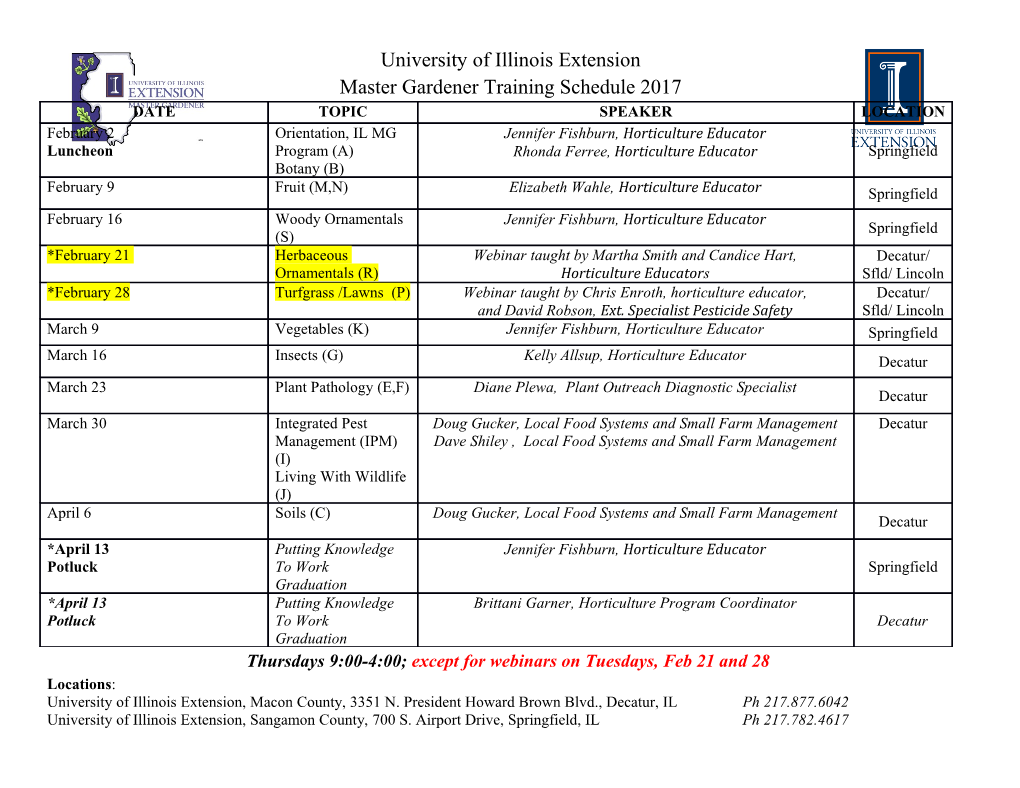
Temporally-Reweighted Chinese Restaurant Process Mixtures for Clustering, Imputing, and Forecasting Multivariate Time Series Feras A. Saad Vikash K. Mansinghka Probabilistic Computing Project Probabilistic Computing Project Massachusetts Institute of Technology Massachusetts Institute of Technology Abstract there are tens or hundreds of time series. One chal- lenge in these settings is that the data may reflect un- derlying processes with widely varying, non-stationary This article proposes a Bayesian nonparamet- dynamics [13]. Another challenge is that standard ric method for forecasting, imputation, and parametric approaches such as state-space models and clustering in sparsely observed, multivariate vector autoregression often become statistically and time series data. The method is appropri- numerically unstable in high dimensions [20]. Models ate for jointly modeling hundreds of time se- from these families further require users to perform ries with widely varying, non-stationary dy- significant custom modeling on a per-dataset basis, or namics. Given a collection of N time se- to search over a large set of possible parameter set- ries, the Bayesian model first partitions them tings and model configurations. In econometrics and into independent clusters using a Chinese finance, there is an increasing need for multivariate restaurant process prior. Within a clus- methods that exploit sparsity, are computationally ef- ter, all time series are modeled jointly us- ficient, and can accurately model hundreds of time se- ing a novel \temporally-reweighted" exten- ries (see introduction of [15], and references therein). sion of the Chinese restaurant process mix- ture. Markov chain Monte Carlo techniques This paper presents a nonparametric Bayesian method are used to obtain samples from the poste- for multivariate time series that aims to address some rior distribution, which are then used to form of the above challenges. The model is based on two ex- predictive inferences. We apply the tech- tensions to Dirichlet process mixtures. First, we intro- nique to challenging forecasting and imputa- duce a recurrent version of the Chinese restaurant pro- tion tasks using seasonal flu data from the US cess mixture to capture temporal dependences. Sec- Center for Disease Control and Prevention, ond, we add a hierarchical prior to discover groups of demonstrating superior forecasting accuracy time series whose underlying dynamics are modeled and competitive imputation accuracy as com- jointly. Unlike autoregressive models, our approach is pared to multiple widely used baselines. We designed to interpolate in regimes where it has seen further show that the model discovers inter- similar history before, and reverts to a broad prior in pretable clusters in datasets with hundreds of previously unseen regimes. This approach does not time series, using macroeconomic data from sacrifice predictive accuracy, when there is sufficient the Gapminder Foundation. signal to make a forecast or impute missing data. We apply the method to forecasting flu rates in 10 US regions using flu, weather, and Twitter data from the 1 Introduction US Center for Disease Control and Prevention. Quan- titative results show that the method outperforms sev- Multivariate time series data is ubiquitous, arising in eral Bayesian and non-Bayesian baselines, including