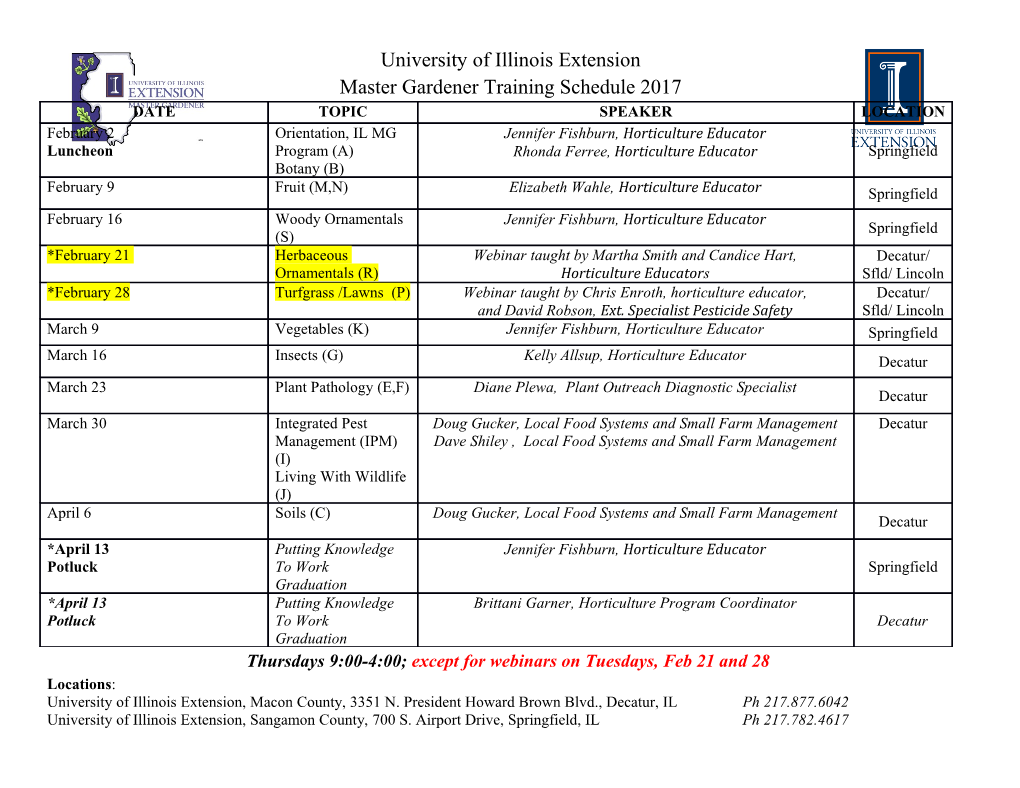
Örebro University Örebro University School of Business Statistics, Paper, Second level, 15 Credits Supervisor: Panagiotis Mantalos Examiner: Thomas Laitila Spring 2013 Testing for Cointegration in Multivariate Time Series An evaluation of the Johansens trace test and three different bootstrap tests when testing for cointegration Jonas Englund 880131 Abstract In this paper we examine, by Monte Carlo simulation, size and power of the Johansens trace test when the error covariance matrix is nonstationary, and we also investigate the properties of three different bootstrap cointegration tests. Earlier studies indicate that the Johansen trace test is not robust in presence of heteroscedasticity, and tests based on resampling methods have been proposed to solve the problem. The tests that are evaluated is the Johansen trace test, nonparametric bootstrap test and two different types of wild bootstrap tests. The wild bootstrap test is a resampling method that attempts to mimic the GARCH model by multiplying each residual by a stochastic variable with an expected value of zero and unit variance. The wild bootstrap tests proved to be superior to the other tests, but not as good as earlier indicated. The more the error terms differs from white noise, the worse these tests are doing. Although the wild bootstrap tests did not do a very bad job, the focus of further investigation should be to derive tests that does an even better job than the wild bootstrap tests examined here. Key words: Johansen trace test, wild bootstrap, cointegration, heteroscedasticity, simulation. Table of contents 1. INTRODUCTION ................................................................................................................ 1 2. THEORY .............................................................................................................................. 3 2.1 Random walk .................................................................................................................. 3 2.2 The vector autoregressive model .................................................................................... 3 2.3 The generalized autoregressive conditional heteroscedasticity model ........................... 3 2.4 Integration and cointegration .......................................................................................... 4 2.5 The vector error correction model .................................................................................. 5 2.6 The Johansen trace statistic ............................................................................................ 5 2.7 Bootstrap ......................................................................................................................... 6 2.7.1 Nonparametric bootstrap ......................................................................................... 6 2.7.2 Wild bootstrap ......................................................................................................... 7 3 METHOD .............................................................................................................................. 9 3.1 Data generating processes .............................................................................................. 9 3.1.1 Standard random walk ............................................................................................. 9 3.1.2 Random walk with a break in the variance.............................................................. 9 3.1.3 Random walk with GARCH effects ........................................................................ 9 3.1.4 Vector error correction model ............................................................................... 10 3.1.5 Vector error correction model with a break in the variance .................................. 10 3.1.6 Vector error correction model with GARCH effects............................................. 10 3.1.7 Comparative simulations ....................................................................................... 10 3.2 Monte Carlo algorithms ................................................................................................ 10 3.2.1 Algorithm for evaluating the Johansens trace test ................................................. 11 3.2.2 Algorithm for evaluating the bootstrap tests ......................................................... 11 3.2.3 Rescaling of the residuals ...................................................................................... 11 4. RESULTS ........................................................................................................................... 12 4.1 Size ............................................................................................................................... 