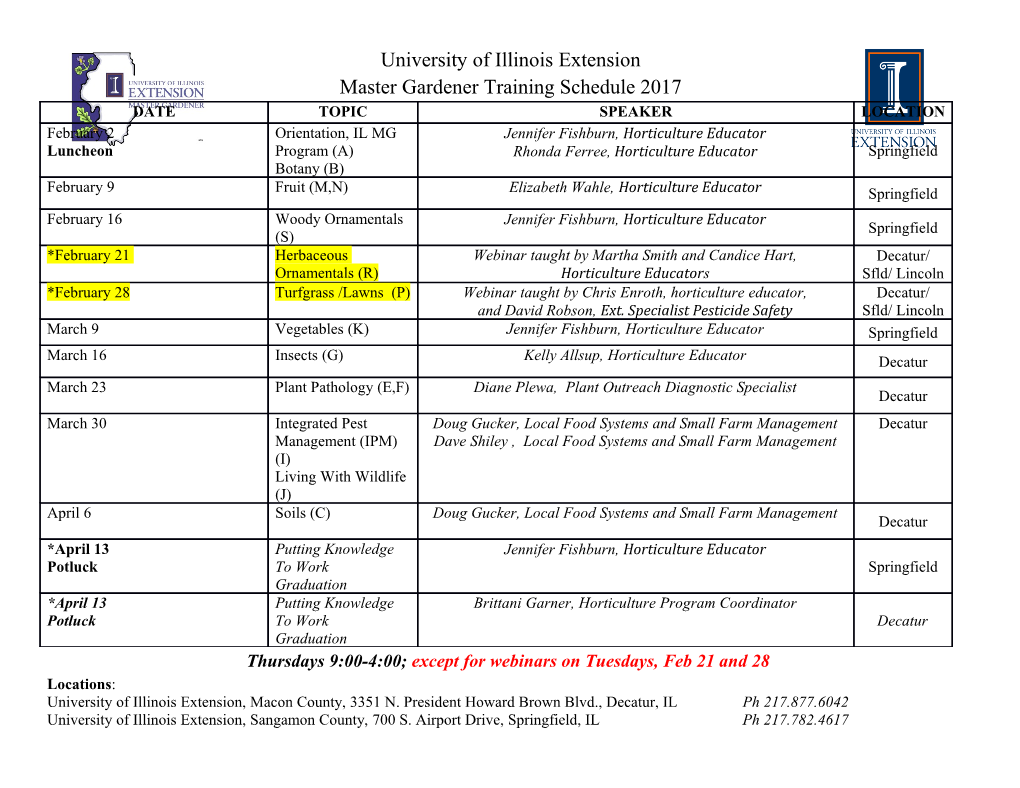
CS229T/STAT231: Statistical Learning Theory (Winter 2016) Percy Liang Last updated Wed Apr 20 2016 01:36 These lecture notes will be updated periodically as the course goes on. The Appendix describes the basic notation, definitions, and theorems. Contents 1 Overview4 1.1 What is this course about? (Lecture 1).....................4 1.2 Asymptotics (Lecture 1).............................5 1.3 Uniform convergence (Lecture 1)........................6 1.4 Kernel methods (Lecture 1)...........................8 1.5 Online learning (Lecture 1)............................9 2 Asymptotics 10 2.1 Overview (Lecture 1)............................... 10 2.2 Gaussian mean estimation (Lecture 1)..................... 11 2.3 Multinomial estimation (Lecture 1)....................... 13 2.4 Exponential families (Lecture 2)......................... 16 2.5 Maximum entropy principle (Lecture 2)..................... 19 2.6 Method of moments for latent-variable models (Lecture 3).......... 23 2.7 Fixed design linear regression (Lecture 3).................... 30 2.8 General loss functions and random design (Lecture 4)............. 33 2.9 Regularized fixed design linear regression (Lecture 4)............. 40 2.10 Summary (Lecture 4)............................... 44 2.11 References..................................... 45 3 Uniform convergence 46 3.1 Overview (Lecture 5)............................... 47 3.2 Formal setup (Lecture 5)............................. 47 3.3 Realizable finite hypothesis classes (Lecture 5)................. 50 3.4 Generalization bounds via uniform convergence (Lecture 5).......... 53 3.5 Concentration inequalities (Lecture 5)...................... 56 3.6 Finite hypothesis classes (Lecture 6)...................... 62 3.7 Concentration inequalities (continued) (Lecture 6)............... 63 3.8 Rademacher complexity (Lecture 6)....................... 66 3.9 Finite hypothesis classes (Lecture 7)...................... 72 3.10 Shattering coefficient (Lecture 7)........................ 74 1 3.11 VC dimension (Lecture 7)............................ 76 3.12 Norm-constrained hypothesis classes (Lecture 7)................ 81 3.13 Covering numbers (metric entropy) (Lecture 8)................ 88 3.14 Algorithmic stability (Lecture 9)......................... 96 3.15 PAC-Bayesian bounds (Lecture 9)........................ 100 3.16 Interpretation of bounds (Lecture 9)...................... 104 3.17 Summary (Lecture 9)............................... 105 3.18 References..................................... 107 4 Kernel methods 108 4.1 Motivation (Lecture 10)............................. 109 4.2 Kernels: definition and examples (Lecture 10)................. 111 4.3 Three views of kernel methods (Lecture 10).................. 114 4.4 Reproducing kernel Hilbert spaces (RKHS) (Lecture 10)........... 116 4.5 Learning using kernels (Lecture 11)....................... 121 4.6 Fourier properties of shift-invariant kernels (Lecture 11)............ 125 4.7 Efficient computation (Lecture 12)....................... 131 4.8 Universality (skipped in class).......................... 138 4.9 RKHS embedding of probability distributions (skipped in class)....... 139 4.10 Summary (Lecture 12).............................. 142 4.11 References..................................... 142 5 Online learning 143 5.1 Introduction (Lecture 13)............................ 143 5.2 Warm-up (Lecture 13).............................. 146 5.3 Online convex optimization (Lecture 13).................... 147 5.4 Follow the leader (FTL) (Lecture 13)...................... 151 5.5 Follow the regularized leader (FTRL) (Lecture 14)............... 155 5.6 Online subgradient descent (OGD) (Lecture 14)................ 158 5.7 Online mirror descent (OMD) (Lecture 14)................... 161 5.8 Regret bounds with Bregman divergences (Lecture 15)............ 165 5.9 Strong convexity and smoothness (Lecture 15)................. 167 5.10 Local norms (Lecture 15)............................. 171 5.11 Adaptive optimistic mirror descent (Lecture 16)................ 174 5.12 Online-to-batch conversion (Lecture 16)..................... 180 5.13 Adversarial bandits: expert advice (Lecture 16)................ 183 5.14 Adversarial bandits: online gradient descent (Lecture 16)........... 185 5.15 Stochastic bandits: upper confidence bound (UCB) (Lecture 16)....... 188 5.16 Stochastic bandits: Thompson sampling (Lecture 16)............. 191 5.17 Summary (Lecture 16).............................. 192 5.18 References..................................... 193 2 6 Neural networks (skipped in class) 194 6.1 Motivation (Lecture 16)............................. 194 6.2 Setup (Lecture 16)................................ 195 6.3 Approximation error (universality) (Lecture 16)................ 196 6.4 Generalization bounds (Lecture 16)....................... 