domains such as macroeconomics, neuroscience, and Facebook Prophet, multi-output Gaussian processes, public health. Unfortunately, forecasting, imputation, seasonal ARIMA, and the HDP-HMM. We also show and clustering problems can be difficult to solve when competitive imputation accuracy with widely used sta- st tistical techniques. Finally, we apply the method to Proceedings of the 21 International Conference on Ar- clustering hundreds of macroeconomic time series from tificial Intelligence and Statistics (AISTATS) 2018, Lan- zarote, Spain. PMLR: Volume 84. Copyright 2018 by the Gapminder, detecting meaningful clusters of countries author(s). whose data exhibit coherent temporal patterns. Temporally-Reweighted Chinese Restaurant Process Mixtures for Multivariate Time Series 2 Related Work 3 Temporally-Reweighted Chinese Restaurant Process Mixture Model The temporally-reweighted Chinese restaurant process (TRCRP) mixture we introduce in Section3 can be We first outline the notations and basic setup as- directly seen as a time series extension to a family of sumed throughout this paper. Let xn : n = 1;:::;N nonparametric Bayesian regression models for cross- denote a collection of N discrete-timef series, whereg th n sectional data [18, 35, 28, 22, 23]. These methods the first T variables of the n time series is x1:T = n n n operate on an exchangeable data sequence xi with (x1 ; x2 ; : : : ; xT ). Slice notation is used to index sub- f g n n n exogenous covariates yi ; the prior CRP cluster prob- sequences of variables, so that x = (x ; : : : ; x ) f g t1:t2 t1 t2 ability p(zi = k) for each observation xi is reweighted for t1 < t2. Superscript n will be often be omit- based on yi. Our method extends this idea to a time ted when discussing a single time series. The re- series xt ; the prior CRP cluster probability p(zt = k) mainder of this section develops a generative pro- f g for xt is now reweighted based on the p previous val- cess for the joint distribution of all random variables n ues xt 1:t p. Moreover, the hierarchical extension in xt : t = 1; : : : ; N; n = 1;:::;N in the N time series, − − Section 3.4 coincides with CrossCat [21], when all tem- whichf we proceed to describe ing stages. poral dependencies are removed (by setting p = 0). Temporal extensions to the Dirichlet process have been 3.1 Background: CRP representation of previously used in the context of dynamic clustering Dirichlet process mixture models [38,1]. The latter work derives a recurrent CRP as the limit of a finite dynamic mixture model. Unlike Our approach is based on a temporal extension of the the method in this paper, those models are used for standard Dirichlet process mixture (DPM), which we clustering batched data and dynamic topic modeling review briefly. First consider the standard DPM in the [7], rather than data analysis tasks such as forecasting non-temporal setting [11], with concentration α and or imputation in real-valued, multivariate time series. base measure πΘ. The joint distribution of a sequence of m exchangeable random variables (x ; : : : ; x ) is: For multivariate time series, recent nonparametric 1 m Bayesian methods include using the dependent Dirich- P DP (α; πΘ); θ∗ P P; xj θ∗ F ( θ∗): let process for dynamic density estimation [31]; hier- ∼ j j ∼ j j ∼ · j j archical DP priors over the state in hidden Markov models [HDP-HMM; 12, 