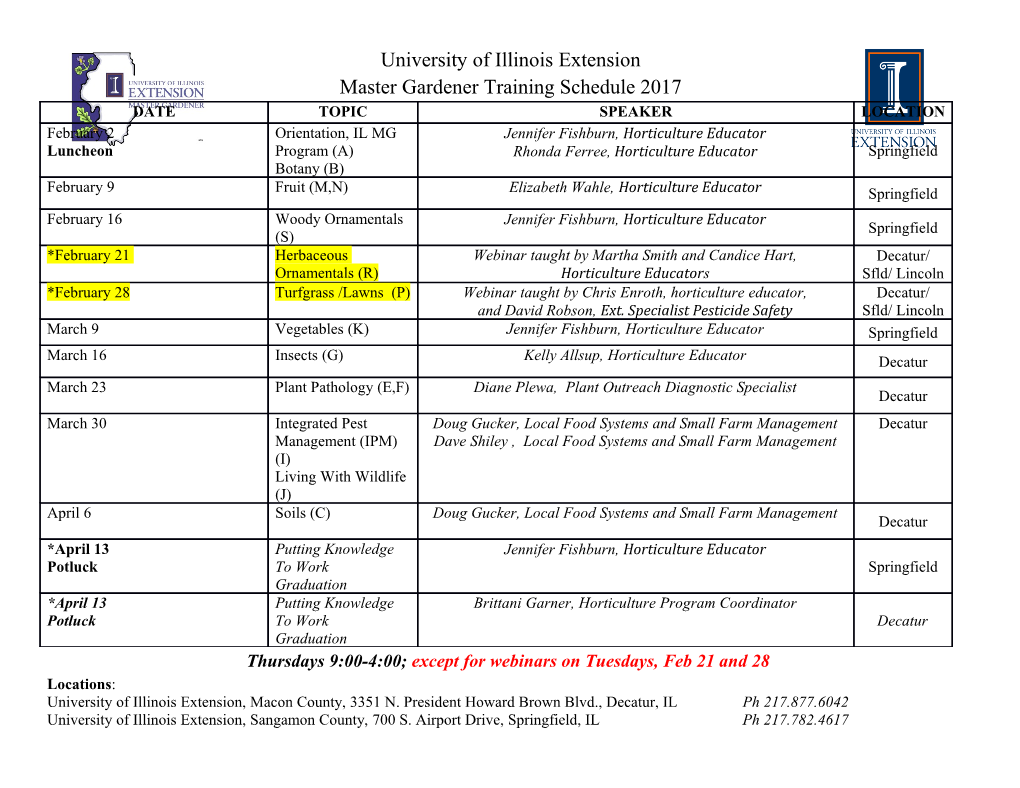
ABSTRACT PRIVACY PRESERVING KIN GENOMIC DATA PUBLISHING by Hui Shang The high availability of genome sequencing data and the advancement in data mining stimulate the progress of biomedical breakthroughs and the hope of personalized medicine. Meanwhile, the popularity of personalized and commercialized genome testing brings about increasing privacy concerns. Differential privacy, derived from cryptography, is a robust framework for sharing aggregate information while protecting an individual’s privacy. However, it is challenging to directly apply the differential privacy methodology for protecting genetic privacy, due to the specific characteristics of genome data: unique, Big Data, and kin-genomic dependency. In this thesis, we construct a genome data model to capture the characteristics of genomic data. We then design an attack algorithm by operating belief propagation on a factor graph. Finally, we develop a differentially private method that contributes to the privacy-preserving dissemination of genomic data. PRIVACY PRESERVING KIN GENOMIC DATA PUBLISHING A Thesis Submitted to the Faculty of Miami University in partial fulfillment of the requirements for the degree of Master of Science by Hui Shang Miami University Oxford, Ohio 2020 Advisor: Dr. He, Zaobo Reader: Dr. Inclezan, Daniela Reader: Dr. Bibak, Khodakhast ©2020 Hui Shang This Thesis titled PRIVACY PRESERVING KIN GENOMIC DATA PUBLISHING by Hui Shang has been approved for publication by The College of Engineering and Computing and The Department of Computer Science & Software Engineering Dr. He, Zaobo Dr. Inclezan, Daniela Dr. Bibak, Khodakhast Table of Contents List of Tables v List of Figures vi Chapter Title vii Chapter Title viii 1 Introduction1 1.1 Motivation . .1 1.2 Contributions and Goals . .2 1.3 Thesis Outline . .2 1.4 Dissemination of Results . .3 2 Background & Related Work4 2.1 Genetic Terms . .4 2.2 Probabilistic Graph Model . .6 2.2.1 Factor Graph . .6 2.2.2 Belief Propagation Algorithm . .7 2.3 Related Work . .8 2.3.1 Inference Attacks . .8 2.3.2 Privacy Preserving Genome Data Sharing Methods . .9 2.4 Comparison . 11 3 Inference Attacks On Kin Genomic Data and Their Mitigation 13 3.1 Introduction . 13 3.2 Preliminaries . 14 3.2.1 Genetics . 14 3.2.2 Differential Privacy . 16 3.2.3 Adversary Model . 17 3.2.4 Problem Definition . 17 3.3 Method . 18 3.3.1 Notations . 19 3.3.2 Construct of Kin Genome Data Model . 19 iii 3.3.3 Applying Belief Propagation . 20 3.4 Sanitization of Kin Genomic Data . 22 3.5 Metrics for Kin Genomic Privacy and Utility . 25 3.5.1 Inference Error . 25 3.5.2 Entropy . 26 3.5.3 Utility . 26 4 Validation 27 4.1 Experimental Design . 27 4.1.1 Experiment Environment . 27 4.1.2 Experimental Data . 27 4.2 Results . 28 4.2.1 Inference Attack . 28 4.2.2 Data Sanitization . 31 5 Conclusion 35 5.1 Summary of This Work . 35 5.2 Challenge . 35 5.3 Future Work . 35 References 37 iv List of Tables 3.1 Probability of a child’s genotypes, given parents’ genotypes . 14 3.2 Probability of a father’s genotypes, given his spouse and his child’s genotypes . 15 3.3 Conditional probability distribution of phenotypes . 16 3.4 Probability of genotypes distribution, given allele frequency . 24 4.1 Inference attack results . 29 v List of Figures 2.1 Example of a factor graph . .6 3.1 Genotype relationships among trio family . 