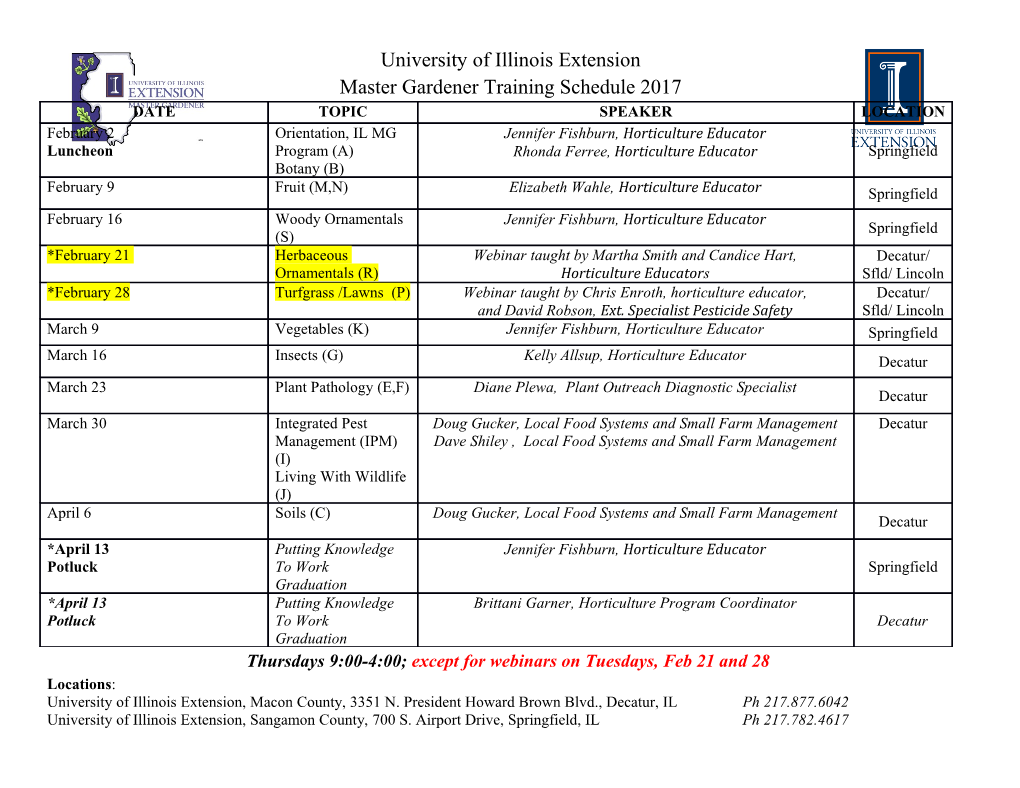
Downloaded from http://jamia.bmj.com/ on November 29, 2014 - Published by group.bmj.com Research and applications Temporal phenome analysis of a large electronic health record cohort enables identification of hospital-acquired complications Jeremy L Warner,1,2 Amin Zollanvari,3,4 Quan Ding,3 Peijin Zhang,5 Graham M Snyder,6 Gil Alterovitz3,7,8 ▸ Additional material is ABSTRACT data, visual analytics has become of increasing published online only. To view Objective To develop methods for visual analysis of prominence.56 please visit the journal online (http://dx.doi.org/10.1136/ temporal phenotype data available through electronic Earlier work has demonstrated that a clinical amiajnl-2013-001861). health records (EHR). phenome, for example, as defined by the prevalence fi fi Materials and methods 24 580 adults from the of International Classi cation of Disease, Ninth For numbered af liations see fi end of article. multiparameter intelligent monitoring in intensive care Revision, Clinical Modi cation (ICD-9-CM) codes V.6 (MIMIC II) EHR database of critically ill patients were or aggregations of such codes, can be used to calcu- Correspondence to analyzed, with significant temporal associations late a phenome-wide association of disease codes – Dr Jeremy L Warner, visualized as a map of associations between hospital with single-nucleotide polymorphisms (SNP).7 9 Department of Medicine, Division of Hematology and length of stay (LOS) and ICD-9-CM codes. An expanded However, most work in this area has focused on Oncology, Vanderbilt phenotype, using ICD-9-CM, microbiology, and dichotomous variables, such as SNP,which are amen- University, 2220 Pierce Ave, computerized physician order entry data, was defined for able to display by conventional probability plotting Preston Research Building 777, hospital-acquired Clostridium difficile (HA-CDI). LOS, (eg, Manhattan and Q-Q plots). In previous work, Nashville, TN 37232, USA; estimated costs, 30-day post-discharge mortality, and we demonstrated that complex phenomic informa- [email protected] antecedent medication provider order entry were tion can be visualized as a function of a continuous Received 31 March 2013 evaluated for HA-CDI cases compared to randomly laboratory value, such as the white blood cell Revised 15 July 2013 selected controls. count.10 As disease conditions are expected to Accepted 16 July 2013 Results Temporal phenome analysis revealed 191 change over time, capturing the continuous temporal Published Online First fi 1 August 2013 signi cant codes (p value, adjusted for false discovery evolution of a phenome could lead to new and valu- rate, ≤0.05). HA-CDI was identified in 414 cases, and able discoveries. For example, hospital-acquired com- was associated with longer median LOS, 20 versus plications are well known to prolong the length of 9 days, and adjusted HR 0.33 (95% CI 0.28 to 0.39). hospitalizations, but may do so to varying degrees. This prolongation carries an estimated annual Information about the temporal associations of spe- incremental cost increase of US$1.2–2.0 billion in the cific hospital-acquired conditions, optimized for USA alone. data visualization, may be evaluable through compre- Discussion Comprehensive EHR data have made large- hensive EHR resources. Previous work on temporal scale phenome-based analysis feasible. Time-dependent phenotype extraction from EHR has focused