198 6.5 Approximation error for polynomials (Lecture 16)............... 200 6.6 References..................................... 203 7 Conclusions and outlook 204 7.1 Review (Lecture 18)............................... 204 7.2 Changes at test time (Lecture 18)........................ 206 7.3 Alternative forms of supervision (Lecture 18).................. 208 7.4 Interaction between computation and statistics (Lecture 18)......... 209 A Appendix 211 A.1 Notation..................................... 211 A.2 Linear algebra................................... 212 A.3 Probability.................................... 213 A.4 Functional analysis................................ 215 3 [begin lecture 1] (1) 1 Overview 1.1 What is this course about? (Lecture 1) • Machine learning has become an indispensible part of many application areas, in both science (biology, neuroscience, psychology, astronomy, etc.) and engineering (natural language processing, computer vision, robotics, etc.). But machine learning is not a single approach; rather, it consists of a dazzling array of seemingly disparate frame- works and paradigms spanning classification, regression, clustering, matrix factoriza- tion, Bayesian networks, Markov random fields, etc. This course aims to uncover the common statistical principles underlying this diverse array of techniques. • This class is about the theoretical analysis of learning algorithms. Many of the analysis techniques introduced in this class|which involve a beautiful blend of probability, linear algebra, and optimization|are worth studying in their own right and are useful outside machine learning. For example, we will provide generic tools to bound the supremum of stochastic processes. We will show how to optimize an arbitrary sequence of convex functions and do as well on average compared to an expert that sees all the functions in advance. • Meanwhile, the practitioner of machine learning is hunkered down trying to get things to work. Suppose we want to build a classifier to predict the topic of a document (e.g., sports, politics, technology, etc.). We train a logistic regression with bag-of-words features and obtain 8% training error on 1000 training documents, test error is 13% on 1000 documents. There are many questions we could ask that could help us move forward. { How reliable are these numbers? If we reshuffled the data, would we get the same answer? { How much should we expect the test error to change if we double the number of examples? { What if we double the number of features? What if our features or parameters are sparse? { What if we double the regularization? Maybe use L1 regularization? { Should we change the model and use an SVM with a polynomial kernel or a neural network? In this class, we develop tools to tackle some of these questions. Our goal isn't to give precise quantitative answers (just like analyses of algorithms doesn't tell you how 4 exactly many hours a particular algorithm will run). Rather, the analyses will reveal the relevant quantities (e.g., dimension, regularization strength, number of training examples), and reveal how they influence the final test error. • While a deeper theoretical understanding can offer a new perspective and can aid in troubleshooting existing algorithms, it can also suggest new algorithms which might have been non-obvious without the conceptual scaffolding that theory provides. { A famous example is boosting. The following question was posed by Kearns and Valiant in the late 1980s: is it possible to combine weak classifiers (that get 51% accuracy) into a strong classifier (that get 99% accuracy)? This theoretical challenge eventually led to the development of AdaBoost in the mid 1990s, a simple and practical algorithm with strong theoretical guarantees. { In a more recent example, Google's latest 22-layer convolutional neural network that won the 2014 ImageNet Visual Recognition Challenge was initially inspired by a theoretically-motivated algorithm for learning deep neural networks with sparsity structure. There is obviously a large gap between theory and practice; theory relies on assump- tions can be simultaneously too strong (e.g., data are i.i.d.) and too weak (e.g., any distribution). The philosophy of this class is that the the purpose of theory here not to churn out formulas that you simply plug numbers into. Rather, theory should change the way you think. • This class is structured into four sections: asymptotics, uniform convergence, kernel methods, and online learning. We will move from very strong assumptions (assuming the data are Gaussian, in asymptotics) to very weak assumptions (assuming the data can be generated by an adversary, in online learning). Kernel methods is a bit of an outlier
Details
-
File Typepdf
-
Upload Time-
-
Content LanguagesEnglish
-
Upload UserAnonymous/Not logged-in
-
File Pages217 Page
-
File Size-