15 3.2 Workflow for kin Genomic data inference attack . 18 3.3 Example of kin genome factor graph, representing a trio with 3 SNP and 2 traits per family member. 19 3.4 Belief propagation on factor graph . 21 3.5 Example of addition of differential privacy noise . 23 4.1 Manuel Corpas Family Tree of the four family members. Females are represented by ellipses and males are represented as squares. 28 4.2 CEPH/UTAH Pedigree 1463 of 11 individuals. Females are represented by ellipses and males are represented as squares. The family tree represents family relationships. 30 4.3 Evaluation of son privacy loss by inference error, given the genome of son’s different relatives . 30 4.4 Evaluation of adversary’s uncertainty for prediction of son, given the genomes of son’s different relatives . 31 4.5 Evaluation of r1 privacy loss by inference error, given the genome of r1’s different relatives . 32 4.6 Evaluation of adversary’s uncertainty for prediction of r1, given the genomes of r1’s different relatives . 33 4.7 Evaluation of DP noise in genetic privacy preservation by inference error . 34 4.8 Evaluation of DP noise in genetic privacy preservation by entropy . 34 vi Dedication To my family, teachers, friends and this beautiful world. vii Acknowledgements It is a challenging but rewarding experience to study Computer Science, a new field that I did not think I would step in a few years ago. It provides me with excellent training and skillsets to broaden my career path. Moreover, it offers me the cherished opportunities to connect many dedicated faculty of the Department of Computer Science and Software Engineering (CSE), and sincere friends who care, support, and encourage me along the way. I would like to offer my special gratitude to Dr. He, Zaobo, my advisor. Thank him for his valuable instructions and professional guidance during the design and progress of this research project. He is very helpful, not only as an advisor but also as a good friend. I would like to express my great appreciation to Dr. Inclezan, Daniela, and Dr. Bibak, Khodakhast, my thesis committee members. They kindly provide for their constructive suggestions and encouragement in my study. They are knowledgeable and professional in both research and teaching. I would also like to thanks other faculties of CSE, Dr. Eric Bachmann, Dr. Md Gani, Dr. Angel Bravo-Salgado, Dr. John Femiani, Dr. Alan Ferrenberg, Dr. Eric Rapos, Dr. Chun Liang, Dr. Karen Davis and Dr. Mike Zmuda for their excellent lectures. I am grateful to my friends, classmates, and those who kindly offer assistance and encourage- ment. Thank Liu Xian and Xiaolin Liu for encouraging me to step out of my comfort zone and beginning my journey in the field of Computer Science. Thank Chitraketu Pandey for helping me implement the Belief Propagation Algorithm in Python for this research work and the collabora- tions for our team course projects. I enjoyed the time we discussed various coding questions and algorithms. Thank Iman Deznabi for providing some crucial datasets for experimenting. Thank Yefei Ye for helping me in the data preprocessing. Thank Shrawani Silwa, Zehua Lin, Zunchen Zhao, Minghua Li, Li Zhang, Yanxue Xie, Janelle Allen, and Shangye Chen for their support. I would like to give my special thanks to my family. My parents gave me