primar- pathological disease states have dynamic phenomic ily on individual patient trajectories or specific evolution, which may be captured through visual case scenarios (eg, LifeLines,11 LifeLines2,12 analytical approaches. Although MIMIC II is a single VISITORS);13 we propose to examine a large cohort institutional retrospective database, our approach should for undiscovered temporal associations. be portable to other EHR data sources, including prospective ‘learning healthcare systems’. For example, Objective interventions to prevent HA-CDI could be dynamically In this paper, we describe a new method for: visual evaluated using the same techniques. analysis of phenomic associations as a function of Conclusions The new visual analytical method time; and hypothesis generation based on the described in this paper led directly to the identification resultant temporal phenome map. These two steps of numerous hospital-acquired conditions, which could comprise a substantial portion of the ‘learning be further explored through an expanded phenotype healthcare system,’ as shown in figure 1. For a clin- definition. ical use case, we demonstrate how the application of these visual analytical methods can lead to rec- ognition of specific hospital-acquired complica- tions, as a function of the length of inpatient BACKGROUND AND SIGNIFICANCE hospitalization, in a diverse EHR database of critic- Clinical phenomics, the measurement of the diver- ally ill patients. sity of disease states across human subjects, is of increasing importance in the analysis of large clin- MATERIALS AND METHODS ical datasets.1 Electronic health records (EHR) now Data source make it possible to evaluate disease prevalence, dis- Multiparameter intelligent monitoring in intensive fi To cite: Warner JL, tribution, and correlation across a prede ned com- care V.6 (MIMIC II), an EHR-based database of crit- Zollanvari A, Ding Q, et al. prehensive collection of phenotypes (a ‘phenome’) ically ill patients admitted to Beth Israel Deaconess – J Am Med Inform Assoc to a degree that was previously not achievable.2 4 Medical Center between 2001 and 2007, was the – 2013;20:e281 e287. Due to the complexity of EHR and related medical primary data source.14 MIMIC II contains detailed Warner JL, et al. J Am Med Inform Assoc 2013;20:e281–e287. doi:10.1136/amiajnl-2013-001861 e281 Downloaded from http://jamia.bmj.com/ on November 29, 2014 - Published by group.bmj.com Research and applications patients in each subgroup, we explored how varying the health- care exposure duration intervals affected the results. A two-dimensional ‘temporal phenome map’ was rendered by plotting time as a function of ICD-9-CM codes, displaying line segments for significant adjusted p values, with segment length corresponding to the time interval containing the subgroup, and width proportionate to the negative logarithm of the adjusted p value. In the baseline analysis, an additional temporal phenome map with all adjusted p values less than 0.99 was rendered. Visual analysis of the temporal phenome map For the purposes of this pilot project, we limited the scope of extended evaluation to those ICD-9-CM codes in chapter one: Figure 1 Example schema of a ‘learning healthcare system’. This Infectious and parasitic disease. These codes were systematically example demonstrates the ideal flow of a learning healthcare system examined, and those that appeared to be consistent with environment, which