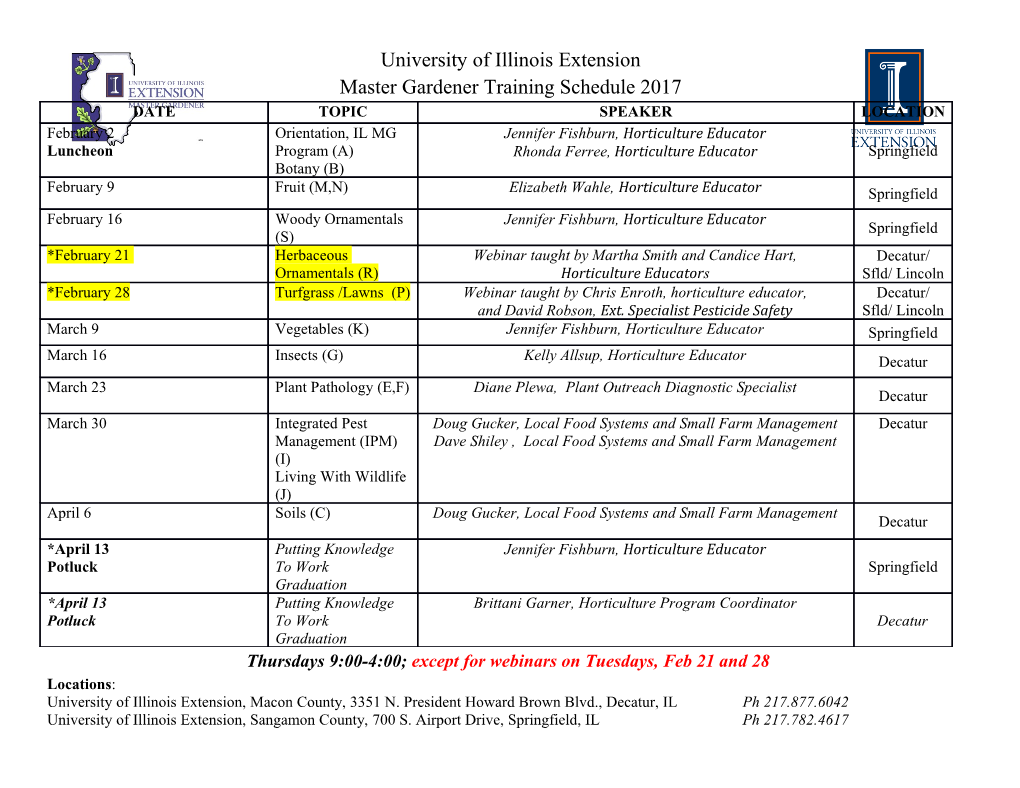
From: ISMB-93 Proceedings. Copyright © 1993, AAAI (www.aaai.org). All rights reserved. Genetic Algorithms for DNA Sequence Assembly Rebecca Parsons" Stephaniet Forrest Christian$ Burks Abstract Gallant et al. have shown that the determination of the shortest commonsuperstring (SCS) for a four- This paper describes a genetic algorithm appli- letter alphabet, in which the fragment a.s~sembly prob- cation to the DNAsequence assembly problem. lem can be recast, is NP-complete (i.e., computation- The genetic algorithm uses a sorted order repre- ally infeasible for large data sets) [Gallant et al., 1980]. sentation for representing the orderings of frag- Even the over-simplified, reduced approach of mod- ments. Twodifferent fitness functions, both based eling fragment assembly as determining the order of on pairwise overlap strengths between fragments, beads (fragments) on a string is NP-complete.~ The are tested. The paper concludes that the ge- determination of overlap strengths is non-trivial, re- netic algorithm is a promising method for frag- quiring O(N2) operations for N fragments [Churchill ment assembly problems, achieving usable solu- et al., 1993]. In particular, these bottlenecks are signifi- tions quickly, but that the current fitness functions cant for the proposed sequence throughput levels of the are flawed and that other representations might be s 1 humangenome project (10 - 107 bp/day) [Hunkapiller more appropriate. et al., 1991]. Keywords: genetic algorithm, DNAsequence as- The assembly of overlapping sets of fragments into sembly, human genome project, ordering prob- large segments of contiguous sequence, described as lems, random key representation. contigs [Staden, 1980], requires the pairwise align- ment of the fragments among themselves, followed by Introduction the synthesis of these pairwise results into an opti- Computers are playing an increasing role in large- mal global alignment, expressed as a consensus se- scale sequencing projects [Burks, 1989; Cinkosky et quence. Randomized search methods have recently al., 1991]. The scope of these projects makes it im- been successfully applied to the fragment assembly practical to assemble by hand the resulting sequence problem [Churchill et al., 1993]. In this paper, we fragments into a coherent consensus sequence. Fur- extend this work to include genetic algorithms. We thermore, the computational complexity of the task report our initial results using the genetic algorithm to makes software tools based on emulation of the man- generate orderings of DNAsequence fragments. The goal is to maximize the overlap strengths of adjacent ual approach increasingly impractical. For example, pairs in the resulting layout. *Los AlamosNational Laboratory, Computer Research Applications Group, MSB265, Los Alamos, NM87545. Introduction to Genetic Algorithms Emall: [email protected], phone: (505) 667-2655, Fax: Genetic algorithms (GAs) are a biased sampling algo- (505) 665-5220 (Corresponding Author) tDepaxtment of Computer Science, University of New rithm based on the Darwinian notions of variation, dif- Mexico, Albuquerque, NM. 87131-1386 Emait: for- ferential reproduction, and inheritance [llolland, 1975; [email protected], phone: (505) 277-3112 Goldberg, 1989]. Computation in a GA proceeds by ~Los Alamos National Laboratory, The- creating successive populations of individuals, gener- oretical Biology and Biophysics Group, MSK710.Email: ally bit strings, with each individual representing a po- [email protected], phone: (505) 667-6683 tential solution to the problem. To generate a new pop- 1This work was performed under the auspices of the ulation from an existing one, individuals are selected DOE. C.B. was supported in part by the DOEGenome in proportion to their fitness; as a consequence, less Projgct (ERW-F137;R. Moyzis, P.I.); R.P. was supported fit individuals have few if any representatives (copies) in part by a Los Alaanos Director’s Fellowship; and S.F. in the new population and more fit individuals have was supported by National Science Foundation (grant IRI- 9157644), and Sandia University Research Program (grant 2Theproof of this involves a reduction to the Hamilto- AE-1679). nian Path problem. 310 ISMB-93 manyrepresentatives. Variation is introduced through such that the crossover operation produces a legal lay- two operators: crossover and mutation. Crossover sim- out. The obvious mapping from bit strings to layouts, ply exchanges substrings between two individuals, cre- which for n fragments, uses k bits (2 k-1 < n < 2~) ating hybrids which replace their parents, while muta- for each fragment in the layout and n fragment posi- tion flips the value of individual bits. Each individual’s tions, does not maintain legal layouts using standard fitness is evaluated, and the procedure is iterated. A crossover. Nothing in the crossover operation prevents cycle of evaluation, reproduction, crossover and muta- the same fragment designator from appearing more tion is called a generation. Populations are typically than once in the new individual, even if it