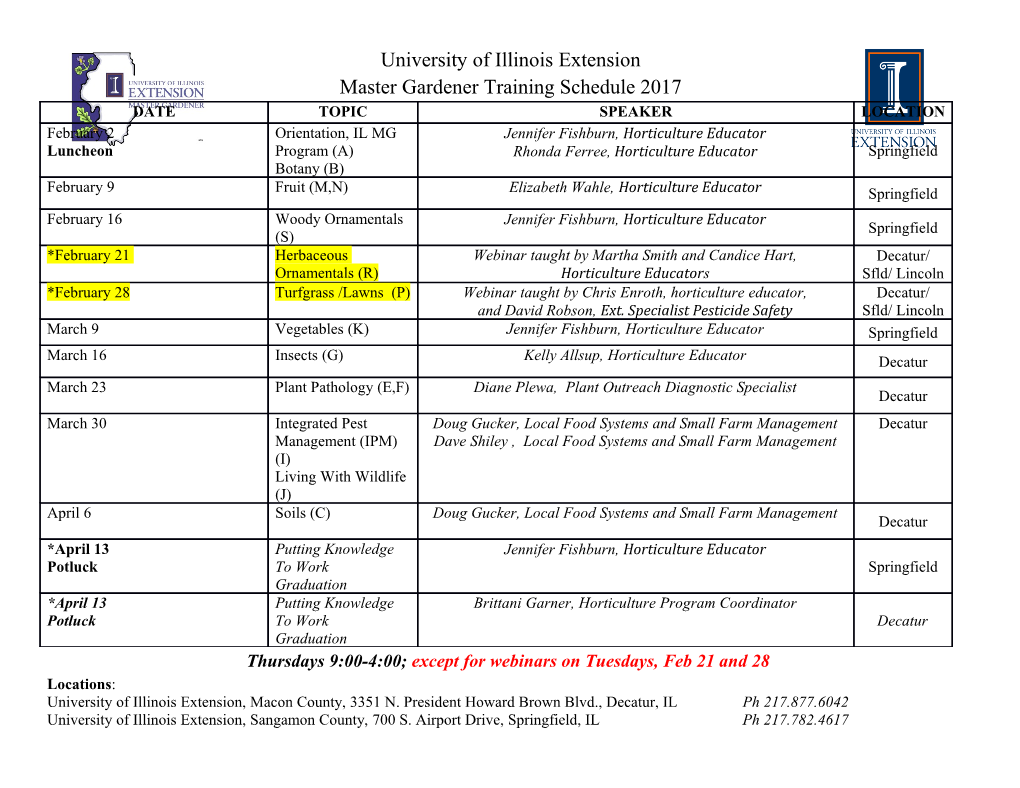
This thesis has been submitted in fulfilment of the requirements for a postgraduate degree (e.g. PhD, MPhil, DClinPsychol) at the University of Edinburgh. Please note the following terms and conditions of use: This work is protected by copyright and other intellectual property rights, which are retained by the thesis author, unless otherwise stated. A copy can be downloaded for personal non-commercial research or study, without prior permission or charge. This thesis cannot be reproduced or quoted extensively from without first obtaining permission in writing from the author. The content must not be changed in any way or sold commercially in any format or medium without the formal permission of the author. When referring to this work, full bibliographic details including the author, title, awarding institution and date of the thesis must be given. Multimodal Sensing for Robust and Energy-Efficient Context Detection with Smart Mobile Devices Valentin Radu Doctor of Philosophy Institute of Computing Systems Architecture School of Informatics University of Edinburgh 2017 Abstract Adoption of smart mobile devices (smartphones, wearables, etc.) is rapidly grow- ing. There are already over 2 billion smartphone users worldwide [1] and the per- centage of smartphone users is expected to be over 50% in the next five years [2]. These devices feature rich sensing capabilities which allow inferences about mobile device user’s surroundings and behavior. Multiple and diverse sensors common on such mobile devices facilitate observing the environment from different perspectives, which helps to increase robustness of inferences and enables more complex context detection tasks. Though a larger number of sensing modalities can be beneficial for more accurate and wider mobile context detection, integrating these sensor streams is non-trivial. This thesis presents how multimodal sensor data can be integrated to facilitate ro- bust and energy efficient mobile context detection, considering three important and challenging detection tasks: indoor localization, indoor-outdoor detection and human activity recognition. This thesis presents three methods for multimodal sensor inte- gration, each applied for a different type of context detection task considered in this thesis. These are gradually decreasing in design complexity, starting with a solution based on an engineering approach decomposing context detection to simpler tasks and integrating these with a particle filter for indoor localization. This is followed by man- ual extraction of features from different sensors and using an adaptive machine learn- ing technique called semi-supervised learning for indoor-outdoor detection. Finally, a method using deep neural networks capable of extracting non-intuitive features di- rectly from raw sensor data is used for human activity recognition; this method also provides higher degree of generalization to other context detection tasks. Energy efficiency is an important consideration in general for battery powered mo- bile devices and context detection is no exception. In the various context detection tasks and solutions presented in this thesis, particular attention is paid to this issue by relying largely on sensors that consume low energy and on lightweight computations. Overall, the solutions presented improve