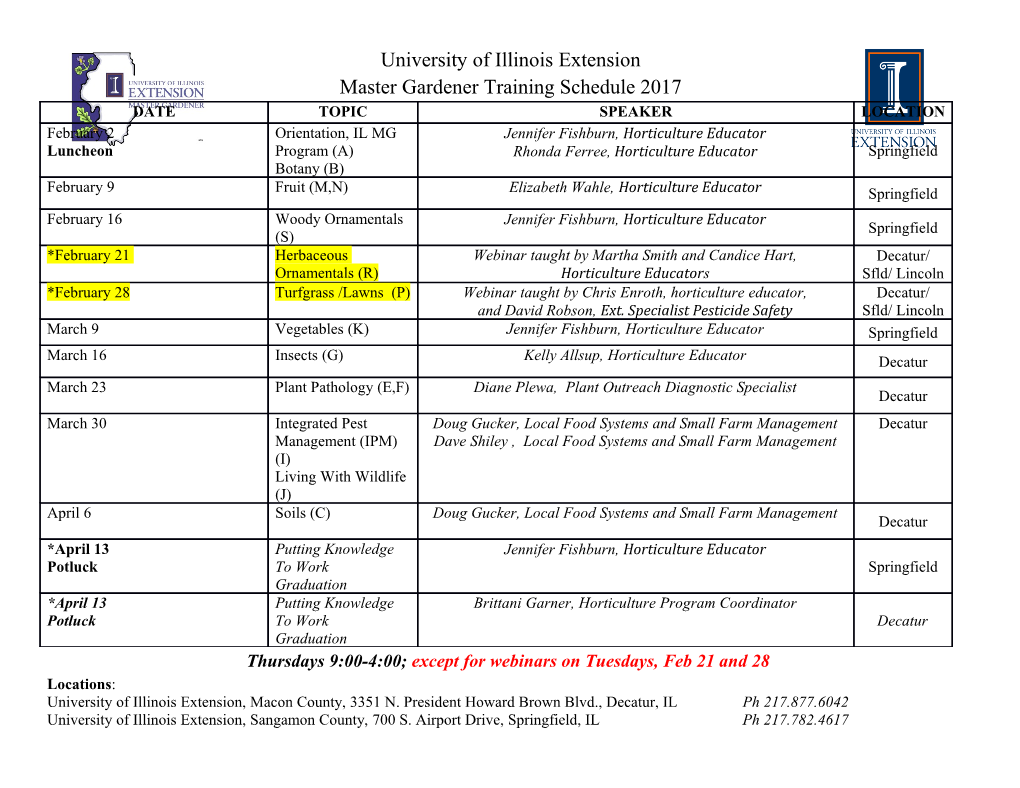
STAT Intro duction to Biostatistics Lecture Notes Intro duction Statistics and Biostatistics The eld of statistics The study and use of theory and metho ds for the analysis of data arising from random pro cesses or phenomena The study of how we make sense of data The eld of statistics provides some of the most fundamental to ols and techniques of the scientic metho d forming hyp otheses designing exp eriments and observational studies gathering data summarizing data drawing inferences from data eg testing hyp otheses A statistic rather than the eld of statistics also refers to a numerical quantity computed from sample data eg the mean the median the maximum Roughly sp eaking the eld of statistics can be divided into Mathematical Statistics the study and development of statistical theory and metho ds in the abstract and Applied Statistics the application of statistical metho ds to solve real problems involving randomly generated data and the development of new statistical metho dology motivated by real problems Read Ch of our text Biostatistics is the branch of applied statistics directed toward applica tions in the health sciences and biology Biostatistics is sometimes distinguished from the eld of biometry based up on whether applications are in the health sciences bio statistics or in broader biology biometry eg agriculture ecology wildlife biology Other branches of applied statistics psychometrics econometrics chemometrics astrostatistics environmetrics etc Why biostatistics Whats the dierence Because some statistical metho ds are more heavily used in health applications than elsewhere eg survival analysis longitudinal data analysis Because examples are drawn from health sciences Makes sub ject more app ealing to those interested in health Illustrates how to apply metho dology to similar problems en countered in real life We will emphasize the metho ds of data analysis but some basic theory will also be necessary to enhance understanding of the metho ds and to allow further coursework Mathematical notation and techniques are necessary No ap ologies We will study what to do and how to do it but also very imp ortant is why the metho ds are appropriate and what are the concepts justifying those metho ds The latter the why will get you further than the former the what Data Data Typ es Data are observations of random variables made on the elements of a p opulation or sample Data are the quantities numbers or qualities attributes measured or observed that are to be collected andor analyzed The word data is plural datum is singular A collection of data is often called a data set singular Example Low Birth Weight Infant Data App endix B of our text contains a data set called lowbwt contain ing measurements and observed attributes on low birth weight infants born in two teaching hospitals in Boston MA The variables measured here are sbp systolic blo o d pressure sex gender male female tox maternal diagnosis of toxemia yes no grmhem whether infant had a germinal matrix hemorrhage yes no gestage gestational age weeks apgar Apgar score measures oxygen deprivation at minutes after birth Data are repro duced on the top of the following page Read Ch of our text There are variables here sbp sex etc measured on unitselementssub jects the infants of a random sample of size An observation can refer to the value of a single variable for a par ticular sub ject but more commonly it refers to the observed values of all variables measured on a particular sub ject There are observations here Typ es of Variables Variable typ es can be distinguished based on their scale Typically dier ent statistical metho ds are appropriate for variables of dierent scales Scale Characteristic Question Examples Nominal Is A dierent than B Marital status Eye color Gender Religious aliation Race Ordinal Is A bigger than B Stage of disease Severity of pain Level of satisfaction Interval By how many units do A and B dier Temp erature SAT score Ratio How many times bigger than B is A Distance Length Time until death Weight Op erations that make sense for variables of dierent scales Op erations that make sense Addition Multiplication Scale Counting Ranking Subtraction Division p Nominal p p Ordinal p p p Interval p p p p Ratio Often the distinction between interval and ratio scales can be ig nored in statistical analyses Distinction between these two typ es and ordinal and nominal are more imp ortant Another way to distinguish between typ es of variables is as quantitative or qualitative Qualitative variables have values that are intrinsically nonnumeric categorical Eg Cause of death nationality race gender severity of pain mild mo derate severe Qualitative variables generally have either nominal or ordinal scales Qualitative variables can be reassigned