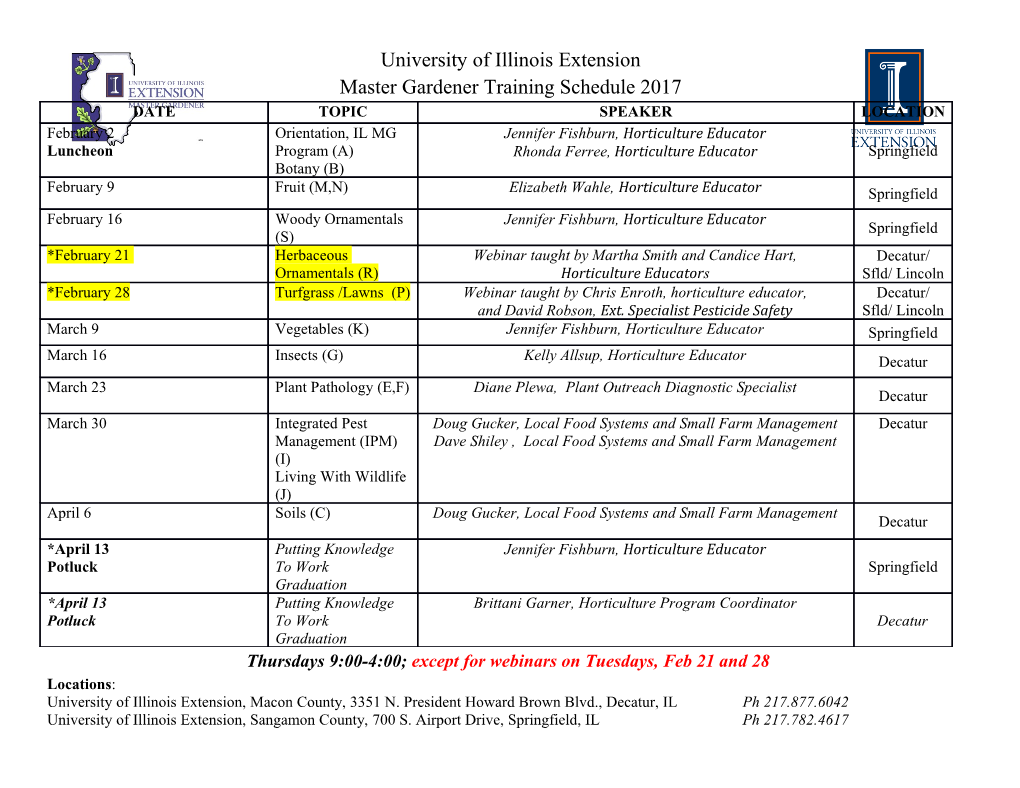
Paradigmatic Morphology and Subjectivity Mark-Up in the RoWordNet Lexical Ontology Dan Tufiş Romanian Academy Research Institute for Artificial Intelligence [email protected] Abstract. Lexical ontologies are fundamental resources for any linguistic application with wide coverage. The reference lexical ontology is the ensemble made of Princeton WordNet, a huge semantic network, and SUMO&MILO ontology, the concepts of which are labelling each synonymic series of Princeton WordNet. This lexical ontology was developed for English language, but currently there are more than 50 similar projects for languages all over the world. RoWordNet is one of the largest lexical ontologies available today. It is sense-aligned to the Princeton WordNet 2.0 and the SUMO&MILO concept definitions have been translated into Romanian. The paper presents the current status of the RoWordNet and some recent enhancement of the knowledge encoded into it. Keywords: lexical ontology, paradigmatic morphology, opinion mining, Romanian language, subjectivity priors. 1 Introduction Most difficult problems in natural language processing stem from the inherent ambiguous nature of the human languages. Ambiguity is present at all levels of traditional structuring of a language system (phonology, morphology, lexicon, syntax, semantics) and not dealing with it at the proper level, exponentially increases the complexity of the problem solving. Most of the successful commercial applications in language processing (text and/or speech) use various shortcuts to syntactic analysis (pattern matching, chunking, partial parsing) and, to a large extent, dispense of explicit concern on semantics, with the usual motivations stemming from the computational high costs required by dealing with full syntax and semantics in case of large volumes of data. With recent advances in corpus linguistics and statistical-based methods in NLP, revealing useful semantic features of linguistic data is becoming cheaper and cheaper and the accuracy of this process is steadily improving. Lately, there seems to be a growing acceptance of the idea that multilingual lexical ontologies might be the key towards aligning different views on the semantic atomic units to be used in characterizing the general meaning of various and multilingual documents. Currently, the state of the art taggers (combining various models, strategies and H.-N. Teodorescu, J. Watada, and L.C. Jain (Eds.): Intel. Sys. and Tech., SCI 217, pp. 161–179. springerlink.com © Springer-Verlag Berlin Heidelberg 2009 162 D. Tufiş processing tiers) ensure no less than 97-98% accuracy in the process of morpho- lexical full disambiguation. For such taggers a 2-best tagging1 is practically 100% accurate. Dependency parsers are doing better and better and, for many significant classes of applications, even dependency linking (which is much cheaper than a full dependency parsing) seems to be sufficient. In a Fregean compositional semantics, the meaning of a complex expression is supposed to be derivable from the meanings of its parts, and the way in which those parts are combined. Therefore, one further step is the word-sense disambiguation (WSD) process. The WSD assigns to an ambiguous word (w) in a text or discourse the sense (sk) which is distinguishable from other senses (s1, …, sk-1, sk+1, …, sn) potentially attributable to that word in a given context (ci). Sense inventories are specified by the semantic dictionaries, and they differ from dictionary to dictionary. For instance, in Merriam-Webster dictionary the verb be has listed 11 fine-grained senses and two coarse-grained senses. Longman Dictionary of Contemporary English glosses 15 fine-grained or 3 coarse-grained senses for the same verb. Cambridge Advanced Learner's Dictionary provides four fine-grained and two coarse-grained senses for the verb be. Therefore, when speaking about word-sense discrimination one has to clearly indicate which sense inventory he/she is using. Word-sense disambiguation is generally considered as the most difficult part of the semantic processing required for deep natural language processing. In a limited domain of discourse this problem is alleviated by considering only coarse-grained sense distinctions, relevant for the given domain. Such a solution, although computationally motivated with respect to the universe of discourse considered, has the disadvantage of reduced portability and is fallible when the meanings of words are outside the boundaries of the prescribed universe of discourse. Given the crucial role played by the dictionaries and lexical semantics in the overall description of a language system, it is not surprising the vast amount of work invested in these areas, during the time and all over the world, resulting in different schools, with different viewpoints and endless debates. Turning the traditional dictionaries into machine readable dictionaries proved to be a thorny enterprise, not only because of the technicalities and large amounts of efforts required, but mainly because of the conceptual problems raised by the intended computer use of knowledge and data initially created for human end-users only. All the implicit knowledge residing in a dictionary had to be made explicit, in a standardized representation, easy to maintain and facilitating interoperability and interchange. The access problem (how to find relevant stored information in a dictionary, with minimal search criteria) became central to computational lexicography. For psycho-linguists the cognitive motivations for lexical knowledge representations and their retrieval mechanisms were, at least, of equal relevance for building credible computational artefacts mimicking the mental lexicons. Multilinguality added a new complexity dimension to the set of issues related to dictionary structuring and sense inventories definition. 