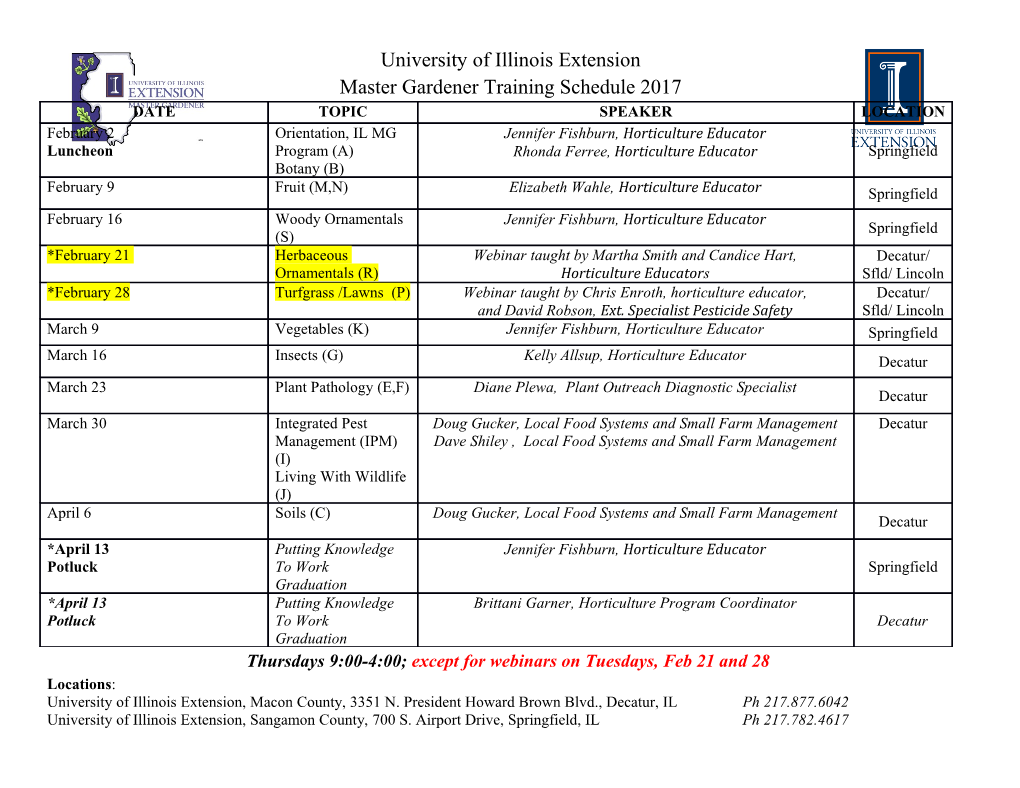
©Thomas Plümper 2007/08 Research Design Thomas Plümper Department of Government University of Essex [email protected] ©Thomas Plümper 2007/08 Organization Lesson 1: Why Research Designs? Lesson 2: What is a good Research Question? Lesson 3: Basic Concepts, Discussions and Axioms in the Philosophy of Science Lesson 4: Causality Lesson 5: Theory Formation Lesson 6: Theory and Empirical Analysis Lesson 7: Qualitative Research Designs and Case Studies Lesson 8: Quantitative Research Designs Lesson 9: Robustness ©Thomas Plümper 2007/08 Lesson 10: Efficient Writing: A Summary of Issues Lesson 11: ©Thomas Plümper 2007/08 Lesson 1: Why Research Design? ©Thomas Plümper 2007/08 What is Science? “Science is a public process. It uses systems of concepts called theories to help interpret and unify observation statements called data; in turn the data are used to check or ‘test’ the theories. Theory creation may be inductive, but demonstration and testing are deductive, although, in inexact subjects, testing will involve statistical inference. Theories that are at once simple, general and coherent are valued as they aid productive and precise scientific practice.” David F. Hendry 1980 ©Thomas Plümper 2007/08 Why Research Design? Axiom 1: A research design is good, if and only if it allows researchers to draw valid inferences. btw: An axiom is a sentence or proposition that is not proved or demonstrated and is considered as self- evident or as an initial necessary consensus for a theory building or acceptation. Nevertheless, axiom 1 leads to two questions: 1. Why should scientists be interested in valid inferences? 2. Can we proof that inferences are valid? ©Thomas Plümper 2007/08 Why should scientists be interested in valid inferences? One step back: What are scientists interested in? ©Thomas Plümper 2007/08 Why (social) scientists should be interested in valid inferences 1 One step back: What are scientists interested in? − maximizing life-time utility (which is a function of income, social status, 1-uncertainty, and so on) − getting tenure − getting cited − publications in a certain type of journals (a book with a very good publisher) or, perhaps more seriously but certainly on a lower plane: − explanations − generalizations − simplifications or, in short − advance scientific knowledge (we repeatedly come back to this statement) ©Thomas Plümper 2007/08 Why (social) scientists should be interested in valid inferences 2 Axiom 2 Eventually, (social) scientists are interested in theories, which are simultaneously as simple and as general as possible. It follows: 1. A simpler theory is better than a more complicated theory which does not explain more. 2. An equally simple theory that explains more is better than a theory that explains less. 3. A more complicated theory that explains more is not per se better than a less complicated theory that explains less. ‘More’ means more cases, more phenomena, … ©Thomas Plümper 2007/08 Why (social) scientists should be interested in valid inferences 3 We can see how complicated a theory is when we see one (or compare it to other theories). We cannot see how valid the generalizations are that the theory makes. Thus: (Social) scientists need to develop theories and test them (test generalizations