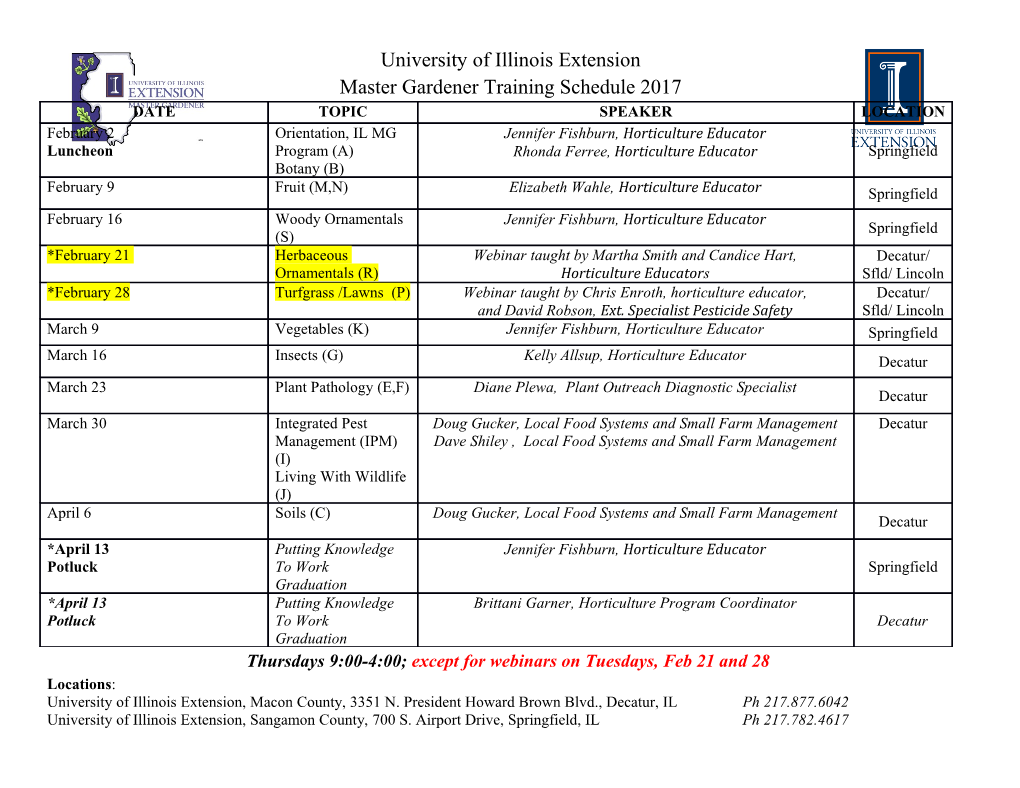
Open Geosci. 2020; 12:44–58 Research Article Liming Xiao, Yonghong Zhang*, Taotao Ge, Chen Wang, and Ming Wei Analysis, Assessment and Early Warning of Mudflow Disasters along the Shigatse Section of the China–Nepal Highway https://doi.org/10.1515/geo-2020-0004 Keywords: Shigatse section of the China–Nepal Highway, Received Jan 07, 2019; accepted Nov 22, 2019 mudflow, disaster analysis, risk assessment, early warning model Abstract: China–Nepal Highway is an important interna- tional passage connecting China and Nepal. Owing to its location in a complex mountainous area in the Qinghai– Tibet Plateau, the Shigatse section of the China–Nepal 1 Introduction Highway is often impacted and troubled by mudflow. In order to effectively conduct road construction and mainte- 1.1 Introduction to the Shigatse section of nance and improve early disaster-warning capability, the the China–Nepal Highway relationship between various hazard factors and disaster points was analysed. It is found that four factors such as China–Nepal Highway, located in the central part of the slope, precipitation, soil type and digital elevation have Hindu Kush–Himalayan region, begins in the east in the strongest correlation with the occurrence of the dis- Lhasa, which is the capital city of the Tibet Autonomous asters. From the distribution of disaster points, it is ob- Region of the People’s Republic of China; it ends towards served that the disaster point is closely related to the slope, the west in Kathmandu, which is the capital of the Federal its local correlation with precipitation is good and the its Democratic Republic of Nepal. It is 943 km long and the local correlation with the soil type and Digital Elevation most important land-based connecting passage between Model (DEM) data is significant. In order to quantitatively China and Nepal. It is the “golden corridor” of tourism in evaluate the susceptibility of mudflow disasters in the Shi- the Tibet Autonomous Region and an important part of gatse region, this paper uses the analytic hierarchy process China’s “One Belt, One Road” initiative, as shown in Fig- (AHP) as the main analysis method supplemented by the ure 1. According to the statistics of the Ministry of Com- fuzzy clustering method. The results show that the slope, merce of the People’s Republic of China, the bilateral trade when accompanied by heavy rainfall, is the most impor- volume between China and Nepal reached 993 million USD tant factor among four factors. In this paper, the neural in 2017,a yearly increase of 11.2% over the last year. China– network method is used to establish the identification and Nepal Highway has played a very important role as the early warning model of mudflow susceptibility. When the main connecting road between the two countries. In 2017, recognition rate reaches 66% or above, it can be used as an bilateral trade between China and Nepal reached US$993 early-warning threshold for mudflow disasters. This study million. has conducted a useful exploration of the research, assess- The Shigatse region is the bridgehead from China to ment and early warning of mudflow disasters along the Nepal, additionally, it is a region susceptible to mudflow. Shigatse section of the China–Nepal Highway. The frequent mudflow disasters in the Shigatse section have hindered the smooth traffic of the China-Nepal High- way. Therefore, it is urgent and pragmatic to study the iden- *Corresponding Author: Yonghong Zhang: School of Automation, tification and early warning of mudflow disasters inthe Nanjing University of Information Science and Technology, 210044 plateau and mountains. Nanjing, China; Email: [email protected] Liming Xiao, Taotao Ge: School of Automation, Nanjing University of Information Science and Technology, 210044 Nanjing, China Chen Wang, Ming Wei: Collaborative Innovation Center on Fore- cast and Evaluation of Meteorological Disasters, Nanjing University of Information Science and Technology, 210044 Nanjing, China Open Access. © 2020 L. Xiao et al., published by De Gruyter. This work is licensed under the Creative Commons Attribution 4.0 License Analysis, Assessment and Early Warning of Mudflow Disasters along the Shigatse Section Ë 45 Figure 1: Location map of China–Nepal Highway (the red line in the picture represents the China–Nepal Highway) 1.2 Research progress area as training points instead of using a point to repre- sent the mudflow catchment; then, geomorphology, lithol- Nguyen combined remote sensing and GIS (Geographic In- ogy, faults, earthquakes and river system were selected to formation System) technology with the AHP to determin- serve as evidence for analysis. The optimal buffer cutoff ing the weights of various environmental factors in trigger- distance of faults and rivers is determined by the maxi- ing debris flow and to map the