on the state of the art in terms of accuracy and robustness while keeping the energy consumption low, making them practical for use on mobile devices. i Acknowledgements I had the pleasure of meeting many wonderful people during my PhD journey, who influenced my research in various ways. This great learning experience would not have been the same without them and I owe a great deal of this achievement to them. Firstly, I would like to extend my deepest gratitude to my supervisor Mahesh K. Marina for all the support, guidance and patience I received throughout my PhD. I learned from him to strive for quality of research with the potential to make an impact. His unfailing positive personality made this an enjoyable experience and I appreciate his good disposition in many social events. I am forever indebted for the valuable lessons learned from him, which will serve me all my life. I am very thankful to my other supervisors, Hugh Leather and Tristan Henderson for very insightful discussions and critical analysis of my approach, steering my research in the right direction. Work- ing closely with Panagiota Katsikouli and Rik Sarkar was a real pleasure and I am very grateful for this fruitful collaboration. I am very grateful for the opportunity to work with Nicholas Lane, who guided me through a new research topic and was always available to offer support. I am also very grateful to Cecilia Mascolo who kindly welcomed me into her research lab at Cambridge, making me feel part of the group and for providing me with resources and advice throughout my stay there. Many thanks to Sourav Bhattacharya for the guided discussions on neural networks. Their great experience in top level research pushed me to improve my work. Many thanks to Myungjin Lee for giving me access to his computer cluster and to Charles Sutton for insightful discussions on machine learning techniques. I am thankful to my thesis examiners, Hamed Haddadi and Michael O’Boyle, for accepting to evaluate this thesis on such short notice and for their valuable feedback to improve the presentation of this work. I feel fortunate to have worked next to some amazing colleagues over the years, cre- ating a productive and enjoyable atmosphere in the lab, Lito Kriara, Arsham Farshad, Saravana Rathinakumar, Galini Tsoukaneri, Mohamed Kassem, Konstantinos Balam- pekos, Jiwei Li, Emilian Radoi, Daniel Duma and Cosmin Dumitrache. Also, special thanks to Imke van Ettinger for putting up with me in the more stressful moments. Not least, I want to thank my family for their love and continuous support. Their en- couragements has been invaluable throughout this journey. I thank my sister, Daniela, for taking more responsibilities in the family to give me space to concentrate on this work. I am very lucky to have such a loving family. ii Declaration I declare that this thesis was composed by myself, that the work contained herein is my own except where explicitly stated otherwise in the text, and that this work has not been submitted for any other degree or professional qualification except as specified. (Valentin Radu) iii Table of Contents 1 Introduction 1 1.1 Mobile Context Sensing . .3 1.2 Challenging Context Detection Tasks . .4 1.2.1 Indoor Localization . .4 1.2.2 Indoor-Outdoor Detection . .6 1.2.3 Human Activity Recognition . .7 1.3 Energy Efficiency . .8 1.4 Contributions . .9 1.4.1 Indoor Localization . .9 1.4.2 Indoor-Outdoor Detection . 