only ap- initialized with randomly generated strings, and over peared once in each of the parents. time, the GAprocedure usually evolves populations of There are two ways to approach this problem: a spe- highly fit individuals. cialized crossover function which only produces legal A commoncrossover operator is two-point crossover layouts, or a different mappingfrom bit strings to lay- in which two crossover points are randomly selected, outs. A number of authors explore different, represen- and the bits between the two crossover points are ex- tations but concentrate on different crossover operators changed, creating two new children. The purpose of for TSP[Grefenstette et ai., 1985; Oliver et al., 1987; crossover is to take two good partial solutions and com- Whitley, 1989; Muhlenbein, 1990]. bluhlenbein also bine their parts to produce, sometimes, a better solu- proposes a change in the overall search strategy of tion. The purpose of mutation is both to explore ran- the GA by clustering members of the population and dom parts of the search space and to allow for the re- performing a smaller number of more directed local introdllction of "genetic" material that may have died searches. off in an earlier generation. Without mutation and Wechoose the second approach---selecting a differ- crossover, no new points in the search space would be ent mapping from bit strings to layouts. This map- explored. For details on the implementation of genetic ping, a modification of one being studied by several algorithms, the reader is referred to [Goldberg, 1989; authors [Bean, 1992; Syswerda, 1989; Schaffer et ai., Davis, 1991]. 1989], ensures that all bit strings represent a legal lay- A primary consideration in the application of GAsto out. Thus, the standard two-point crossover operator, a particular problem is the selection of a representation or any crossover operator, always produces a legal lay- for candidate solutions. The obvious representation of out. Our representation is illustrated in Figure 1. For a layout, an ordered sequence of numbers representing a layout problem consisting of n fragments, select a the fragments, is not necessarily appropriate for the value k such that 2k > n, a number of bits sufficient fragment assembly problem; we address this issue in to represent a number up to n. Increasing the value the next section. Another crucial consideration in the of k beyond the minimum required may slightly im- design of a GAfor a particular problem is the creation prove performance [Parsons and Burks, 1993]. The bit of a function to evaluate the fitness of an individual. In string required is then m = k * (n + 1) bits in length. this paper, we report on two different fitness measures Mappinga bitstring bl, b2, ..., b,n to a layout of n frag- for the fragment assembly problem. ments fl, f2, ..., fn, proceeds by considering the string as a sequence of n + 1 key values, each of k bits. The Representation Issues first n keys are then sorted. If the key value in position The fragment assembly problem is closely related to j of the individual appears in position i of the sorted the familiar combinatorial optimization problem, the list, then fragment j is in position i of the intermedi- traveling salesperson (TSP) [Lawler et al., 1985]. Like ate layout. The last key in the individual specifies the TSP, the fragment assembly problem restricts legal starting position in the intermediate layout; the final solutions to those in which each fragment (city) ap- layout is simply a rotation of the intermediate layout pears exactly once. In addition, both problems have with the fragment in the specified starting position as a measure of how desirable it is to have two frag- the first fragment in the final layout. This starting po- ments (cities) adjacent in the final solution. In the sition is required to allow crossover to swap fragments case of fragment assembly, this measure is the over- from one end of the layout to the other. Since the lap strength [Churchill e~ al., 1993], which essentially layout is linear, standard crossover would not perform represents the number of overlapping contiguous base this operation without the starting position. pairs between the two fragments. One significant dif- As an example, consider a bit string (see Figure 1) ference between the two problems is that a legal tour which produces the following integers, using 3 bits per in the TSPis a circle; thus, all starting points on the fragment: 2 7 1 5 3 2. Under this mapping, the frag- circle represent equivalent solutions. In contrast, a lay- ment layout represented by this bit string is 1 5 4 2 3 out has definite starting and ending points. This issue with an intermediate layout of 3 1 5 4 2. Since the low- is discussed at the end of this section. est value, 1, appears in the third position of the string, Unfortunately, TSP and the fragment assembly the first fragment in the intermediate layout, is 3. The problem are not easily amenable to a GA approach.
Details
-
File Typepdf
-
Upload Time-
-
Content LanguagesEnglish
-
Upload UserAnonymous/Not logged-in
-
File Pages9 Page
-
File Size-