begins with data analysis and visualization. Based hospital-acquired complications were selected for further study, on interpretation of these data, potential problems are recognized and with a final selection of one condition for further evaluation: hypotheses are generated. These lead to the development of hospital-acquired Clostridium difficile infection (HA-CDI). interventions to mitigate or improve the identified problems, which are fi then implemented and evaluated in an iterative fashion. The rst two fi steps of this process, represented by solid lines, are described within Expanded case de nitions the paper. We then developed an expanded phenotypic definition for HA-CDI, using medication and microbiology information avail- able in MIMIC II. Cases of HA-CDI were defined by one or information about vital sign parameters, laboratory data, and pro- more of the following occurring at least 48 h after initial vider order entry (POE), including time stamps of ordering, start, contact: (1) a positive assay for C difficile toxin; (2) POE for and stop times for medications. Our analysis was restricted to oral or rectal vancomycin; (3) POE for oral or intravenous adult patients (≥16 years old). All investigators completed appro- metronidazole and ICD-9-CM code 008.45: C difficile. For the priate human subjects training before accessing the data, which is second criterion, treatment of C difficile is the only common classified as institutional review board exempt. This study complies use for oral or rectal vancomycin so the ICD-9-CM code was with the guidelines of the Declaration of Helsinki. not required; conversely, oral or intravenous metronidazole is used to treat other conditions, so the ICD-9-CM code was required for the third criterion. Non-HA-CDI was defined using Duration of healthcare exposure definition the same criteria but with cutoffs before 48 h for case We d e fined healthcare exposure duration as the interval from definition. initial contact with the healthcare system to hospital discharge. In MIMIC II, hospital admissions and discharges are recorded Matching controls to cases as midnight-timed date stamps; intensive care unit (ICU) admis- For the set of HA-CDI cases, the outlying 1st percentile and sion and discharge, POE orders, and laboratory tests are 99th percentile of healthcare exposure duration were excluded recorded with time stamps. We used the first time stamp for a before selection of a matching control group. Candidate con- laboratory test or POE obtained within ±48 h of the admission trols were randomly selected from the remaining MIMIC II date stamp as a proxy for initial contact. For same-day hospital/ cohort, excluding cases of non-HA-CDI. Candidates were ICU discharges, we used the ICU discharge time as the last excluded if their healthcare exposure duration was less than the contact. Otherwise, we used the last time stamp of a laboratory 1st percentile or greater than the 99th percentile of the case test or POE stop order occurring within 24 h of the hospital dis- hospitalizations. If candidates had at least one laboratory value charge date stamp as a proxy for last contact. If there were no measurement during the first 48 h of hospitalization, they were stop orders or labs within 24 h of the discharge date stamp, the included as a control.
Details
-
File Typepdf
-
Upload Time-
-
Content LanguagesEnglish
-
Upload UserAnonymous/Not logged-in
-
File Pages8 Page
-
File Size-