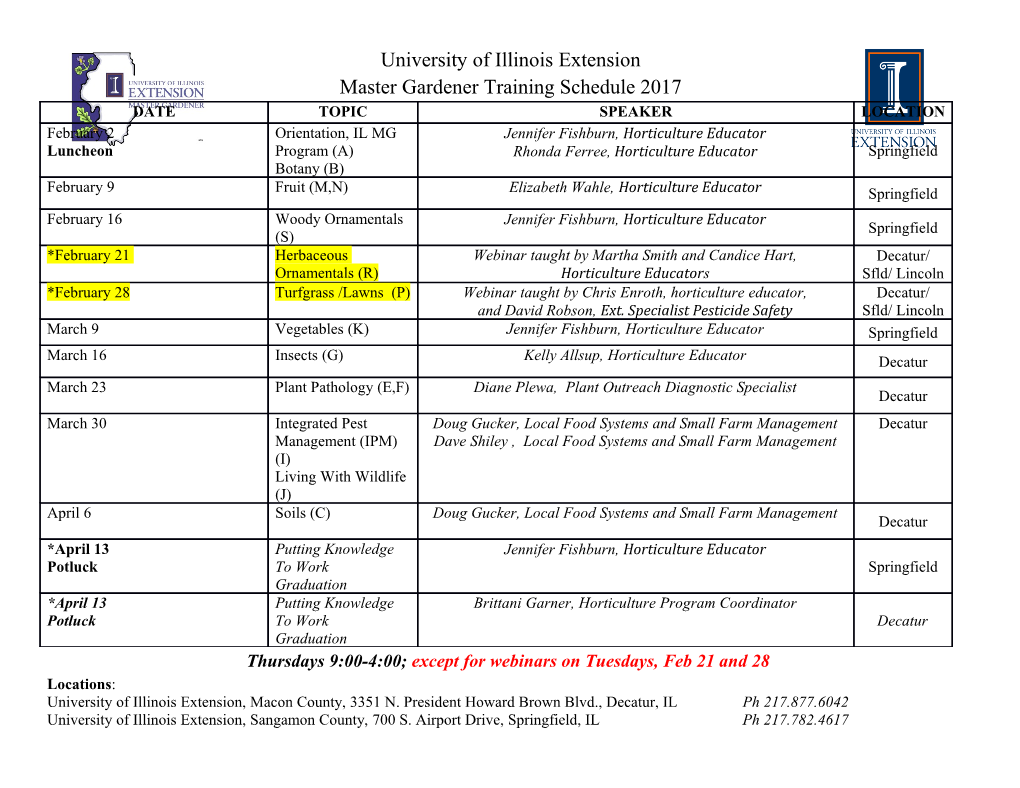
Clark University Clark Digital Commons International Development, Community and Master’s Papers Environment (IDCE) 4-2019 An Evaluation of Sentinel-1 and Sentinel-2 for Land Cover Classification Aaron Meneghini Clark University, [email protected] Follow this and additional works at: https://commons.clarku.edu/idce_masters_papers Part of the Geographic Information Sciences Commons, Physical and Environmental Geography Commons, and the Remote Sensing Commons Recommended Citation Meneghini, Aaron, "An Evaluation of Sentinel-1 and Sentinel-2 for Land Cover Classification" (2019). International Development, Community and Environment (IDCE). 235. https://commons.clarku.edu/idce_masters_papers/235 This Research Paper is brought to you for free and open access by the Master’s Papers at Clark Digital Commons. It has been accepted for inclusion in International Development, Community and Environment (IDCE) by an authorized administrator of Clark Digital Commons. For more information, please contact [email protected], [email protected]. An Evaluation of Sentinel-1 and Sentinel-2 for Land Cover Classification. Author: Aaron Meneghini1 1Department of International Development, Community, and Environment. Clark University 950 Main Street, Worcester, MA. 01610. United States of America. Date of Degree Conferment: May, 2019 A Master’s Paper Submitted to the faculty of Clark University, Worcester, Massachusetts, in partial fulfillment of the requirements for the degree of Master of Sciences in the department of International Development, Community, and the Environment. And accepted on the recommendation of Dr. Florencia Sangermano, Chief Instructor Signature: _____________________________ i Abstract: An Evaluation of Sentinel-1 and Sentinel-2 for Land Cover Classification. Aaron Meneghini This study evaluates Sentinel-1 and Sentinel-2 remotely sensed images for tropical land cover classification. The dual polarized Sentinel-1 VV and VH backscatter images and four 10-meter multispectral bands of Sentinel-2 were used to create six land cover classification images across two study areas along the border of the Bolivian Pando Department and the Brazilian state of Acre. Results indicate that Sentinel-2 multispectral bands possess a higher overall performance in delineating land cover types than the Sentinel-1 backscatter bands. Sentinel-1 backscatter bands delineated land cover types based on their surficial properties but did not facilitate the separation of similarly textured classes. The combination of Sentinel-1 and -2 resulted in higher accuracy for delineating land cover through increasing the accuracy in delineating the classes of secondary vegetation from exposed soil. While Sentinel-2 demonstrated the capability to consistently capture land cover in both case studies, there is potential for single date Sentinel-1 backscatter image to act as ancillary information in Sentinel-2 scenes affected by clouds or for increasing separability across classes of mixed multispectral qualities but distinct surficial roughness, such as bare ground versus sparsely vegetation areas. Dr. Florencia Sangermano, Chief Instructor ii ACADEMIC HISTORY Name: Aaron Meneghini Date: May 2019 