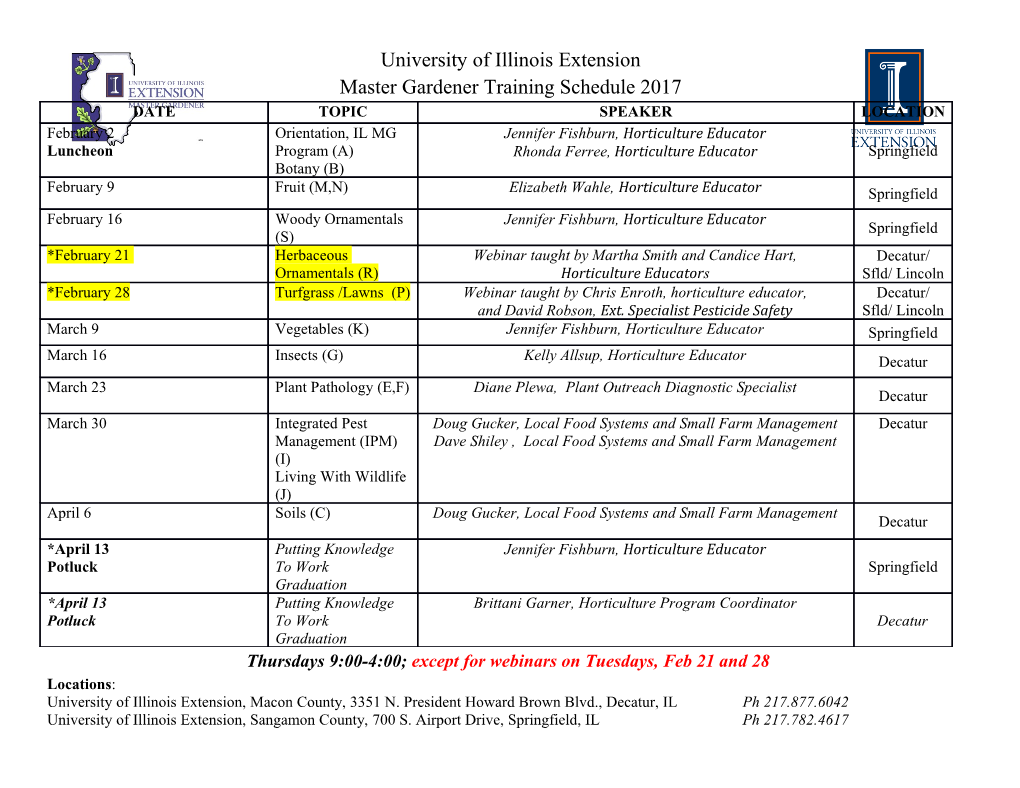
Connectionism and Classical Conditioning Michael R.W. Dawson University of Alberta Edmonton, Alberta Canada T6G 2P9 1-(780)-492-5175 Abstract The purpose of this monograph is to examine the relationship between a par- ticular artificial neural network, the perceptron, and the Rescorla-Wagner model of learning. It is shown that in spite of the fact that there is a formal equivalence be- tween the two, they can make different predictions about the outcomes of a num- ber of classical conditioning experiments. It is argued that this is due to algo- rithmic differences between the two, differences which are separate from their computational equivalence. [email protected] Rosenblatt Software Available As Freeware At: http://www.bcp.psych.ualberta.ca/~mike/Software/Rosenblatt/index.html A peer-reviewed monograph published by Comparative Cognition and Behavior Reviews on behalf of the Comparative Cognition Society. © Michael R. W. Dawson 2008 Chapter 1: Learning in Networks and Animals_____________________________ 9 1.1 An Empirical Puzzle __________________________________________________ 10 1.1.1 Identical, But Different _____________________________________________________ 10 1.1.2 Networks and Learning_____________________________________________________ 10 1.2 A Formal Surprise____________________________________________________ 11 1.2.1 Perceptron Nonlinearity ____________________________________________________ 11 1.2.2 A Linear Proof ___________________________________________________________ 11 1.2.3 A Nonlinear Proof_________________________________________________________ 11 1.3 Cognitive Science and Associative Learning_______________________________ 12 1.3.1 Cognition vs. Association ___________________________________________________ 12 1.3.2 Levels and Equivalence ____________________________________________________ 12 1.4 The Synthetic Approach _______________________________________________ 13 1.4.1 The Analytic Approach_____________________________________________________ 13 1.4.2 A Synthetic Alternative_____________________________________________________ 13 1.4.3 Synthetic Association ______________________________________________________ 13 1.5 Purposes and Intended Readership ______________________________________ 14 1.5.1 Purposes ________________________________________________________________ 14 1.5.2 Intended Readership _______________________________________________________ 14 1.6 What Is This Book Not About? _________________________________________ 15 1.6.1 Multi-layer Perceptrons ____________________________________________________ 15 1.6.2 Temporal Models _________________________________________________________ 15 1.6.3 Rescorla-Wagner Alternatives _______________________________________________ 15 1.7 What Is This Book About? _____________________________________________ 16 1.7.1 What Lies Ahead _________________________________________________________ 16 1.7.2. What Lay Behind_________________________________________________________ 16 Chapter 2: The Perceptron ____________________________________________ 17 2.1 Neuronal Inspiration __________________________________________________ 18 2.1.1 Functional Explanations ____________________________________________________ 18 2.1.2 Neuronal Function ________________________________________________________ 18 2.2 A Digital Output Unit _________________________________________________ 19 2.2.1 Perceptron as Neuron ______________________________________________________ 19 2.2.2 Computing Net Input ______________________________________________________ 19 2.2.3 Converting Net Input to Activity _____________________________________________ 19 2.3 Activation and Response_______________________________________________ 20 2.3.1 What Is Activity? _________________________________________________________ 20 2.3.2 Activity and Response _____________________________________________________ 20 2.4 Association in the Perceptron___________________________________________ 21 2.4.1 Stimulus and Response _____________________________________________________ 21 2.4.2 UCS→UCR in the Perceptron _______________________________________________ 21 2.5 Multiple Associations _________________________________________________ 22 2.5.1 Multiple Inputs and Outputs _________________________________________________ 22 2.5.2 An Example Perceptron ____________________________________________________ 22 2.6 Learning in Perceptrons _______________________________________________ 23 2.6.1 Neurons That Learn _______________________________________________________ 23 2.6.2 Supervised Learning _______________________________________________________ 23 A peer-reviewed monograph published by Comparative Cognition and Behavior Reviews on behalf of the Comparative Cognition Society. © Michael R. W. Dawson 2008 2.7 Hebb Learning _______________________________________________________ 24 2.7.1 The Law of Exercise_______________________________________________________ 24 2.7.2 The Hebb Rule ___________________________________________________________ 24 2.8 Pros and Cons of Hebb Learning________________________________________ 25 2.8.1 Hebb Rule Advantages _____________________________________________________ 25 2.8.2 Hebb Rule Disadvantages___________________________________________________ 25 2.8.3 Learning from Mistakes ____________________________________________________ 25 2.9 The Delta Rule _______________________________________________________ 26 2.9.1 Defining Error____________________________________________________________ 26 2.9.2 Delta Learning Of Associations ______________________________________________ 26 2.9.3 Supervised Learning and US_________________________________________________ 26 Chapter 3: Associative Learning in the Perceptron: Case Studies _____________ 27 3.1 Studying Associative Learning in the Perceptron __________________________ 28 3.1.1 Setting the Stage __________________________________________________________ 28 3.1.2 Technical Details _________________________________________________________ 28 3.2 Simple Association____________________________________________________ 29 3.2.1 Classical Conditioning _____________________________________________________ 29 3.2.2 Training Set _____________________________________________________________ 29 3.2.3 Results__________________________________________________________________ 29 3.3 Intensity of Conditioned Stimuli ________________________________________ 30 3.3.1 Conditioning and CS Intensity _______________________________________________ 30 3.3.2 CS Intensity in the Perceptron _______________________________________________ 30 3.3.3 Results__________________________________________________________________ 30 3.4 Intensity of Unconditioned Stimuli ______________________________________ 31 3.4.1 The Effect of US Intensity __________________________________________________ 31 3.4.2 Effects of Decreasing η ____________________________________________________ 31 3.4.2 Effects of Increasing η _____________________________________________________ 31 3.5 Configural Representations of Compound Stimuli _________________________ 32 3.5.1 Compound Stimuli ________________________________________________________ 32 3.5.2 Elemental Representations __________________________________________________ 32 3.5.2 Configural Representation __________________________________________________ 32 3.6 Positive Patterning In The Perceptron ___________________________________ 33 3.6.1 Positive Patterning ________________________________________________________ 33 3.6.2 Training Set _____________________________________________________________ 33 3.6.3 Results__________________________________________________________________ 33 3.6.4 The Modern Perceptron ____________________________________________________ 33 Chapter 4: Modernizing the Perceptron: The Integration Device _____________ 34 4.1 A Continuous Approximation of the Heaviside Equation ____________________ 35 4.1.1 From Discrete To Continuous________________________________________________ 35 4.1.2 The Logistic Activation Function _____________________________________________ 35 4.2 Training an Integration Device _________________________________________ 36 4.2.1 The Delta Rule ___________________________________________________________ 36 4.3 Acquisition Curves for the Integration Device _____________________________ 37 4.3.1 General Method __________________________________________________________ 37 4.3.2 Association and Extinction __________________________________________________ 37 4.3.2 Results__________________________________________________________________ 37 4.4 On Two Responses____________________________________________________ 38 A peer-reviewed monograph published by Comparative Cognition and Behavior Reviews on behalf of the Comparative Cognition Society. © Michael R. W. Dawson 2008 4.4.1 Go or No Go _____________________________________________________________ 38 4.4.2 Approach or Avoid ________________________________________________________ 38 4.5 The Hyperbolic Integration Device ______________________________________ 39 4.5.1 The Hyperbolic Tangent ____________________________________________________ 39 4.5.2 Hyperbolic Learning Rule___________________________________________________ 39 4.6 Approach or Avoid ___________________________________________________ 40 4.6.1 Inhibition and Excitation____________________________________________________ 40 4.6.2 Training Set _____________________________________________________________ 40 4.6.3 Results__________________________________________________________________
Details
-
File Typepdf
-
Upload Time-
-
Content LanguagesEnglish
-
Upload UserAnonymous/Not logged-in
-
File Pages115 Page
-
File Size-