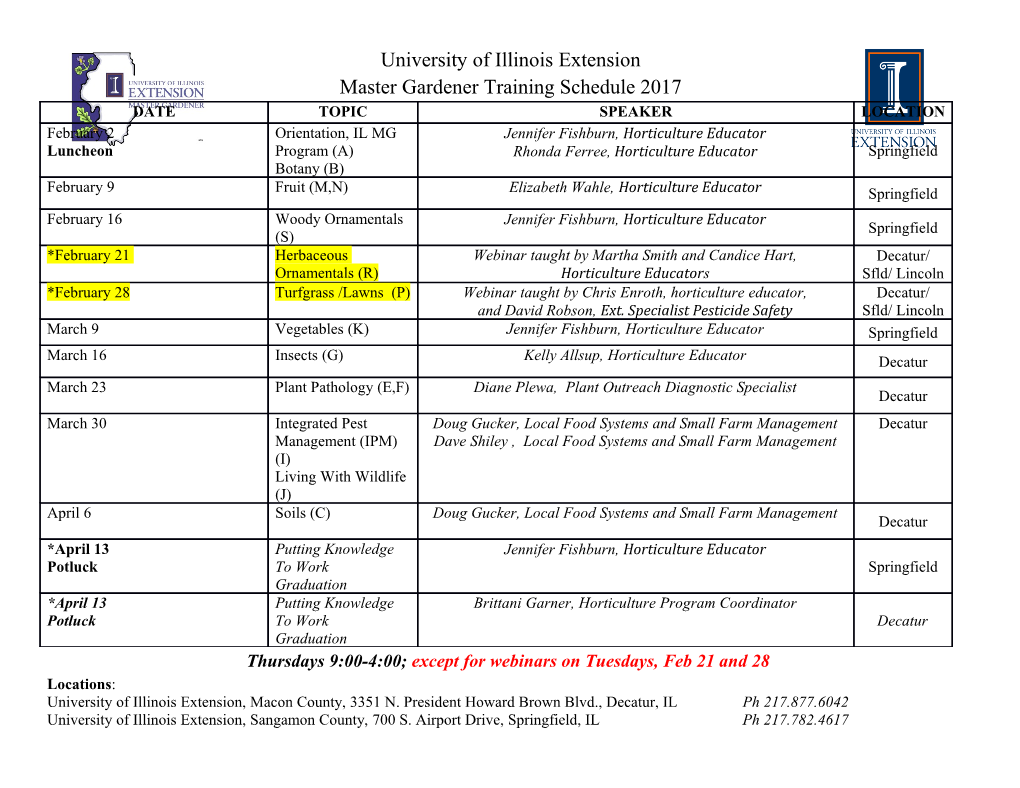
university of copenhagen departmentofeconomics Econometrics II Non-Stationary Time Series and Unit Root Testing Morten Nyboe Tabor university of copenhagen departmentofeconomics Course Outline: Non-Stationary Time Series and Unit Root Testing 1 Stationarity and Deviation from Stationarity Trend-Stationarity Level Shifts and Structural Breaks Variance Changes Unit Roots 2 An Autoregressive Unit Root Process Stationary AR(1) Autoregression with a Unit Root Deterministic Terms Stationary vs. unit root AR(1) 3 Dickey-Fuller Unit Root Testing Test in an AR(1) Test in an AR(p) Test with a Constant Term Test with a Trend Term 4 Empirical Examples 5 Further Issues Econometrics II — Non-Stationary Time Series and Unit Root Testing— Slide 2/35 1. Stationarity and Deviation from Stationarity university of copenhagen departmentofeconomics Stationarity • The main assumption on the time series data so far has been stationarity. Recall the definition: Weak stationarity A time series is called weakly stationary if E(yt ) = µ 2 V (yt ) = E((yt − µ) ) = γ0 Cov(yt , yt−k ) = E((yt − µ)(yt−k − µ)) = γk for k = 1, 2, ... • This can be violated in different ways. Examples of non-stationarity: 1 Deterministic trends (trend stationarity). 2 Level shifts. 3 Variance changes. 4 Unit roots (stochastic trends). Econometrics II — Non-Stationary Time Series and Unit Root Testing— Slide 4/35 university of copenhagen departmentofeconomics Four Non-StationaryFour Non-Stationary Time Series Time Series (A) Stationary and trend-stationary process (B) Process with a level shift 10 yt 5 5 zt 0 0 0 50 100 150 200 0 50 100 150 200 (C) Process with a change in the variance (D) Unit root process 5 10 0 5 0 -5 0 50 100 150 200 0 50 100 150 200 4of28 Econometrics II — Non-Stationary Time Series and Unit Root Testing— Slide 5/35 university of copenhagen departmentofeconomics (A) Trend-Stationarity • Observation: Many macro-economic variables are trending. How should we model the relationship between trending variables? • Assume that zt is stationary and that yt is zt plus a deterministic linear trend, e.g., zt = θzt−1 + t , | θ | < 1, and yt = zt + µ0 + µ1t. • Remarks: 1 yt has a trending mean, E(yt ) = µ0 + µ1t, and is non-stationary. 2 The de-trended variable, zt = yt − µ0 − µ1t, is stationary. yt is trend-stationary. 3 We may analyze the OLS-detrended variable, bzt = yt − µb0 − µb1t. Standard asymptotics apply to regressions with bzt . 4 This is equivalent to extending the regression with a deterministic trend, e.g., yt = β0 + β1 · xt + β3 · t + t . Econometrics II — Non-Stationary Time Series and Unit Root Testing— Slide 6/35 university of copenhagen departmentofeconomics (B) Level Shifts and Structural Breaks • Another type of non-stationarity is due to changes in parameters, e.g., a level shift: µ1 for t = 1, 2, ..., T0 E(yt ) = µ2 for t = T0 + 1, T0 + 2, ..., T . • If each sub-sample is stationary, then there are two modelling approaches: 1 Include a dummy variable n 0 for t = 1, 2, ..., T0 Dt = 1 for t = T0 + 1, T0 + 2, ..., T in the regression model, yt = β0 + β1 · xt + β3 · Dt + t . If yt − β3 · Dt is stationary, standard asymptotics apply. 