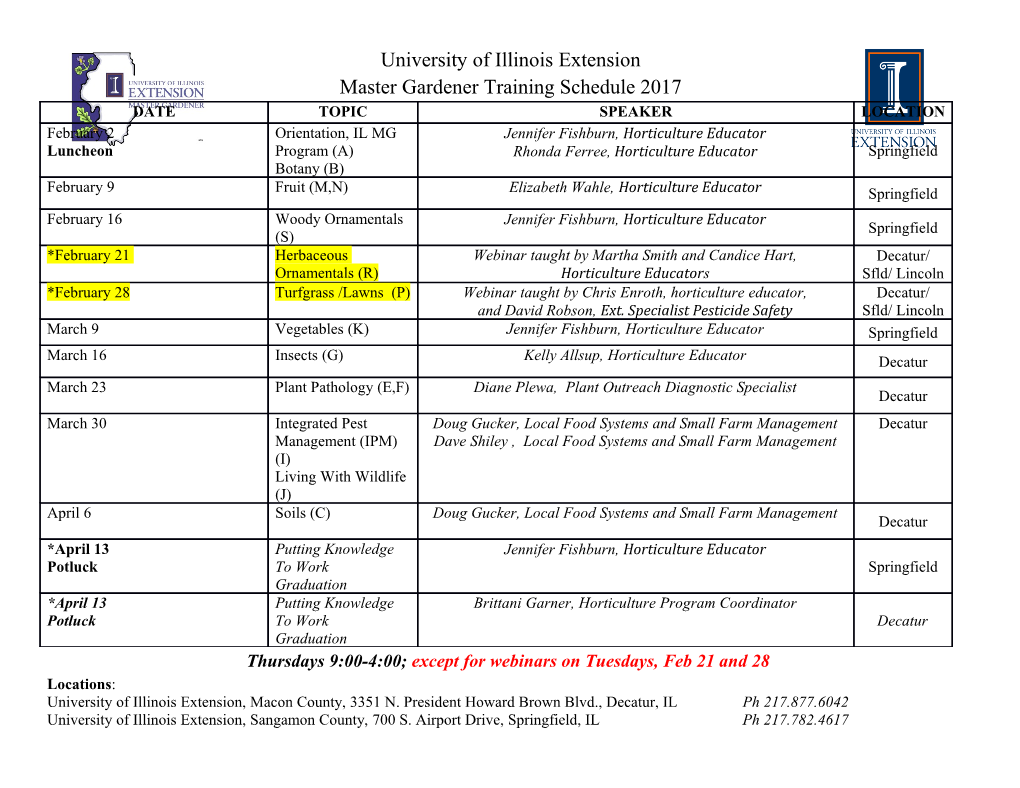
www.nature.com/scientificreports OPEN Systems biology comprehensive analysis on breast cancer for identifcation of key gene modules and genes associated with TNM‑based clinical stages Elham Amjad1,3, Solmaz Asnaashari1,3, Babak Sokouti1* & Siavoush Dastmalchi1,2* Breast cancer (BC), as one of the leading causes of death among women, comprises several subtypes with controversial and poor prognosis. Considering the TNM (tumor, lymph node, metastasis) based classifcation for staging of breast cancer, it is essential to diagnose the disease at early stages. The present study aims to take advantage of the systems biology approach on genome wide gene expression profling datasets to identify the potential biomarkers involved at stage I, stage II, stage III, and stage IV as well as in the integrated group. Three HER2-negative breast cancer microarray datasets were retrieved from the GEO database, including normal, stage I, stage II, stage III, and stage IV samples. Additionally, one dataset was also extracted to test the developed predictive models trained on the three datasets. The analysis of gene expression profles to identify diferentially expressed genes (DEGs) was performed after preprocessing and normalization of data. Then, statistically signifcant prioritized DEGs were used to construct protein–protein interaction networks for the stages for module analysis and biomarker identifcation. Furthermore, the prioritized DEGs were used to determine the involved GO enrichment and KEGG signaling pathways at various stages of the breast cancer. The recurrence survival rate analysis of the identifed gene biomarkers was conducted based on Kaplan–Meier methodology. Furthermore, the identifed genes were validated not only by using several classifcation models but also through screening the experimental literature reports on the target genes. Fourteen (21 genes), nine (17 genes), eight (10 genes), four (7 genes), and six (8 genes) gene modules (total of 53 unique genes out of 63 genes with involving those with the same connectivity degree) were identifed for stage I, stage II, stage III, stage IV, and the integrated group. Moreover, SMC4, FN1, FOS, JUN, and KIF11 and RACGAP1 genes with the highest connectivity degrees were in module 1 for abovementioned stages, respectively. The biological processes, cellular components, and molecular functions were demonstrated for outcomes of GO analysis and KEGG pathway assessment. Additionally, the Kaplan–Meier analysis revealed that 33 genes were found to be signifcant while considering the recurrence-free survival rate as an alternative to overall survival rate. Furthermore, the machine learning calcifcation models show good performance on the determined biomarkers. Moreover, the literature reports have confrmed all of the identifed gene biomarkers for breast cancer. According to the literature evidence, the identifed hub genes are highly correlated with HER2-negative breast cancer. The 53-mRNA signature might be a potential gene set for TNM based stages as well as possible therapeutics with potentially good performance in predicting and managing recurrence-free survival rates at stages I, II, III, and IV as well as in the integrated group. Moreover, the identifed genes for the TNM-based stages can also be used as mRNA profle signatures to determine the current stage of the breast cancer. 1Biotechnology Research Center, Tabriz University of Medical Sciences, Tabriz, Iran. 2School of Pharmacy, Tabriz University of Medical Sciences, Tabriz, Iran. 3These authors contributed equally: Elham Amjad and Solmaz Asnaashari. *email: [email protected]; [email protected] SCIENTIFIC REPORTS | (2020) 10:10816 | https://doi.org/10.1038/s41598-020-67643-w 1 Vol.:(0123456789) www.nature.com/scientificreports/ Breast cancer (BC) is one of the most common health threatening problems among women in the world, leading to death of those patients with BC1. It has been reported in 2019 that the incidence and mortality of breast cancer worldwide are 24.2% and 15.0%, respectively, deserving more attention from healthcare systems and policy- makers1. To clinically classify the status of breast cancer, the American Joint Committee on Cancer (AJCC) has announced eight editions on the Tumor-Node-Metastasis (TNM)-based staging of breast cancer, specifcally for treatment and prognosis2,3. Since more than 50% of the afected patients were died, increasing the survival rate of these patients is highly important by determining the stage of the disease. Te earlier the identifcation of the stage, the more superior the survival rate. To increase the therapeutic efciency and consider the molecular portrait diferences in BC along with their diferent clinical outcomes4, breast cancer can be classifed into six main subtypes, including normal-like, luminal A, luminal