of the theory). BUT: keep in mind that theories need to simplify. Thus, testing theories means testing whether the prediction of the theory are correct, not whether the assumptions are ‘true’ (or whatsoever). Hence Axiom 3: Valid inferences are a necessary condition for an appropriate test of a theory. ©Thomas Plümper 2007/08 And back to research design: Axiom 1 reformulated: A good research design is one that allows making valid inferences and thus is a necessary condition for an appropriate test of a theory. ©Thomas Plümper 2007/08 Lesson 2: What is a good Research Question Axiom 4: A good research question is one that leads to a theory, which has an ex ante probability of being correct close to 0.5. ‘Correct’ here means: the theory simplifies reality in a way that leads to generalizations which help understanding many real world phenomena. ©Thomas Plümper 2007/08 Why app. 0.5? Given that research should increase (or foster) the visibility of the researcher: A theory which has a prior probability of finding empirical support close to 1.0 is trivial. A theory which has a prior probability of finding empirical support close to 0.0 is risky. Again, keep in mind that researchers test the predictions of a theory, not the assumptions. Do we know prior probabilities? Of course, just ask your colleagues whether they think your hypotheses are correct. ©Thomas Plümper 2007/08 Falsification and Falsifiability Karl Popper (1963): Theories must be falsifiable. Thus, the words ‘may’, ‘could’, ‘should’ and so on shall not be used in theories. If some action or effect is conditional, make the conditionality explicit, if it takes place only with a certain probability, make this clear. Imre Lakatos (1973): “The demarcation between science and pseudoscience is not merely a problem of armchair philosophy, it is of vital social and political relevance.” David Hume (1748): “Let us ask: does it [any volume] contain any abstract reasoning concerning quantity or numbers? (…) No. Commit it then to the flames.” ©Thomas Plümper 2007/08 Falsifiability Popper uses the term falsifiability with two different meanings: 1. Falsifiability is a logical property of statements which requires that scientific statements logically imply at least one testable prediction. 2. Falsifiability is a normative construct, telling scientists that an test of a theory should try to refute it. There is no relevant dissent with the first meaning, but the prescriptive meaning has let to huge controversies. I use the term in the first sense and will explain why ‘naïve simplification’ does not lead to scientific progress. ©Thomas Plümper 2007/08 On Naïve Falsification Thomas Kuhn and Imre Lakatos: Abandoning a theory the instant it makes false predictions would eliminate too much good research. Well, yes, but the main point is that The huge majority of (social science) theories is not deterministic but probabilistic. This implies that we cannot falsify a theory in Popper’s sense. Rather, we have to show that on average the theory’s predictions are wrong. Thomas Kuhn (1962): Actual scientists do not refute a theory simply because it makes false predictions (or even worse: one false prediction). But let’s not talk about paradigmatic change and scientific revolutions here… ©Thomas Plümper 2007/08 ‘Proving’ theories right? Hume and Popper and Lakatos and in fact about everyone agree that scientists cannot prove a theory to be right. This made Hume and Popper stress that scientists need to try to prove a theory wrong. Lakatos, however, claims that science is not a competition between theories. For Lakatos, research programs compete. Research programs can either be progressive or degenerating: progressive research programs continue to predict novel facts, degenerating research programs fabricate theories in order to accommodate known facts. But let’s repeat and keep in mind: Verification of truth is logically impossible. ©Thomas Plümper 2007/08 Can we test probabilistic theories? ©Thomas Plümper 2007/08 Can probabilistic theories be tested? Of course, but scientists need to socially agree on a certain threshold which tells us when empirical evidence ‘too much’ contradicts the probabilistic predictions derived from a theory. ©Thomas Plümper 2007/08 Probabilistic theories and verification “Verification is logically impossible.” (page 13) Yet, when studying the empirical relevance of probabilistic theories, we get a more complex result (the statement still remains correct as we will see). Let’s turn to Bayesian Philosophy of Science … Bayesians contend that confirmation is quantitative: Evidence E confirms a hypothesis H if and only if it raises the probability of H: p(H|E)>p(H) read: the probability of H (being correct) given empirical evidence E is larger than the probability of H prior to the presentation of empirical evidence E. We refer to these probabilities as prior and posterior probability. ©Thomas Plümper 2007/08 Bayes’s Theorem Axioms 1) Every probability is a real number between 0 and 1, 0≤p ( A ) ≤ 1. 2) If A is necessarily true, then p(A)=1. Note: we cannot prove p(A)=1 but we can believe it. 3) If A and B are mutually exclusive (it is not possible that statements A and B are both true), then p()()(() A∨ B = p A + p B 4) p(¬ A ) = 1 − p ( A ) 5) If A logically entails B, then p()() B≥ p A . 6) p()()()(&) A∨ B = p A + p B − p A B if A and B are mutually exclusive we get 3. Bayes’s theorem in its simplest form p( A | B )⋅ p ( B ) p( B | A ) = p() A ©Thomas Plümper 2007/08 Bayes and Advancement in Scientific Knowledge A more complex form of Bayes’s theorem: p( E| T)⋅ p( T ) p( T| E) = p( E| T)⋅ p( T) + p( E |∼ T) ⋅ p( ∼ T ) Read: The probability that a theory T is correct given some evidence E is a function of − the prior probability that T is correct, − the expectedness of evidence E, − the posterior probability of T. If a theory is deterministic, then p(E|T)=1. If a theory is probabilistic, then p(E|T)<1. ©Thomas Plümper 2007/08 Problems p(E), p(T) and p(~T)are difficult to determine. p(E) might be biased upward due to old evidence. p(~T) would be rival theories, which may or may not exist. p(T) may vary across researchers (early Bayesians suggested to derive p(T) from observed behavior). ©Thomas Plümper 2007/08 Choosing research questions according to Bayes Scientist can ‘confirm’ theories in a Bayesian world. Confirmation, however, just means that new evidence increases the (inter-) subjective probability if T.
Details
-
File Typepdf
-
Upload Time-
-
Content LanguagesEnglish
-
Upload UserAnonymous/Not logged-in
-
File Pages196 Page
-
File Size-