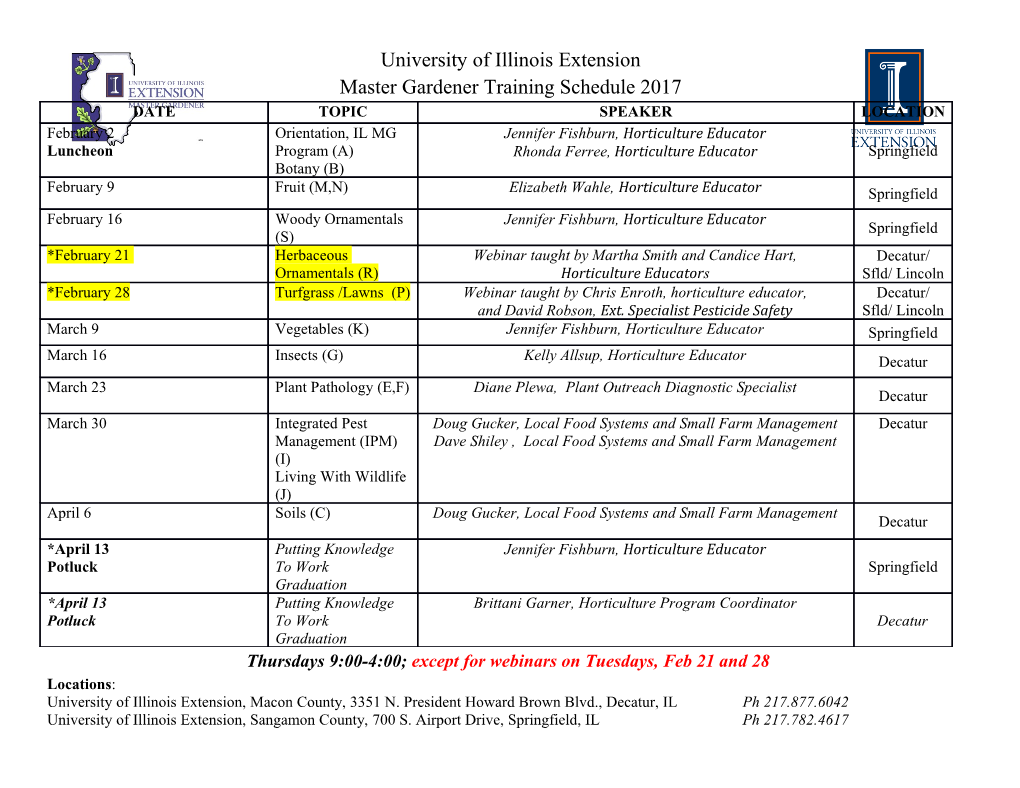
Real and complex inner products We discuss inner products on finite dimensional real and complex vector spaces. Although we are mainly interested in complex vector spaces, we begin with the more familiar case of the usual inner product. 1 Real inner products n Let v = (v1; : : : ; vn) and w = (w1; : : : ; wn) 2 R . We define the inner product (or dot product or scalar product) of v and w by the following formula: hv; wi = v1w1 + ··· + vnwn: Define the length or norm of v by the formula q p 2 2 kvk = hv; vi = v1 + ··· + vn: Note that we can define hv; wi for the vector space kn, where k is any field, but kvk only makes sense for k = R. We have the following properties for the inner product: n 1. (Bilinearity) For all v, u, w 2 R , hv + u; wi = hv; wi + hu; wi and n hv; u + wi = hv; ui + hv; wi. For all v, w 2 R and t 2 R, htv; wi = hv; twi = thv; wi. n 2. (Symmetry) For all v, w 2 R , hv; wi = hw; vi. n 2 3. (Positive definiteness) For all v 2 R , hv; vi = kvk ≥ 0, and hv; vi = 0 if and only if v = 0. The inner product and norm satisfy the familiar inequalities: n 1. (Cauchy-Schwarz) For all v; w 2 R , jhv; wij ≤ kvkkwk, with equality () v and w are linearly dependent. 1 n 2. (Triangle) For all v; w 2 R , jv + wk ≤ kvk + kwk, with equality () v is a positive scalar multiple of w or vice versa. n 3. For all v; w 2 R , jkvk − kwkj ≤ kv − wk. Recall that the standard basis e1; : : : ; en is orthonormal: ( 1; if i = j; hei; eji = δij = 0; if i 6= j. n More generally, vectors u1; : : : ; un 2 R are orthonormal if, for all i; j, 2 hui; uji = δij, i.e. hui; uii = kuik = 1, and hui; uji = 0 for i 6= j. In this case, u1; : : : ; un are linearly independent and hence automatically a ba- n sis of R . One advantage of working with an orthonormal basis u1; : : : ; un is that, for an arbitrary vector v, it is easy to read off the coefficients of Pn v with respect to the basis u1; : : : ; un, i.e. if v = i=1 tiui is written as a linear combination of the ui, then clearly n X hv; uii = tjhuj; uii = ti: j=1 n Equivalently, for all v 2 R , n X v = hv; uiiui: i=1 We have the following: n Proposition 1.1 (Gram-Schmidt). Let v1; : : : ; vn be a basis of R . Then n there exists an orthonormal basis u1; : : : ; un of R such that, for all i, 1 ≤ i ≤ n, spanfv1; : : : ; vig = spanfu1; : : : ; uig: n In particular, for every subspace W of R , there exists an orthonormal basis n u1; : : : ; un of R such that u1; : : : ; ua is a basis of W . Proof. Given the basis v1; : : : ; vn, we define the ui inductively as follows. Since v 6= 0, kv k 6= 0. Set u = 1 v , a