numeric values eg male female but they are still intrinsically qualitative Quantitative variables have values that are intrinsically numeric Eg survival time systolic blood pressure number of children in a family height age body mass index Quantitative variables can be further sub divided into discrete and con tinuous variables Discrete variables have a set of p ossible values that is either nite or countably innite Eg numb er of pregnancies sho e size numb er of missing teeth For a discrete variable there are gaps between its p ossible val ues Discrete values often take integer whole numb ers values eg counts but some discrete variables can take noninteger values A continuous variable has a set of p ossible values including all values in an interval of the real line Eg duration of a seizure body mass index height No gaps between p ossible values The distinction between discrete and continuous quantitative variables is typically clear theoretically but can be fuzzy in practice In practice the continuity of a variable is limited by the precision of the measurement Eg height is measured to the nearest centimeter or p erhaps millimeter so in practice heights measured in millimeters only take integer values Another example survival time is measured to the nearest day but could theoretically be measured to any level of precision On the other hand the total annual attendance at UGA fo otball games is a discrete inherently integervalued variable but in prac tice can be treated as continuous In practice all variables are discrete but we treat some variables as continuous based up on whether their distribution can be well approximated by a continuous distribution Data Sources Data arise from exp erimental or observational studies and it is imp ortant to distinguish the two In an exp eriment the researcher delib erately imp oses a treatment on one or more sub jects or exp erimental units not necessarily hu man The exp erimenter then measures or observes the sub jects resp onse to the treatment Crucial element is that there is an intervention Example To assess whether or not saccharine is carcinogenic a re searcher feeds mice daily doses of saccharine After months of the mice have develop ed tumors By denition this is an exp eriment but not a very go o d one In the saccharine example we dont know whether with tumors is high b ecause there is no control group to which comparison can b e made Solution Select more mice and treat them exactly the same but give them daily doses of an inert substance a placeb o Supp ose that in the control group only mouse develops a tumor Is this evidence of a carcinogenic eect Mayb e but theres still a problem What if the mice in the groups dier systematically Eg group from genetic strain group from genetic strain Here we dont know whether saccharine is carcinogenic or if genetic strain is simply more susceptible to tumors We say that the eects of genetic strain and saccharine are con founded mixed up Solution Starting with relatively homogeneous similar mice ran domly assign to the saccharine treatment and to the control treat ment Randomization an extremely imp ortant asp ect of exp erimental de sign In the saccharine example we should start out with homoge neous mice but of course they will dier some Randomization ensures that the two exp erimental groups will b e probabilisti cally alike with resp ect to all nuisance variables p otential confounders Eg the distribution of body weights should be ab out the same in the two groups Another imp ortant concept esp ecially in human exp erimentation is blind ing An exp eriment is blind if the sub jects dont know which treatment they receive Eg supp ose we randomize of migraine suerers to an active drug and the remaining to a placeb o control treatment Exp eriment is blind if pills in the two treatment groups lo ok and taste identical and sub jects are not told which treatment they receive This guards against the placeb o eect An exp eriment is doubleblind if the researcher who administers the treatments and measures the resp onse do es not know which treat ment is assigned Guards against exp erimenter eects Exp erimenter may behave dierently toward the sub jects in the two groups or measure the resp onse dierently in the two groups Exp eriments are to be contrasted with observational studies No intervention Data collected on an existing system Less exp ensive Easier logistically More often ethically practical Interventions often not p ossible Exp eriments have many advantages and are strongly preferred when p ossible However exp eriments are rarely feasible in public healthepidemiology In health sciencesmedicine exp eriments involving humans
Details
-
File Typepdf
-
Upload Time-
-
Content LanguagesEnglish
-
Upload UserAnonymous/Not logged-in
-
File Pages100 Page
-
File Size-