10 1.4.3 Deep Learning for Human Activity Recognition and Other Con- text Detection . 12 1.5 Structure . 13 2 Background and Related Work 15 2.1 Previous Work on Mobile Context Detection . 15 2.1.1 Indoor Localization . 15 2.1.2 Indoor-outdoor Detection . 18 2.1.3 Human Activity Recognition . 19 2.1.4 Other Contexts . 20 2.2 Multimodal Sensing and Learning Approaches . 21 2.2.1 Machine learning with hand-crafted features . 22 2.2.2 Particle Filter . 22 2.2.3 Deep Learning . 23 3 A Hybrid Particle Filter based Approach for Indoor Localization 25 3.1 Design and Implementation . 26 iv 3.1.1 HiMLoc Hybrid Localization Mechanism Overview . 26 3.1.2 Pedestrian Dead Reckoning Component . 27 3.1.3 WiFi Fingerprinting Component . 32 3.1.4 Fusion via Particle Filter . 34 3.2 HiMLoc Evaluation . 36 3.3 Pazl: Using HiMLoc for Crowdsourced Indoor Wireless Network Mon- itoring . 39 3.3.1 Pazl System Components . 40 3.3.2 Case Study 1: Indoor WiFi Monitoring . 41 3.3.3 Case Study 2: Indoor Cellular Coverage Measurement . 44 3.4 Discussion . 45 3.5 Summary . 46 4 A Semi-Supervised Learning based Approach for Indoor-Outdoor Detec- tion 47 4.1 Preliminary Exploration and Critique of Existing Indoor-Outdoor De- tection Techniques . 48 4.1.1 GPS based IO Detection . 48 4.1.2 IODetector . 50 4.1.3 Summary of preliminary investigation . 55 4.2 Opportunity for Indoor-Outdoor Detection with Machine Learning . 55 4.3 Large Experiment across Multiple Environments . 57 4.3.1 Experimental Setup . 57 4.3.2 Baseline with Supervised Learning . 58 4.4 Robust Indoor-Outdoor Detection with Semi-Supervised Learning . 59 4.4.1 Cluster-then-Label . 60 4.4.2 Self-Training . 61 4.4.3 Co-Training . 63 4.4.4 Learning Curve . 66 4.4.5 Learning across Devices . 68 4.4.6 Discussion for Semi-supervised Learning . 70 4.5 Implementation and Evaluation of Mobile Application using Co-Training for IO Detector . 71 4.5.1 Efficient Implementation via Incremental Learning . 72 4.5.2 Power Consumption . 73 v 4.6 Case Study - Mobile App to Control WiFi Interface . 75 4.7 Summary . 77 5 Multimodal Deep Learning for Versatile Mobile Context Sensing 78 5.1 Deep Learning for Multimodal Sensing . 79 5.1.1 Learning representations with Convolutional Neural Networks 82 5.2 Implementation . 83 5.3 Evaluation . 84 5.3.1 Human Activity Recognition . 84 5.3.2 Energy Efficiency and Mobile Hardware Feasibility . 90 5.4 Detection of Other Contexts with Multimodal Deep Learning . 92 5.4.1 Sleep Stage Detection . 93 5.4.2 Indoor-Outdoor Detection . 94 5.5 Landmarks Discrimination . 94 5.5.1 Landmarks and Dataset . 95 5.5.2 Differentiability Between Landmarks . 96 5.5.3 Multimodal RBM for Landmark Differentiation . 98 5.6 Summary . 99 6 Conclusions and Future work 100 6.1 Conclusions . 100 6.1.1 Indoor Localization . 101 6.1.2 Indoor-Outdoor Detection . 101 6.1.3 Context Detection with Deep Learning . 102 6.2 Discussion and Opportunities for Future Work . 102 Bibliography 107 vi Chapter 1 Introduction With the advancement of electronics and processor miniaturization, a new generation of smart mobile devices has emerged for personal computing (i.e., monitoring and private data processing).
Details
-
File Typepdf
-
Upload Time-
-
Content LanguagesEnglish
-
Upload UserAnonymous/Not logged-in
-
File Pages124 Page
-
File Size-