Baccalaureate Degree: B.S. Environmental Science Source: University of Massachusetts, Lowell Date: May 2017 Occupation and Academic Connection since date of baccalaureate degree: Clark University, M.S. in Geographic Information Science for Development and the Environment iii Acknowledgements I wish to thank my research advisor, Professor Florencia Sangermano, whose support, guidance, and encouragement enabled me to pursue this project. I am profoundly grateful for her expertise and patience and I consider it a privilege to have had the opportunity to work with her. I am also grateful to my family and friends, for their unwavering support throughout my academic career. iv Table of Contents List of Illustrations Tables vi Figures vii Introduction 1 Roles of Remote Sensing 2 Background of Synthetic Aperture Radar 2 Copernicus Program 4 Sentinel-2 5 Sentinel-1 7 Combing Sentinel-1 and -2 10 Research Goals 11 Methods 12 Results 17 Discussion 21 Conclusion 25 Figures 26 Literature Cited 44 Appendixes 47 v List of Illustrations Tables: Table 1. Sensor Specifications for Sentinel-1 and Sentinel-2 26 Table 2. Data Products 26 Table 3. Case 1 Summary Table of Accuracy Assessment 43 Table 4. Case 2 Summary Table of Accuracy Assessment 44 Table 5. Confusion Matrices for Case Study 1 44 Table 6. Confusion Matrices Case Study 2 45 Table 8. Cross Tabulation Matrices, Case Study 1 46 Table 9. Cross Tabulation Matrices, Case Study 2 47 vi List of Illustrations Figures: Figure 1. Study Area Map 27 Figure 2. Case Study 1 and 2 False Color Composites 28 Figure 3. Training Sit Locations 29 Figure 4. Training Site Backscatter Values – Case Study 1 30 Figure 5. Training Site Backscatter Values – Case Study 2 31 Figure 6. Spectral Signature Plots 32 Figure 7. Classification Results – Case Study 1 33 Figure 8. Classification Results – Case Study 2 34 Figure 9. Accuracy Assessment Points – Case Study 1 35 Figure 10. Accuracy Assessment Points – Case Study 2 36 Figure 11. Map Accuracy 37 Figure 12. Class Accuracy Case Study 1 37 Figure 13. Class Accuracy Case Study 2 38 Figure 14. Cross tabulation of Sentinel – 1 and Sentinel – 2 Classification Case Study 1 39 Figure 15. Cross tabulation of Sentinel – 1 and Sentinel – 1 and – 2 Classification Case Study 1 40 Figure 16. Cross tabulation of Sentinel – 2 and Sentinel – 1 and – 2 Classification Case Study 1 41 Figure 17. Cross tabulation of Sentinel – 1 and Sentinel – 2 Classification Case Study 2 42 Figure 18. Cross tabulation of Sentinel – 1 and Sentinel – 1 and – 2 Classification Case Study 2 43 Figure 19. Cross tabulation of Sentinel – 2 and Sentinel – 1 and – 2 Classification Case Study 2 44 vii 1.1 Introduction Accounting for nearly 30% of the world’s land cover, forested ecosystems contain great quantities of the world’s biodiversity, are fundamental providers of global ecosystem services, and are quintessential carbon sinks (Morley, 2000; Fagan and DeFries, 2009). Over the past 25 years, these ecosystems have been experiencing net losses as forests are degraded and transformed for anthropogenic use, particularly in tropical regions (FRA, 2015; UNFCCC, 2014; Müller et al., 2014). This landscape transformation has large impacts on biodiversity through changing habitat availability