unit vector (i.e. ku k = 1). Now 1 1 1 kv1k 1 1 suppose inductively that we have found u1; : : : ; ui such that, for all k; ` ≤ i, huk; u`i = δk`, and such that spanfv1; : : : ; vig = spanfu1; : : : ; uig. Define 0 Pi vi+1 = vi+1 − j=1hvi+1; ujiuj. Clearly 0 spanfv1; : : : ; vi+1g = spanfu1; : : : ; ui; vi+1g: 2 0 Thus, vi+1 6= 0, since otherwise dim spanfv1; : : : ; vi+1g would be less than i. Also, for k ≤ i, i 0 X hvi+1; uki = hvi+1; uki − hvi+1; ujihuj; uki = hvi+1; uki − hvi+1; uki = 0: j=1 1 0 Set ui+1 = 0 vi+1. Then ui+1 is a unit vector and (since ui+1 is a scalar kvi+1k 0 multiple of vi+1) hui+1; uki = 0 for all k ≤ i. This completes the inductive definition of the basis u1; : : : ; un, which has the desired properties. The final n statement is then clear, by starting with a basis v1; : : : ; vn of R such that v1; : : : ; va is a basis of W . The construction of the proof above leads to the construction of orthog- n onal projections. If W is a subspace of R , then there are many different 0 n 0 complements to W , i.e. subspaces W such that R is the direct sum W ⊕W . Given the inner product, there is a natural choice: n Definition 1.2. Let X ⊆ R . Then ? n X = fv 2 R : hv; xi = 0 for all x 2 Xg: ? n It is easy to see from the definitions that X is a subspace of R and ? ? n that X = W , where W is the smallest subspace of R containing X, which we can take to be the set of all linear combinations of elements of X. In particular, if W = spanfw1; : : : ; wag, then ? n W = fv 2 R : hv; wii = 0; 1 ≤ i ≤ ag: n n Proposition 1.3. If W is a vector subspace of R , then R is the direct ? n ? sum of W and W . In this case, the projection p: R ! W with kernel W is called the orthogonal projection onto W . Proof. We begin by giving a formula for the orthogonal projection. Let n u1; : : : ; un be an an orthonormal basis of R such that u1; : : : ; ua is a basis of W , and define a X pW (v) = hv; uiiui: i=1 Clearly Im pW ⊆ W . Moreover, if w 2 W , then there exist ti 2 R with Pa w = i=1 tiui, and in fact ti = hw; uii. Thus, for all w 2 W , a X w = hw; uiiui = pW (w): i=1 ? Finally, v 2 Ker pW () hv; uii = 0 for 1 ≤ i ≤ a () v 2 W . It follows n ? that R = W ⊕ W and that pW is the corresponding projection. 