ecotone [1]. Xu established mum study value of contrast ratio; finally, based on the an information value model to calculate a total of seven im- subject of evidence, the mudflow susceptibility map in pact factors: elevation, slope, aspect, flow accumulation, Kangding County is obtained [4]. Wang used SPOT5 im- vegetation coverage, soil type and land use. The compre- ages, DEM, the lithology distribution map and rainfall data hensive information value was analysed using GIS tech- to identify the triggering factors for mudflow susceptibil- nology to determine the mudflow susceptibility [2]. Zhang ity classification. Principal Component Analysis and Self- selected the following seven major factors: loose material organising Maps methods were used to analyse the trig- volume per square kilometre, loose material supply length gering factors such as basin relief ratio, slope gradient in ratio, average gradient of the main channel, average hill the initiation zone, drainage density, downslope curvature slope, drainage density, curvature of the main channel and of the main channel, vegetation coverage, main channel poor vegetation area ratio and then analysed their impacts aspect, topographic wetness index(TWI), Meltons rugged- on the susceptibility of mudflow. The researchers used the ness number, lithology, annual rainfall, form factor, and combination weighting method, AHP and entropy weight cross-slope curvature of the transportation zone. The 14 method to select major factors causing mud-rock flow [3]. triggering factors were analysed to evaluate the mudflow Chen modified the method of determining training points disasters, and the results were further verified [5]. Truong by setting the cells covered by the mudflow catchment proposed a new machine learning integration method, i.e., 46 Ë L. Xiao et al. a hybrid method of Bagging Ensemble (BE) and Logistic and compiled the following ten mudflow conditioning fac- Model Tree (LMtree), called BE-LMtree. The following eight tors to determine the relationship between mudflow con- triggering factors were extracted: slope, aspect, elevation, ditioning factors and mudflow inventory map: land use, land cover, soil type, lithology, distance to faults and dis- distance to drainage, slope, elevation, lithology, distance tance to river; 255 landslides were trained and verified. The to roads, distance to faults, slope orientation, TWI, and performance of the new method is better than the support stream power index. Among these mudflow conditioning vector machine and LMtree models [6]. Chang numerically factors, land use was found to be an important factor af- simulated the mudflow intensity, velocity and maximum fecting mudflow [12] Ba compared the slope unit and grid depth using the FLO-2D numerical analysis software and cell as the mapping unit for mudflow susceptibility as- combined the regression cycles of 20, 100 and 200 years sessment. Using the improved information value model, to classify the mudflow hazard levels in the study area [7]. the mudflow susceptibility maps based on slope units and Aditian selected the following eight mudflow susceptibil- grid cells were obtained. Receiver Operating Characteristic ity factors in Ambon to verify and compare the accuracy curve was used to evaluate the results, and mudflow sus- of the mudflow susceptibility models based on bivariate ceptibility mapping based on slope units was found to per- frequency ratio, multivariate logistic regression and artifi- form better than the grid cell-based method [13]. Tekin se- cial neural network: elevation, slope, aspect, distance to lected 78%–83% for the training set and 17%–22% for the river network, lithology, density of geological boundaries, validation set and used the LR model to model the mud- distance to faults and distance to road network. Among flow susceptibility, including factors such as geology, land- these susceptibility factors, Neural network model has ad- form classification, land use, elevation, slope, plane curva- vantages in explaining the relationship between debris ture, profile curvature, slope length factor, solar radiation, flow and other factors [8]. Othman extracted 16 geomor- stream power index, slope second derivate, TWI, heat load phological factors mainly from the Digital Elevation Model index, mean slope, slope position, roughness, dissection, (DEM) and evaluated and compared the frequency ratio, surface relief ratio, linear aspect and slope/aspect ratio. weight of evidence, logistic regression and probability re- The results show that the susceptibility map generated us- gression methods combined with geomorphological fac- ing the random selections considering the entire mudflow tors to determine mudflow susceptibility.
Details
-
File Typepdf
-
Upload Time-
-
Content LanguagesEnglish
-
Upload UserAnonymous/Not logged-in
-
File Pages15 Page
-
File Size-