19]; Pitman-Yor mixtures of The DPM can be represented in terms of the Chi- non-linear state-space models for clustering [26]; and nese restaurant process [2]. As P is almost-surely dis- crete, the m draws θ∗ P contain repeated val- DP mixtures [8] and Polya trees [27] for modeling noise j ∼ distributions. As nonparametric Bayesian extensions ues, thereby inducing a clustering among data xj. Let of state-space models, all of these approaches specify λF be the hyperparameters of πΘ, θk be the unique f g priors that fall under distinct model classes to the one values among the θj∗ , and zj denote the cluster as- developed in this paper. They typically encode para- signment of xj which satisfies θj∗ = θzj . Define njk metric assumptions (such as linear autoregression and to be the number of observations xi with zi = k for hidden-state transition matrices), or integrate explicit i < j. Using the conditional distribution of zj given previous cluster assignments z1:j 1, the joint distribu- specifications of underlying temporal dynamics such − as seasonality, trends, and time-varying functionals. tion of exchangeable data sequence (x1; x2;::: ) in the Our method instead builds purely empirical models CRP mixture model can be described sequentially: and uses simple infinite mixtures to detect patterns iid in the data, without relying on dataset-specific cus- θk πΘ( λF ) tomizations. As a multivariate interpolator, the TR- f g ∼ · j CRP mixture is best applied to time series where there Pr [zj = k z1:j 1; α](j = 1; 2;::: ) is no structural theory of the temporal dynamics, and ( j − njk if 1 k max (z1:j 1) where there is sufficient statistical signal in the history ≤ ≤ − / α if k = max (z1:j 1) + 1 of the time series to inform probable future values. − (1) To the best of our knowledge, this paper presents the first multivariate, nonparametric Bayesian model that xj zj; θk F ( θzj ) j f g ∼ ·| provides strong baseline results without specifying cus- tom dynamics on a problem-specific basis; and that The CRP mixture model (1), and algorithms for pos- has been benchmarked against multiple Bayesian and terior inference, have been studied extensively for non- non-Bayesian techniques to cluster, impute, and fore- parametric modeling in a variety of statistical applica- cast sparsely observed real-world time series data. tions (for a survey see [37], and references therein). Saad and Mansinghka α; λG values are a-priori more likely to have similar distribu- tions for generating xt1 and xt2 , because G increases the probability that zt = zt = k , so that xt and f 1 2 g 1 λF xt2 are both drawn from F ( θk). z1 z2 z3 z4 ·| ··· Figure1 shows a graphical model for the TRCRP mix- ture (2) with window size p = 1. The model pro- ceeds as follows: first assume the initial p observations x x x x x θk 0 1 2 3 4 (x p+1; : : : ; x0) are fixed or have a known joint dis- ··· − k=1; 2;::: tribution. At step t, the generative process samples a cluster assignment zt, whose probability of joining Figure 1: Graphical model for the TRCRP mixture cluster k is a product of (i) the CRP probability for in a single time series x = (x ; x ;::: ) with lagged zt = k given all previous cluster assignments z1:t 1, 1 2 f g − and (ii) the \cohesion" term G(xt p:t 1; λG;Dtk).
Details
-
File Typepdf
-
Upload Time-
-
Content LanguagesEnglish
-
Upload UserAnonymous/Not logged-in
-
File Pages10 Page
-
File Size-