3 2 Symmetric and orthogonal matrices Let A be an m × n matrix with real coefficients, corresponding to a linear n m map R ! R which we will also denote by A. If A = (aij), we define the t t transpose A to be the n × m matrix (aji); in case A is a square matrix, A is the reflection of A about the diagonal going from upper left to lower right. Since tA is an n × m matrix, it corresponds to a linear map (also denoted t m n Pm by A) from R to R . Since a(ei) = j=1 ajiej, it is easy to see that one n m has the formula: for all v 2 R and w 2 R , hAv; wi = hv; tAwi; m where the first inner product is of two vectors in R and the second is of two n vectors in R . In fact, using bilinearity of the inner product, it is enough to t check that hAei; eji = hei; Aeji for 1 ≤ i ≤ n and 1 ≤ j ≤ m, which follows immediately. From this formula, or directly, it is easy to check that t(BA) = tAtB whenever the product is defined. In other words, taking transpose reverses the order of multiplication. Finally, we leave it as an exercise to check that, if m = n and A is invertible, then so is tA, and in fact (tA)−1 = t(A−1): Note that all of the above formulas make sense when we replace R by an arbitrary field k. If A is a square matrix, then tA is also a square matrix, and we can compare A and tA. Definition 2.1. Let A 2 Mn(R), or more generally let A 2 Mn(k). Then t n A is symmetric if A = A. Equivalently, for all v; w 2 R , hAv; wi = hv; Awi: Definition 2.2. Let A 2 Mn(R). Then A is an orthogonal matrix if, for n all v; w 2 R , hAv; Awi = hv; wi. In other words, A preserves the inner product. Lemma 2.3. Let A 2 Mn(R). Then the following are equivalent: n (i) A is orthogonal, i.e. for all v; w 2 R , hAv; Awi = hv; wi. n (ii) For all v 2 R , kAvk = kvk, i.e. A preserves length. 4 (iii) A is invertible, and A−1 = tA. n (iv) The columns of A are an orthonormal basis of R . n (v) The rows of A are an orthonormal basis of R . Proof. (i) =) (ii): Clear, since we can take w = v. (ii) =) (i): Follows n from the polarization identity: For all v; w 2 R , 2hv; wi = kv + wk2 − kvk2 − kwk2: n (i) =) (iii): Suppose that, for all v; w 2 R , hAv; Awi = hv; wi. Now t n t hAv; Awi = hv; AAwi, and hence, for all w 2 R , hv; wi = hv; AAwi for all n v 2 R . It follows that hv; w − tAAwi = 0: n t n In other words, for every w 2 R , w − AAw is orthogonal to every v 2 R , hence w − tAAw = 0, w = tAAw, and so tAA = Id. Thus A−1 = tA. −1 t n (iii) =) (i): If A = A, then, for all v; w 2 R , hAv; Awi = hv; tAAwi = hv; A−1Awi = hv; wi: t (iii) () (iv): In general, the entries of AA are the inner products hci; cji, where c1; : : : ; cn are the columns of A. Thus, the columns of A are an n t −1 t orthonormal basis of R () AA = Id () A = A. (iii) () (v): t Similar, using the fact that the entries of A A are the inner products hri; rji, where r1; : : : ; rn are the rows of A.
Details
-
File Typepdf
-
Upload Time-
-
Content LanguagesEnglish
-
Upload UserAnonymous/Not logged-in
-
File Pages12 Page
-
File Size-