1 In k-best tagging, instead of assigning each word exactly one tag (the most probable in the given context), it is allowed to have occasionally at most k-best tags attached to a word and if the correct tag is among the k-best tags, the annotation is considered to be correct. Paradigmatic Morphology and Subjectivity Mark-Up 163 2 Princeton WordNet The computational lexicography has been tremendously influenced by the pioneering WordNet project, started in the early 80'ies at Princeton University by a group of psychologists and linguists led by George Miller [11]. WordNet is a special form of traditional semantic networks, very popular in the AI knowledge representation work of the 70'ies and 80'ies. George Miller and his research group developed the concept of a lexical semantic network, the nodes of which represented sets of actual words of English sharing (in certain contexts) a common meaning. These sets of words, called synsets (synonymy sets), constitute the building blocks for representing the lexical knowledge reflected in WordNet, the first implementation of lexical semantic networks. As in the semantic networks formalisms, the semantics of the lexical nodes (the synsets) is given by the properties of the nodes (implicitly, by the synonymy relation that holds between the literals of the synset and explicitly, by the gloss attached to the synset and, sometimes, by specific examples of usage) and the relations to the other nodes of the network. These relations are either of a semantic nature, similar to those to be found in the inheritance hierarchies of the semantic networks, and/or of a lexical nature, specific to lexical semantics representation domains. In mare than 25 years of continuous development, Princeton WordNet [6] (henceforth PWN) reached an impressive coverage and it is the largest freely available semantic dictionary today. The current version, PWN3.02, is a huge lexical semantic network in which almost 120,000 meanings/synsets (lexicalized by more than 155,000 literals) are related by semantic and lexical relations. The lexical stock covers the open class categories and is distributed among four semantic networks, each of them corresponding to a different word class: nouns, verbs, adjectives and adverbs. The notion of meaning in PWN is equivalent to the notion of concept and it is represented, according to a differential lexicographic theory, by a series of words which, in specific contexts, could be mutually substituted. This set of words is called a synset (synonymy set). A word occurring in several synsets is a polysemous one and each of its meanings is distinguished by a sense number. A pair made of a word and a sense number is generically called a word-sense. In the last version of PWN there are 206941 English word-senses. The basic structuring unit of PWN, the synset, is an equivalence relation over the set of word-senses. The major quantitative data about this unique lexical resource for English is given in the table 1 and table 2. Table 1. POS distribution of the synsets and word s-senses in WPN 3.0 Noun Verb Adjective Adverb Total literal/synset/ literal/synset/ literal/synset/ literal/synset/ literal/synset/ sense sense sense sense sense 117798/82115/ 11529/13767/ 21479/18156/ 4481/3621/ 155287/117659/ 146312 25047 30002 5580 206941 2 http://www.cogsci.princeton.edu/~wn/ 164 D. Tufiş Table 2. Polysemy in PWN 3.0 Noun Verb Adjective Adverb Total literal/sense literal/sense literal/sense literal/sense literal/sense 15935/44449 5252/18770 4976/14399 733/1832 26896/79450 Information in Table 1 shows that most of the literals, synsets and word-senses are given by the noun grammatical category: 117798 literals (75,85%), altogether with 146312 word-senses (70,70%) are clustered into 82115 synonymy equivalence classes (69,79% synsets). The data in Table 2, show that only a small part of the lexical stock is polysemous, many nouns, verbs, adjectives and adverbs being monosemous. For instance, only 15935 nouns, that is 13.52%, occur in two or more synsets, all of them having 44449 word-senses, representing 30.37% of the total number of noun senses. The relations among the synsets differ, being dependent on the grammar category of the literals in a synset. For each relation there is a reverse one. The major relations in PWN are: synonymy, hypernymy, meronymy (for nouns), troponymy, entailment (for verbs).
Details
-
File Typepdf
-
Upload Time-
-
Content LanguagesEnglish
-
Upload UserAnonymous/Not logged-in
-
File Pages20 Page
-
File Size-