life, and raised me, taught me to be kind and helpful towards others. They also teach me to be persistent and keep optimistic about life. I want to thank my husband, who accompanies me and supports me all the time. He spares no effort to make me happy. I am also grateful to my parents in law who care for us. I feel blessed to have connected with many lovely people. At a hard time of the COVID-19 pandemic, I hope everyone is fine and safe. "Love and Honor" by the code of Miami University. viii Chapter 1 Introduction 1.1 Motivation The development of genome sequencing technologies and the fast dropping price of sequencing has accelerated the rapid accumulation of genetic data. For example, the cost of sequencing a human genome decreased significantly from over $ 1 million in 2007 to around $ 1 thousand in 2015 [1]. Meanwhile, a tremendous amount of human genome data are generated and stored. For instance, in 2017, the European Genome-phenome Archive (AGA) stored 5.85 petabytes (PB) of human genomic data at a 29:5% yearly increase in the total storage size [2]. Nowadays, researchers across the world have access to more than terabytes of genetic data through the websites supported by academic and research organizations such as the European Bioinformatics Institute (EBI) and the US National Center for Biotechnology Information (NCBI) [3]. Moreover, commercial genome service platforms such as 23andMe [4], OpenSNP [5], and PatientsLikeMe [6] allow individuals/customers to obtain their personal genetic/health information, and release their data. Publishing and sharing these human genomic data and genetic discovery are essential for better health. With the perspective of researchers, the availability of human genome sequences stimulates the progress of team-oriented interdisciplinary projects and biomedical breakthroughs for human evolution, disease diagnostics, and therapies [7]. Big Data analytic allows researchers to reveal the associations between genetic information and disease. For individuals, the genome data help them to learn their risk of diseases, their ancestry, and receive personalized medicine for better treatment [8]. One major issue with genomic data publishing is that the release of a person’s genetic in- formation constitutes a threat to their privacy. For example, an attacker is capable of gaining sensitive information by computing an individual’s disease susceptibility from single nucleotide polymorphisms (SNPs) in a published genetic database such as Genome-wide Association Studies (GWASs) Catalog [9]. In this way, the privacy of participants and even their family members will be adversely affected when their sensitive information is exposed. The leakage of personal sensitive genetic information could bring harm to many aspects of their lives, such as financial situation, social status, and family relationships. For example, they may face discrimination from health insurance or employer if their genomic data are revealing their higher risks of certain diseases. Moreover, with the increasing concern of privacy and the risk of genetic information breaches, people will be unlikely to share their genetic data or participate into biomedical research such as GWAS.
Details
-
File Typepdf
-
Upload Time-
-
Content LanguagesEnglish
-
Upload UserAnonymous/Not logged-in
-
File Pages51 Page
-
File Size-