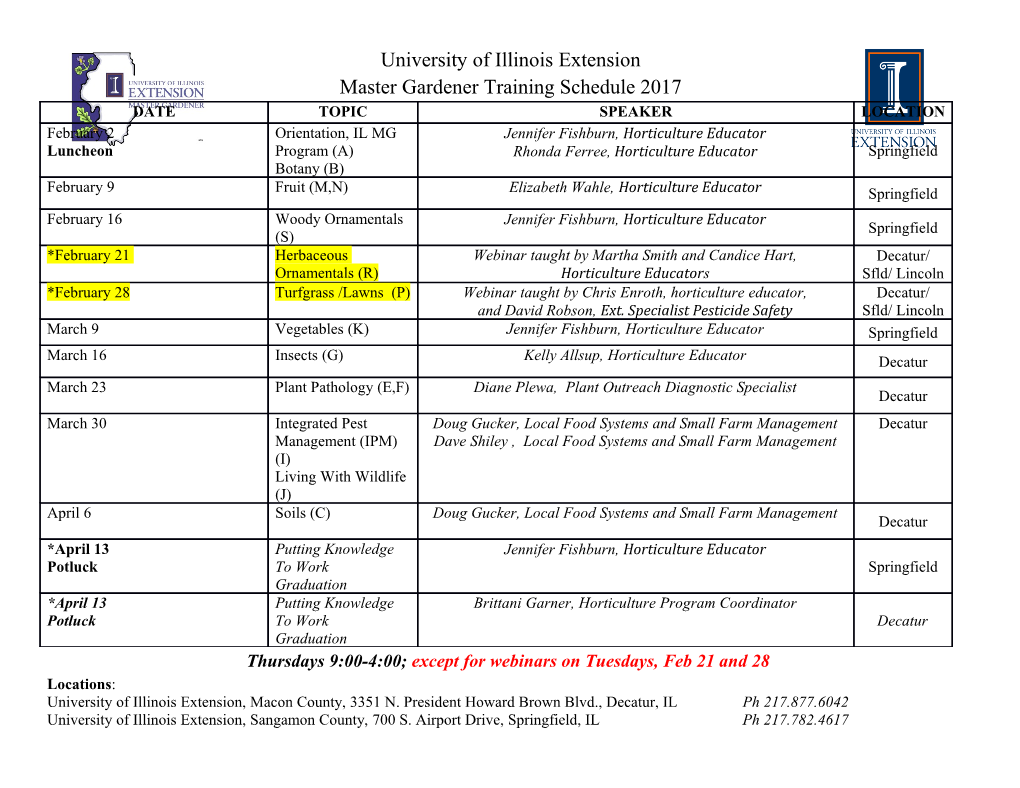
Introduction to Biosystematics - Zool 575 Introduction to Biosystematics Outline Lecture 22 - Performance: ML & MP 1. Mechanistic comparison with Parsimony - branch lengths & parameters 2. Performance comparison with Parsimony - Desirable attributes of a method - The Felsenstein and Farris zones Derek S. Sikes University of Calgary Zool 575 Lack of a reproducible method resulted in three Statistical Phylogenetics major approaches: (Explosion in 1960s) - Grew from mathematical, computer, evolutionary, numerical studies, not as much from systematics 1. Phenetics - similarity / distances only, not evolution, not phylogeny 1. Parsimony (Cavalli-Sforza & Edwards, 1963) [uncorrected distance data] tree with minimum changes preferred 2. Cladistics - phylogeny inferred using characters & parsimony 2. Maximum Likelihood (Cavalli-Sforza & [+/- uncorrected character data] Edwards, 1964) 3. Statistical Phylogenetics - phylogeny tree that maximizes probability of the data is inferred using corrected data & “best fitting preferred model” [both distance & character data] Made available for DNA based phylogenetics by Felsenstein in the early 1980s Optimality Criteria - Given 2+ trees ML comparison with Parsimony (MP) 1. The most parsimonious topology is often, but not Maximum Parsimony always, the same as the maximum likelihood The tree hypothesizing the fewest topology number of character state changes is 2. Parsimony does not correct the data for unobserved changes and so parsimony branch lengths are the best typically underestimates of actual branch lengths - Parsimony branch lengths = the estimated number Maximum Likelihood of changes that occurred & are mapped onto The tree maximizing the probability the tree after it has been found (not used during tree searching - only total tree length is used to of the observed data is best select the best tree) 1 Introduction to Biosystematics - Zool 575 ML comparison with Parsimony (MP) ML comparison with Parsimony (MP) 3. Because Parsimony ignores branch length 4. Parsimony minimizes homoplasy information during searches it is - ML will sometimes prefer a tree with more than the - much faster than ML minimum amount of homoplasy (if it makes the data - unable to use this information to help it find the more likely given the model) most probable tree (some strict cladists argue that they do not use parsimony to find the most - Again, it is branch lengths which indicate where probable tree, they claim there is no connection homoplasy may be common in the tree - longer between minimal tree length and probability of branches are more likely to be involved in homoplastic being correct, “truth is unknowable”) events than shorter ones - especially unable to use branch lengths (~ rates of change) to detect areas of the tree that are [Note: Parsimony is sometimes called Maximum Parsimony likely to experience higher rates of homoplasy and abbreviated MP in contrast to ML] than other regions ML comparison with Parsimony (MP) Outline Parsimony would never prefer the correct, but longer 1. Mechanistic comparison with Parsimony tree on the left whereas ML would (more on this later) - - branch lengths & parameters Parsimony also ignores autapomorphies 2. Performance comparison with Parsimony autapomorphies - Desirable attributes of a method A B A B - The Felsenstein and Farris zones 3 1 1 1 2 2 2 ML 3 MP 3 C D C D 2 convergence events 1 convergence event Desirable Attributes of a method Desirable Attributes of a method 1. Consistency 1. Consistency 2. Efficiency 3. Robustness - a method is consistent if it converges on the 4. Computational Speed correct tree as the data set becomes infinitely 5. Discriminating ability large (ie there is no systematic error) 6. Versatility - all methods are consistent if their assumptions are met (ie their model is not - Some can be assessed using simulations violated) - Data are simulated and later analyzed using a method to determine its attributes - conversely, model misspecification can cause any method to be inconsistent 2 Introduction to Biosystematics - Zool 575 Desirable Attributes of a method Desirable Attributes of a method 1. Consistency 2. Efficiency (cont.) - inconsistency manifests as the preference for - some methods are consistent but not very the wrong tree as the data become infinitely efficient (require TONS of data to work) numerous - MP, when its assumptions are not violated, is far more efficient than ML 2. Efficiency 3. Robustness - how quickly a method obtains the correct solution (how many data it needs to work) - a method is robust if it is relatively insensitive - tradeoff between consistency & efficiency to violations of its assumptions (how easily does it become inconsistent?) Desirable Attributes of a method Desirable Attributes of a method 4. Computational speed 5. Discriminating