2 Analyze the two sub-samples separately. This is particularly relevant if we think that more parameters have changed. Econometrics II — Non-Stationary Time Series and Unit Root Testing— Slide 7/35 university of copenhagen departmentofeconomics (C) Variance Changes • A third type of non-stationary is related to changes in the variance. An example is yt = 0.5 · yt−1 + t , where N(0, 1) for t = 1, 2, ..., T0 t ∼ N(0, 5) for t = T0 + 1, T0 + 2, ..., T The interpretation is that the time series covers different regimes. • A natural solution is to model the regimes separately. • Alternatively we can try to model the variance. We return to so-called ARCH models for changing variance later. Econometrics II — Non-Stationary Time Series and Unit Root Testing— Slide 8/35 Socrative Question 1 We simulate M = 10, 000 replications of the AR(1) model, yt = θyt−1 + t , where t ∼ iidN(0, 1) and y0 = 0. We simulate for both θ = 0.5 and θ = 1. For an increasing sample√ size, T = {10, 20, ..., 500}, we consider the distribution of θb and T (θb− θ). Q: What are the properties of θb when θ = 1? (A) It is consistent and asymptotically normal. (B) It is consistent, but not asymptotically normal. (C) It is inconsistent, but asymptotically normal. (D) It is inconsistent and not asymptotically normal. (E) Don’t know. Please go to www.socrative.com, click Student login, and enter room id Econometrics2. university of copenhagen departmentofeconomics (D) Unit Roots • If there is a unit root in(D) an autoregressive Unit Roots model, no standard asymptotics apply! If there is a unit root in an autoregressive model, no standard asymptotics apply! • Consider the DGP Consider the DGP yt = θyt−1 + t , t ∼ N(0, 1), = 1 + (0 1) − ∼ forfort=1= 12,2, ...,500500,,and and0 =0y0 .= Consider 0. Consider the distribution the distribution of . of θb. • Note:Note: the theshape shape, location, locationand andvariance varianceof the of distributions. the distributions. • b (C) Distribution of ^ for =0.5 (D) Distribution of ^ for =1 ^ 10.0 Distribution of Distribution of ^ N(s=0.0389) N(s=0.00588) 100 7.5 5.0 50 2.5 0.0 0.35 0.40 0.45 0.50 0.55 0.60 0.65 0.97 0.98 0.99 1.00 1.01 8of28 Econometrics II — Non-Stationary Time Series and Unit Root Testing— Slide 9/35 2. An Autoregressive Unit Root Process university of copenhagen departmentofeconomics Properties of a Stationary AR(1) • Consider the AR(1) model yt = θyt−1 + t , t = 1, 2, ..., T . The characteristic polynomial is θ(z) = 1 − θz, with characteristic root −1 −1 z1 = θ and inverse root φ1 = z1 = θ. The stationarity condition is that |φ1| = |θ| < 1. • Recall the solution 2 t−1 t yt = t + θt−1 + θ t−2 + ... + θ 1 + θ y0, i where θ → 0 for i → ∞. Shocks have only transitory effects; yt has an attractor (mean reversion). • Note the