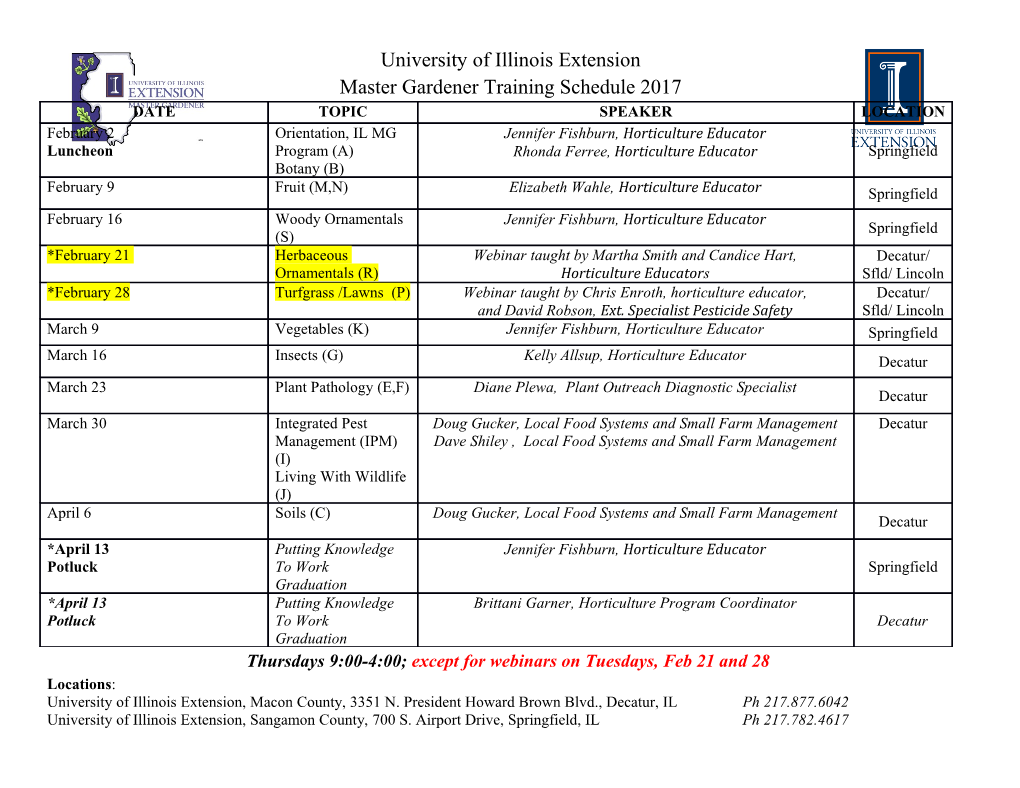
Model Counting for Logical Theories Wednesday Dmitry Chistikov Rayna Dimitrova Department of Computer Science University of Oxford, UK Max Planck Institute for Software Systems (MPI-SWS) Kaiserslautern and Saarbr¨ucken, Germany ESSLLI 2016 SAT SMT satisfiability satisfiability modulo theories #SMT #SAT model counting model counting modulo theories 2/54 Agenda Tuesday computational complexity, probability theory Wednesday randomized algorithms, Monte Carlo methods Thursday hashing-based approach to model counting Friday from discrete to continuous model counting 3/54 Outline 1. Randomized algorithms Complexity classes RP and BPP 2. Monte Carlo methods 3. Markov chain Monte Carlo 4/54 Decision problems and algorithms Decision problem: L ⊆ f0; 1g∗ (encodings of yes-instances) Algorithm for L: says \yes" on every x 2 L, \no" on every x 2 f0; 1g∗ n L 5/54 Complexity classes: brief summary P: polynomial time (efficiently solvable) NP: nondeterministic polynomial time (with efficiently verifiable solutions) #P: counting polynomial time 6/54 Examples of randomized algorithms I Primality testing I Polynomial identity testing I Undirected reachability I Volume estimation 7/54 Randomized algorithms: our model The algorithm can toss fair coins: 1. Syntactically, a randomized algorithm is an algorithm that has access to a source of randomness, but acts deterministically if the random input is fixed. 2. It has on-demand access to arbitrary many independent 1 random variables that have Bernoulli( 2 ) distribution. 3. Each request takes 1 computational step. 8/54 Deterministic and randomized time complexity Recall deterministic time complexity: I of algorithm A on input x Randomized time complexity: Maximum (worst-case) over all possible sequences of random bits Then take maximum (worst-case) over all inputs of length n. 9/54 RP: class of languages L for which there exists a randomized polynomial-time algorithm A such that x 2 L =) P[ A(x) accepts ] ≥ 1=2 x 62 L =) P[ A(x) accepts ] = 0 BPP: class of languages L for which there exists a randomized polynomial-time algorithm A such that x 2 L =) P[ A(x) accepts ] ≥ 3=4 x 62 L =) P[ A(x) accepts ] ≤ 1=4 Complexity classes P, RP, and BPP P: class of languages L for which there exists a deterministic polynomial-time algorithm A such that x 2 L =)A(x) accepts x 62 L =)A(x) rejects 10/54 BPP: class of languages L for which there exists a randomized polynomial-time algorithm A such that x 2 L =) P[ A(x) accepts ] ≥ 3=4 x 62 L =) P[ A(x) accepts ] ≤ 1=4 Complexity classes P, RP, and BPP P: class of languages L for which there exists a deterministic polynomial-time algorithm A such that x 2 L =)A(x) accepts x 62 L =)A(x) rejects RP: class of languages L for which there exists a randomized polynomial-time algorithm A such that x 2 L =) P[ A(x) accepts ] ≥ 1=2 x 62 L =) P[ A(x) accepts ] = 0 10/54 Complexity classes P, RP, and BPP P: class of languages L for which there exists a deterministic polynomial-time algorithm A such that x 2 L =)A(x) accepts x 62 L =)A(x) rejects RP: class of languages L for which there exists a randomized