ability (cont.) - clustering methods are very fast, optimality - ability to discriminate among alternative criterion methods are much slower, and ML is hypotheses - increases with decreasing speed the slowest method known - clustering methods (eg NJ) - problem: ML has far better discriminating 1. Do not guarantee an optimal solution ability than NJ but is so computationally 2. Do not permit the comparison of intense it cannot evaluate as much tree space alternative solutions (cannot compare as many alternative trees) as faster methods like MP 5. Discriminating ability - ability to discriminate among alternative - may limit its applicability (but see future hypotheses - increases with decreasing speed lectures on Bayesian methods) Desirable Attributes of a method A comment on data 6. Versatility When a single dataset has multiple - what kinds of data can be analyzed? components - eg morphology and DNA - MP (before 2001) had this as a huge advantage over ML these are called partitions of the data - mixed analyses of morphology & DNA - behavioral data unweighted MP treats all character state - can weight different DNA characters changes, whether morphology or DNA, the same - now (since 2001) we can do all this with ML - morphology, behavior, etc - mixed dataset analyses - different models for different genes or types of data 3 Introduction to Biosystematics - Zool 575 A comment on data - Partitions Back to performance attributes… ML methods, esp Bayesian, allow us to assign Choosing a method requires balancing the different models to different partitions importance of all these attributes This greatly increases the fit of the meta- eg clustering is good to get starting trees or to model (combination of all sub-models) to explore the data quickly before doing a the data longer analysis New mixture-model methods (Bayesian) eg MP might be used to explore tree-space allow the data to tell the investigator how thoroughly and obtain starting trees and many distinct data partitions are present parameters to feed into a slow ML search (more on this later) Back to performance attributes… Outline But the attribute most relevant to 1. Mechanistic comparison with Parsimony phylogenetic accuracy is determining if - branch lengths & parameters the assumptions of the method are met by the data - Model Fitting! 2. Performance comparison with Parsimony - Desirable attributes of a method If not, then one risks model misspecification - The Felsenstein and Farris zones and potential inconsistency (or at least poor performance) of the method If not, then all the other attributes matter little (except the method’s robustness to violation of assumptions!) The Felsenstein & Farris Zones Long Branch Attraction • Felsenstein (1978) used simulated DNA evolved on a simple model Felsenstein (1978) demonstrated that MP phylogeny of four taxa with a mixture of short and long branches would be inconsistent in a region of tree • Parsimony will give the wrong tree [misled by convergence] space now called the “Felsenstein Zone” A Model tree B Parsimony tree Long branches are - Long Branch Attraction (LBA) C Rates or A attracted but the p p Branch lengths similarity is q p >> q homoplastic Wrong Siddall (1998) dubbed the opposite region of q q C D B D tree space, where he hoped ML would be • With more data the certainty that parsimony will give the inconsistent, the “Farris Zone” wrong tree increases - parsimony is statistically biased and - Long Branch Repulsion???? inconsistent “positively misleading” • Cladists claimed that Felsenstein’s results were unrealistic • Few empirical examples known 4 Introduction to Biosystematics - Zool 575 Results of simulations Long Branch Repulsion? Or lack of LBA? With Felsenstein zone • Siddall (1998) claimed to have demonstrated that when long branches trees are sister taxa ML will fail to find the correct tree more often than random choice (worse than random). He suggested the long branches repelled each other and ML was failing the same way MP fails • He called this region of treespace in which ML supposedly fails and MP never fails “the Farris Zone” in honor of Steve Farris, a great advocate of MP • Swofford et al. (2001) demonstrated, in reply: • That, ML, will, as theory predicts prefer the correct tree, given enough data • ie it is not statistically inconsistent • Repulsion does not happen for ML - and neither does LBA • MP prefers the correct tree (with artifactually inflated branch support) due to LBA - ie its bias works in its favor Farris Zone - even JC69 shows consistency with enough data Felsenstein zone Tree > - s e r u l i a F MP succeeds because it interprets homoplasy as homology Farris zone tree There is no “Long Branch Repulsion” MP chooses the wrong
Details
-
File Typepdf
-
Upload Time-
-
Content LanguagesEnglish
-
Upload UserAnonymous/Not logged-in
-
File Pages10 Page
-
File Size-