properties t E(yt ) = θ y0 → 0 σ2 V (y ) = σ2 + θ2σ2 + θ4σ2 + ... + θt−1σ → t 1 − θ2 s ρs = Corr(yt , yt−s ) = θ Econometrics II — Non-Stationary Time Series and Unit Root Testing— Slide 11/35 university of copenhagen departmentofeconomics Simulated Example Simulated Example (A) Shock to a stationary process, = 0.8 (B) Shock to a unit root process, = 1 10 10 5 0 0 0 20 40 60 80 100 0 20 40 60 80 100 (C) ACF for stationary process, =0.8 (D) ACF for unit root process, =1 1.0 1.0 0.5 0.5 0 5 10 15 20 25 0 5 10 15 20 25 10 of 28 Econometrics II — Non-Stationary Time Series and Unit Root Testing— Slide 12/35 Socrative Question 2 Consider the following models for yt : yt = 0.8yt−1 + 0.2yt−2 + t , (1) ∆yt = t , (2) yt = 0.2yt−1 − 1.2yt−2 + t , (3) yt = 0.2yt−1 + 1.2yt−2 − 0.4yt−3 + t , (4) Q: In which of the models (1)-(4) is yt a unit-root process? (A) None of them are unit-root processes. (B) (1) and (2). (C) (1), (2), and (4). (D) They are all unit-root processes. (E) Don’t know. university of copenhagen departmentofeconomics Autoregression with a Unit Root • Consider the AR(1) with θ = 1, i.e., yt = yt−1 + t . The characteristic polynomial is θ(z) = 1 − z. There is a unit root, θ(1) = 0. • The solution is given by: yt = y0 + ∆y1 + ∆y2 + ... + ∆yt−1 + t = y0 + 1 + 2 + ... + t t X = y0 + i . i=1 • Note the remarkable differences between θ = 1 and a stationary process, |θ| < 1 : 1 The effect of the initial value, y0, stays in the process. And E(yt | y0) = y0. 2 Shocks, , have permanent effects. t P Accumulate to a random walk component, i , called a stochastic trend. Econometrics II — Non-Stationary Time Series and Unit Root Testing— Slide 13/35 Socrative Question 3 Consider the AR(1) process with a unit root, yt = yt−1 + t , 2 where t ∼ iid(0, σ ). Q: What is the variance of yt conditional on the initial value? σ2 (A) V (yt |y0) = 1−θ2 2 (B) V (yt |y0) = σ 2 (C) V (yt |y0) = tσ 2 (D) V (yt |y0) = y0 + tσ (E) Don’t know. university of copenhagen departmentofeconomics 3 The variance increases, t X 2 V (yt |y0) = V i |y0 = t · σ . i=1 The process is clearly non-stationary. 4 The covariance, Cov(yt , yt−s |y0), is given by 2 E((yt −y0)(yt−s −y0)|y0) = E((1+2+...+t )(1+2+...+t−s )|y0) = (t−s)σ . The autocorrelation is 2 r Cov(yt , yt−s |y0) (t − s)σ t − s Corr(yt , yt−s |y0) = = = , p p 2 2 V (yt |y0) · V (yt−s |y0) tσ · (t − s)σ t which dies out very slowly with s. 5 The first difference, ∆yt = t , is stationary. yt is called integrated of first order, I(1). Econometrics II — Non-Stationary Time Series and Unit Root Testing— Slide 14/35 Socrative Question 4 Consider the unit root AR(1) process with a constant term and a linear trend, yt = δ + γ · t + yt−1 + t , t = 1, 2, ..., T . Q. What is the effect of the deterministic terms δ and γ · t on yt ? (A) δ determines the level of yt , while γ · t is a linear trend in yt .
Details
-
File Typepdf
-
Upload Time-
-
Content LanguagesEnglish
-
Upload UserAnonymous/Not logged-in
-
File Pages53 Page
-
File Size-