and straining established biogeochemical cycling (Skinner and Murk, 2011). At regional scales deforestation represents a significant loss in carbon reservoirs, without which, the effects of greenhouse gases would be substantial on global climate, through carbon introduction into atmospheric cycling (Skinner and Murk, 2011; Rahman M. and Tetuko Sri Sumantyo J., 2010). Given the essential roles played by forest ecosystems on regional and global scales, international concerns have been raised over the effects of net forest loss due to clear-cut deforestation and forest structure degradation (UNFCCC, 2014). To mitigate the potentially disastrous effects of climate change and biodiversity loss, the program “Reducing Emissions from Deforestation and Forest Degradation” (REDD+) was instituted by the United Nations Framework Convention on Climate Change (UNFCCC) (Pistorius et al 2012; UNFCCC, 2014). Countries participating in this voluntary program have the potential to receive economic benefits for reducing deforestation and degradation in their country (UNFCCC, 2014). However, the dispersal of these financial incentives are reliant on the quantification of national carbon inventories through measurement, reporting and verification systems (MRVs) (Mitchel et al. 2017). These systems are built through the operational implementation of satellite earth observation missions complemented by ground-based forest assessments (Mitchell et al., 2017; Violini, 2013). 1.2 Roles of Remote Sensing: 1 For almost 40 years, optical satellite missions such as Landsat, Landsat Thematic Mapper, Satellite Pour l’Observation de la Terre (SPOT), and the Moderate Resolution Imaging Spectroradiometer (MODIS), have been used to capture and quantify land cover globally (Mitchell et al. 2017). These missions operate through optically capturing the visible to shortwave infrared wavelengths of the electromagnetic spectrum (Mitchell et al. 2017; Joshi et al., 2016). As they pass over landscapes, these optical platforms scan illuminated objects through push broom, line scanner, or framing camera sensors (Warner, 2009; Chuvieco, 2016). For REDD+ mapping and other monitoring projects, the scanned images are transformed into maps of discrete and quantified land cover classes through classification techniques. Despite the global implementation of optical platforms, challenges remain for capturing scenes of regions effected by persistent cloud cover (Haack and Mahabir 2018). To capture areas affected by persistent cloud cover, sensors can comprise between high revisit time and spatial resolution, where a higher sensor revisit time enables the acquisition of cloudless scenes at the cost of spatial resolution (Warner, 2009). However, there is potential for meeting these requirements with recent developments of synthetic aperture radar (SAR) and high spatial resolution multispectral satellite constellations (Mitchell et al. 2017). 1.3 Background of Synthetic Aperture Radar (SAR): SAR platforms can penetrate cloud cover and observe negligible atmospheric effects, especially in wavelengths that are greater than
Details
-
File Typepdf
-
Upload Time-
-
Content LanguagesEnglish
-
Upload UserAnonymous/Not logged-in
-
File Pages59 Page
-
File Size-