12 4.2 Power ............................................................................................................................ 14 4.3 Comparative results ...................................................................................................... 16 5. DISCUSSION ..................................................................................................................... 18 5.1 Conclusion .................................................................................................................... 18 6. REFERENCES ................................................................................................................... 19 Appendix: P-value plots ......................................................................................................... 20 1 1. INTRODUCTION In this paper we examine the size and power of the Johansens trace test and three bootstrap tests when testing for cointegration when the error terms in the series exhibit certain behavior. In earlier studies, this “certain behavior” has been accompanied by using a simple break in the variance as well as a generalized autoregressive conditional heteroscedasticity model (Mantalos, 2001; Cavaliere & Taylor, 2008); this kind of certain behavior of the error terms is also used in this study. To begin with, a short introduction of time series analysis along with its methods and models used through the rest of the paper will be brought into attention. In the usual concept of regression analysis, we often attempt to explain the variation in one variable by estimating a model consisting of independent or explaining variables. Another useful tool of regression analysis is that it can provide predictions of a dependent variable. In this case, the data are collected from several entities at one point in time (Stock & Watson, 2012). In a time series though, as Stock and Watson (2012) describes it, the data consists of several measurements over time on one or several entities. Time series analysis with all of its applications is a useful asset mainly when examination of macro-data is to be carried out, such as inflation and stock prices. One main condition that has to be fulfilled in order to make good predictions of the future, is that the series has to be stationary (Stock & Watson, 2012). For a thorough introduction of time series analysis, see Greene (2008) or Tsay (2005). There are several tests derived for testing for stationarity in a time series, such as the Dickey Fuller (DF) test, the Augmented DF test and the KPSS test (Greene, 2008). This paper will not consider univariate time series though, but multivariate ditto. In a multivariate time series setting, it is often of interest whether two or more series are cointegrated and of which rank. If two or more nonstationary series are said to be cointegrated then there exists a linear combination of them that is stationary. A few tests derived for this purpose have been suggested through the years such as the Johansens trace test, Johansens max test and the DOLS estimator (Stock & Watson, 2012; Greene, 2008). The Johansen trace test was derived by Johansen (1991) in order to test for cointegration in multivariate time series. This test tests the null hypothesis of at most 푟 cointegration relationships in multivariate time series, against the alternative that there are more than 푟 cointegration relationships. The test statistic derived by Johansen follows a distribution that is a function of standard Brownian motions, and thus has critical values that has to be found via simulation (Tsay, 2005). The critical values used by most statistical software are simulated asymptotical critical values, which in turn may make this test sensitive to situations where small samples are used. Moreover, this test does not seem to be robust when the series are heteroscedastic; or with other words, when the error terms are not white noise (Mantalos, 2001; Cavaliere, Rahbek & Taylor, 2007). The wild bootstrap test has been suggested by as an attempt to solve the problem with the nonrobust Johansen trace test in presence of heteroscedasticity. The first version of the wild bootstrap was suggested by Wu (1986), and refined by Liu (1988), as a suggestion to how to use bootstrapping effectively when the innovation terms in a model are heteroscedastic. They proposed a solution by forcing the residuals to follow a distribution with expectation 0 2 and constant variance. Cavaliere and Taylor (2008) studied the properties of the wild bootstrap test for cointegration, and found it to be robust to heteroscedasticity. Mantalos (2001) also studied the properties of the wild bootstrap cointegration test and got similar results as Cavaliere and Taylor. The methodology of this simulation study
Details
-
File Typepdf
-
Upload Time-
-
Content LanguagesEnglish
-
Upload UserAnonymous/Not logged-in
-
File Pages36 Page
-
File Size-