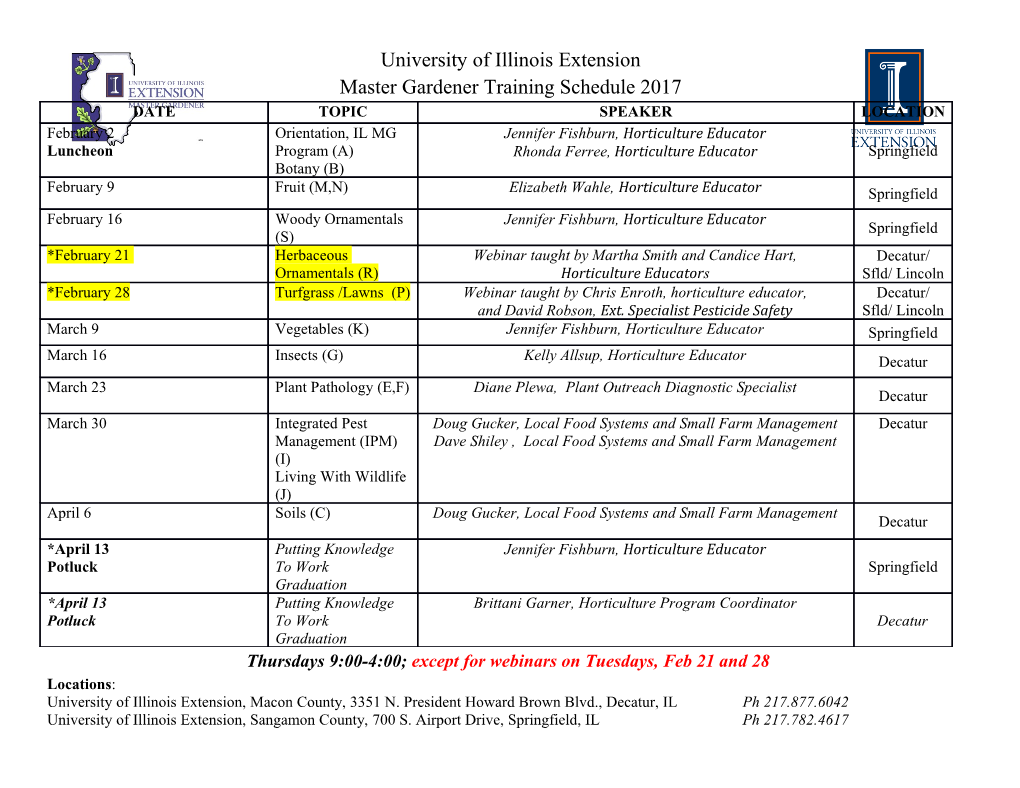
Advances in Reinforcement Learning and Their Implications for Intelligent Control Steven D. Whitehead*, Richard S. Suttoni and Dana H. Ballard* 1 Introduction 0 Robust under Incomplete and Uncertain Domain Knowledge: An intelligent control sys- What is an intelligent control system, and how will we tem must not depend upon a complete and accu- know one when we see it? This question is hard to rate domain model. Even for relatively simple, answer definitively, but intuitively what distinguishes narrow domains, it is extremely difficult to build intelligent control from more mundane control is the models that are complete and accurate [ShaSO]. complexity of the task and the ability of the control If control depends upon a domain model, it must system to deal with uncertain and changing conditions. suffice that the model be incomplete and inaccu- A list of the definitive properties of an intelligent con- rate. If domain knowledge is available, then the trol system might include some of the following: system should be capable of exploiting it, but it should not be a prerequisite for intelligent con- 0 Effective: The first requirement of any control system, intelligent or not, is that it generate con- trol. A system that uses a domain model that is trol actions that adequately control the plant (en- learned incrementally through experience is to be vironment). The adverb adequately is intention- preferred over one that relies upon a complete a ally imprecise since effective control rarely means priori model. optimal, but more often means sufficient for the Perceptual Feasibility: The information pro- purposes of the task. vided directly by a system's sensors is necessar- ily limited, and an intelligent control architecture 0 Reactive: Equally important is that a system's control be timely. Effective control is useless if must take this limitation into account. Instead of it is executed too late. In general, an intelligent assuming that any and all information about the control system cannot require arbitrarily long de- state of the environment is immediately available, lays between control decisions. On the contrary, intelligent system must be designed with limited it must be capable of making decisions on a mo- but flexible sensory-motor systems. Control algo- ments notice-upon demand. If extra time is rithms must consider actions for collecting neces- available that can be exploited to improve the sary state information as well as actions for af- quality of a decision, so much the better, but con- fecting change in the environment. trol decisions must be available any--lime [DB88]. 0 Mathematical Foundations: To facilitate per- formance analysis, it is important that an intelli- 0 Situated: An intelligent control system should be tightly coupled to its environment, and its con- gent control system be based on a framework that trol decisions must be based on the immediate sit- has a solid mathematical foundation. uation. A system must be capable of responding While the above list is not particularly surprising to unexpected contingencies and opportunities as or new, few if any control systems have been built they arise [AC87]. that satisfy all of the requirements. For example, 0 Adaptive An intelligent control system must use the problem-solving architectures that have been the its experience with the environment to improve dominate approach in Artificial Intelligence over the its performance. Adaptability allows a system to past twenty years fall well short of these goals. These refine an initially suboptimal control policy and problem-solving architectures are not particularly re- to maintain effective control in the face of non- active, situated, adaptive, or robust in the face of in- stationary environments. complete and inaccurate domain models. They also tend to make unrealistic assumptions about the ca- Department of Computer Science, University of pabilities of the sensory system. While extensions Rochester, Rochester NY 14627 and modifications to these architectures continue to 'GTE Laboratories Incorporated, Waltham, MA 02254 be popular, other approaches are being considered as 1289 TH0333-5/90/0000/1289$01.OO 0 1990 IEEE Authorized licensed use limited to: IEEE Xplore. Downloaded on January 30, 2009 at 17:01 from IEEE Xplore. Restrictions apply. well. (punishment). This paper focuses on control architectures that are Recent examples of controllers based on reinforce- based on reinforcement learning. In particular, we sur- ment learning include Barto et al.’s pole balancer vey several recent advances in reinforcement learning [BSA83, Sut841, Grefenstette’s simulated flight con- that have substantially increased its viability as a gen- troller [Greg01, Lin’s animats [Lingo], and Franklin’s eral approach to intelligent control. adapt,ive robot controllers [Fra88], among others. We begin by considering the relationship between re- inforcement learning and dynamic programming, both 2.1 Evaluating Reinforcement Learning viewed as methods for solving multi-stage decision problems [Wat89, BSWSO]. It is shown that many re- Reinforcement learning is emerging as an important alternative to classical problem-solving approaches to inforcement learning algorithms can be viewed as a kind of incremental dynamic programming; this pro- intelligent control because it possesses many of the properties for intelligent control that problem-solving vides a mathematical foundation for the study of rein- focrement learning systems. Connecting reinforcement approaches lack. In many respects the two approaches are complimentary and it is likely that eventual intel- learning to dynamic programming has also led to a ligent control architectures will incorporate aspects of strong optimal convergence theorem for one class of both.’ reinforcement learning algorithms and opens the door for similar analyses of other algorithms [Wat89]. Following is a discussion of the degree to which cur- Next we show how reinforcement learning methods rent reinforcement learning systems achieve each of the can be used to go beyond simple trial-and-error learn- properties that we associate with intelligent control. ing. By augmenting them with a predictive domain Effective: Reinforcement learning systems are model and using the model to perform a kind of in- effective in the sense that they eventually learn cremental planning, their learning performance can be effective control strategies. Although a system’s substantially improved. These control architectures initial performance may be poor, with enough in- learn both by performing experiments in the world teraction with the world it will eventually learn and by searching a domain model. ,Because the do- an effective strategy for obtaining reward. For main model need not be complete or accurate, it can the most part, the asymptotic effectiveness of re- be learned incrementally through experience with the inforcement learning systems has been validated world [SutSOa, SutSOb, WB89, Whi89, Lingo]. only empirically, however recent advances in the Finally, we discuss active sensory-motor systems theory of reinforcement learning have yielded for feasible perception and how these systems inter- mathematical results that guarantee optimality in act with reinforcement learning. We find that, with the limit for an important class of reinforcement some modification, many of the ideas from reinforce- learning systems [Wat89]. ment learning can be successfully combined with ac- tive sensory-motor systems. The system then learns Reactive: Decision-making in reinforcement not only an overt control strategy, but also where to learning systems is based on a policy func- focus its at,tention in order to collect necessary sensory tion which maps situations (inputs) directly into information [WBSOb]. actions (outputs) and which can be evaluated The results surveyed in this paper have been re- quickly. Consequently, reinforcement learning ported elsewhere (primarily in the machine learning systems are extremely reactive. literature). The objective here is to summarize them Situated: Reinforcement learning systems are and to consider their implications for the design of in- situated because each action is choser? based on telligent control architectures. the current state of the world. 2 Reinforcement Learning for Adaptive: Reinforcement learning systems are adaptive because they use feedback to improve Intelligent Control their performance. What is reinforcement learning? A reinforcement Incomplete and Uncertain Domain Knowl- learning system is any system that through interac- edge: Reinforcement learning systems do not de- tion with its environment improves its performance by pend upon internal domain models because they receiving feedback in the form of a scalar reward (or learn through trial-and-error experience with the penalty) that is commensurate with the appropriate- world. However, when available, they can ex- ness of the response. By improves its performance, ploit domain knowledge by 1) using prior knowl- we mean that the system uses the feedback to adapt edge about the control task to determine a good its behavior in an effort to maximize some measure of the reward it receives in the future. Intuitively, ‘To some extent this int,egration has already begun to a reinforcement learning system can be viewed as a occur, with the development of reinforcement learning sys- hedonistic automaton whose sole objective is to maxi- tem that learn and use internal domain models to improve mize the positive (reward) and minimize the
Details
-
File Typepdf
-
Upload Time-
-
Content LanguagesEnglish
-
Upload UserAnonymous/Not logged-in
-
File Pages9 Page
-
File Size-