B, HER2-positive, basal-like, and claudin-low5; the classifcation has also been confrmed by the Cancer Genome Atlas (TCGA) program6. It has been frequently reported that the human epidermal growth factor receptor (HER) family (i.e., HER-1, HER-2, HER-3, and HER-4) plays a pivotal role in various cancers 7. Among them, HER-2 (known as HER-2/neu gene), as an oncogene with 1,255 amino acids and 185kD transmembrane glycoprotein with tyrosine kinase activ- ity, is located at chromosome 177,8. Moreover, HER-2/neu gene makes breast cancer classifed as HER2-positive and HER2-negative9. In 15–30% of patients with invasive breast carcinomas, an overexpression or amplifcation of HER2 has been identifed7,10. It is worth mentioning that is not efective for HER2-negative. Although, endocrine therapy is the target of chemotherapy, there are no successful reports for survival rates of these types of patients in the literature11. Moreover, several traditional diagnostic approaches such as mammography, magnetic resonance imaging (MRI), ultrasound, computerized tomography (CT), positron emission tomography (PET), and biopsy have been studied in breast cancer diagnosis12. Nowadays, molecular biomarkers have been proposed to provide more efciency in the prognosis and diag- nosis of cancers in defciency of traditional cancer tests. Additionally, the biomarkers are now regularly utilized to better understand the development of the tumors13. Hence, owing to the large number of stored microarray gene expression profles by several genomics laboratories in the most publicly available database websites such as National Center for Biotechnology Information (NCBI), their analyses by various bioinformatics and systems biology analyses are essential4. Finally, these biomarkers will be helpful in personalizing the treatments for each patient with their special stage of the disease4. Considering the HER2-targeted therapy, there are still no predic- tive biomarkers validated for the prognosis and diagnosis of the stages of breast cancer14,15. Consequently, the aim of the current study is to identify the potential biomarkers in breast cancer at stages I, II, III, IV as well as in the integrated group simultaneously regarded as one. To reach this aim, three microarray gene expression profling datasets have been included to identify the diferentially expressed genes (DEGs). By prioritizing those DEGs, their cellular and molecular functions will be further analyzed. Ten, the involved GO (Gene Ontology) and KEGG (Kyoto Encyclopedia of Genes and Genomes) signaling pathways will be studied. Moreover, the protein–protein interaction network for all stages are developed based on the STRING database, and the signifcant hub genes are identifed by clustering algorithm from which the gene biomarkers will later be determined based on their higher connectivity degrees. Finally, the Kaplan–Meier analysis tool was used to assess recurrence-free survival rates of the identifed gene biomarkers. Materials and methods Figure 1 presents the summarization of the fowchart diagram of the approach to satisfy the research question. Data sources. All the datasets used in this study were retrieved from the NCBI GEO database (i.e., https :// www.ncbi.nlm.nih.gov/geo/). Te platform and fle type of the breast cancer microarray datasets were GPL96 [HG-U133A] Afymetrix Human Genome U133A Array and CEL fles, respectively. To cover the aim of this study, GSE124647, GSE129551, and GSE124646 were used as train set including 140 biopsy samples from meta- static patients with stage IV breast cancer, 147 samples from patients with stages I, II, III, and IV breast cancer, and 10 normal samples (0 percent cancer) out of 100 samples, respectively. Moreover, GSE15852 (i.e., includes 43 normal, 8 grade 1 ~ stage I, 23 grade 2 ~ stage II, and 12 grade 3 ~ stage III samples) was used as a test set for external validation. Data preprocessing and identifcation of diferentially expressed genes (DEGs). Te BRB- ArrayTools (v4.6.0, stable version), an excel graphical user interface (GUI) for communicating with R (v 3.5.1) programming environment developed by Dr. Richard Simon and the BRB-ArrayTools Development Team, was used for all stages of preprocessing (i.e., data import, data fltering, and normalization), gene annotation using “hthgu133a.db” R annotation package16 and identifcation of DEGs. During the data import phase, Microarray Suite version 5.0 (MAS 5.0) algorithm was utilized, and then spot fltering, quantile normalization, and gene fltering (gene exclusion criteria of fold change ≤ 2 with expression data values less than %20) were carried out. Next, class comparison between groups of arrays in terms of their label classifcation was performed
Details
-
File Typepdf
-
Upload Time-
-
Content LanguagesEnglish
-
Upload UserAnonymous/Not logged-in
-
File Pages14 Page
-
File Size-