicates that it will be a useful tool for the analysis of upcoming spectral surveys. Key words. techniques: spectroscopic – surveys – stars: fundamental parameters – methods: data analysis – methods: statistical 1. Introduction New surveys such as RAVE (Steinmetz et al. 2006)andGaia (Perryman et al. 2001) will give extremely high accuracies in The astronomical community has conducted many massive sur- velocities and positions, LAMOST (Zhao et al. 2006) and part veys of the Universe, and many more are either ongoing or of the SDSS-III project, APOGEE and SEGUE-2 (Rockosi et al. planned. Recently completed is the Sloan Digital Sky Survey 2009), will give high-quality spectra and therefore stellar param- (SDSS, York et al. 2000), notable for assembling in a consis- eters and chemical abundances of millions of stars over all the tent manner the positions, photometry and spectra of millions of sky. These more accurate properties and larger samples will in- galaxies, quasars and stars, and covering a significant fraction crease our knowledge of the Milky Way and answer wider ques- of the sky. The size of such a survey allows us to answer many tions about the formation and evolution of spiral galaxies. questions about the structure and evolution of our universe. More As data sets grow it becomes of prime importance to create locally, massive surveys of stars have been undertaken to reveal efficient and automatic tools capable of producing robust results their properties, for example the Geneva-Copenhagen Survey for in a timely manner. A standard technique for estimating param- the solar neighborhood (Nordström et al. 2004) and the ELODIE eters from data is the maximum-likelihood method. In a survey library (Prugniel et al. 2007). These surveys provide a copi- such as SEGUE, which contains more than 240 000 stellar spec- ous amount of stellar photometry and spectra from the solar tra, each with more than 3000 flux measurements, it becomes ex- neighborhood, broadening the range of stellar types studied. The tremely time-consuming to do spectral fitting with a brute-force SEGUE catalog – Sloan Extension for Galactic Understanding search on the multi-dimensional likelihood surface, even more and Exploration (Yanny et al. 2009) – a component of SDSS, so if one wants to explore the errors on the recovered parame- contains additional imaging data at lower galactic latitudes, to ters. better explore the interface between the disk and halo popula- Efforts to reduce the computational burden of characteriz- tion. ing the likelihood surface include Markov-Chain Monte-Carlo By statistically analyzing the properties of these stars such methods (Ballot et al. 2006), where a chain is allowed to explore as chemical abundances, velocities, distances, etc., it has been the likelihood surface to determine the global solution. An al- possible to match the structure and evolution of the Milky Way ternative method is to start at an initial estimate and hope that to the current generation of galaxy formation models (Juricetal.´ the likelihood surface is smooth enough that a gradient search 2008; Ivezic´ et al. 2008; Bond et al. 2010; Wyse 2006). will converge on the solution. An example of this approach can Studies of large samples of stars of our galaxy are crucial be found in Allende Prieto et al. (2006)andGray et al. (2001), tests for the theory of the structure and formation of spiral galax- based on the Nelder-Mead downhill simplex method (Nelder & ies. Even though the statistics given by SEGUE or the Geneva- Mead 1965), where the derivatives of the likelihood function Copenhagen survey agree with the models and simulations of give the direction toward the maximum. In both cases, the like- galaxy formation, there is some contention over whether the ac- lihood is evaluated only partially, leaving large amounts of pa- curacies of measurements adequately support the conclusions. rameter space untouched. Moreover, the time needed to find the Article published by EDP Sciences Page 1 of 17 A&A 517, A57 (2010) maximum depends on the starting point and the steps used to If the noise has zero mean, X = μ, we can think of X as evaluate the next point. a random variable of a probability distribution L(X, Θ), which Another parameter estimation method utilizes neural net- depends in a known way on a vector Θ of m model parameters. works, for example Snider et al. (2001)andRe Fiorentin et al. In the present case m = 3 with (2007). While these non-linear regression models can obtain Θ = = quick accurate results, they are entirely dependent on the quality (θ1,θ2,θ3) ([Fe/H], Teff, log g), (2) of the training data, in which many parameters must be previ- / ff T ff ously known. Careful attention must also be paid to the sampling with the iron abundance [Fe H], e ective temperature e and surface gravity log g. A certain combination of the single param- of the model grid which is used to generate the neural network. = = Θ An alternative approach to spectral analysis is the Massively eters θ j ( j 1, 2, 3) also produces a theoretical model F F( ), Optimized Parameter Estimation and Data compression method and we can build an m-dimensional grid of theoretical models by varying the θ parameters. If the noise is Gaussian and the (MOPED1, Heavens et al. 2000). This novel approach to the max- j imum likelihood problem involves compressing both data and parameters have uniform priors, then the likelihood models to allow very rapid evaluation of a set of parameters. 1 −χ2 The evaluation is fast enough to do a complete search of the L f (X, Θ) = exp (3) N/2 2 2 parameter space on a finely resolved grid of parameters. Using (2π) σav carefully constructed linear combinations, the data are weighted 2 and the size is reduced from a full spectrum to only one num- gives the probability for the parameters, where σav is the aver- ber per parameter. This number, with certain caveats (discussed aged square of the noise of each data point and later), contains all the information about the parameter contained N (F − μ )2 in the full data. The method has been successfully applied in the χ2 = i i · (4) fields of CMB analysis (Bond et al. 1998), medical image reg- 2 i = 0 σi istration2 and galaxy shape fitting (Tojeiro et al. 2007). A com- plete background of the development of the MOPED algorithm The position of its maximum estimates the set of parameters Θ0 can be found in Tegmark et al. (1997, hereafter T97), Heavens that best describe the data X. The fit between F(Θ0)andX is et al. (2000, hereafter H00) and Panter et al. (2003). good if χ2 < d.o.f.,whered.o.f. = N − m are the degrees of free- We present a new derivative of MOPED,MAχ (MAssive dom. In the most basic form one finds the maximum in the likeli- compression of χ2), to analyze stellar spectra. To estimate the hood surface by exploring all points in the parameter space, with metallicity history of a galaxy for instance, MOPED needs mod- each likelihood estimation calculated using all N data points. els where the spectra are the sum of single stellar populations. This procedure is of course very time-consuming if N and m are Here we study one population, meaning the metallicity has to large. be estimated from the spectrum of each single star. It is there- fore necessary to develop a specific tool for this task: MAχ.In Sect. 2 we describe the method, giving a summary of the deriva- 2.2. Data compression tion of the algorithm. We then test the method in Sect. 3 on In practice not all data points give information about the param- the basic stellar atmosphere parameter estimation of a sample eters, because either they are noisy or not sensitive to the pa- of low-resolution stars of SEGUE, where we compare our re- rameter under study. The MOPEDalgorithm uses this knowledge sults with the SEGUE Stellar Parameter Pipeline in Sect.
Details
-
File Typepdf
-
Upload Time-
-
Content LanguagesEnglish
-
Upload UserAnonymous/Not logged-in
-
File Pages17 Page
-
File Size-