polynomial-time algorithm A such that x 2 L =) P[ A(x) accepts ] ≥ 1=2 x 62 L =) P[ A(x) accepts ] = 0 BPP: class of languages L for which there exists a randomized polynomial-time algorithm A such that x 2 L =) P[ A(x) accepts ] ≥ 3=4 x 62 L =) P[ A(x) accepts ] ≤ 1=4 10/54 Complexity classes P, RP, and BPP Intuition: P: deterministic polynomial time RP: randomized polynomial time with one-sided error BPP: randomized polynomial time with bounded two-sided error 11/54 Complexity classes P, RP, and BPP P: class of languages L for which there exists a deterministic polynomial-time algorithm A such that x 2 L =)A(x) accepts x 62 L =)A(x) rejects RP: class of languages L for which there exists a randomized polynomial-time algorithm A such that x 2 L =) P[ A(x) accepts ] ≥ 1=2 x 62 L =) P[ A(x) accepts ] = 0 BPP: class of languages L for which there exists a randomized polynomial-time algorithm A such that x 2 L =) P[ A(x) accepts ] ≥ 3=4 x 62 L =) P[ A(x) accepts ] ≤ 1=4 12/54 Hence, RP ⊆ NP. Definition of RP via certificates Recall: L 2 NP () there exist a polynomial p(n) and a polynomial-time algorithm V (x; y) such that the following holds: x 2 L =) there exists a y 2 f0; 1gp(jxj) such that V (x; y) = YES x 62 L =) there is no such y 2 f0; 1gp(jxj) L 2 RP () there exists a polynomial p(n) and a polynomial-time algorithm V (x; y) such that the following holds: 1 p(jxj) x 2 L =) for at least 2 -fraction of all y 2 f0; 1g it holds that V (x; y) = YES x 62 L =) there is no such y 2 f0; 1gp(jxj) 13/54 Definition of RP via certificates Recall: L 2 NP () there exist a polynomial p(n) and a polynomial-time algorithm V (x; y) such that the following holds: x 2 L =) there exists a y 2 f0; 1gp(jxj) such that V (x; y) = YES x 62 L =) there is no such y 2 f0; 1gp(jxj) L 2 RP () there exists a polynomial p(n) and a polynomial-time algorithm V (x; y) such that the following holds: 1 p(jxj) x 2 L =) for at least 2 -fraction of all y 2 f0; 1g it holds that V (x; y) = YES x 62 L =) there is no such y 2 f0; 1gp(jxj) Hence, RP ⊆ NP. 13/54 Definition of BPP via certificates L 2 RP () there exists a polynomial p(n) and a polynomial-time algorithm V (x; y) such that the following holds: 1 p(jxj) x 2 L =) for at least 2 -fraction of all y 2 f0; 1g it holds that V (x; y) = YES x 62 L =) there is no such y 2 f0; 1gp(jxj) L 2 BPP () there exists a polynomial p(n) and a polynomial-time algorithm V (x; y) such that the following holds: 3 p(jxj) x 2 L =) for at least 4 -fraction of all y 2 f0; 1g it holds that V (x; y) = YES 1 p(jxj) x 62 L =) for at most 4 -fraction of all y 2 f0; 1g it holds that that V (x; y) = YES 14/54 Error reduction (confidence amplification) for RP RP: class of languages L for which there exists a randomized polynomial-time algorithm A such that x 2 L =) P[ A(x) accepts ] ≥ 1=2 x 62 L =) P[ A(x) accepts ] = 0 Error reduction: −nd I Can replace 1=2 above with 1 − 2 for any d ≥ 1. d I Can replace 1=2 above with 1=n for any d ≥ 1. 15/54 Error reduction (confidence amplification) for BPP BPP: class of languages L for which there exists a randomized polynomial-time algorithm A such that 1 x 2 L =) P[ A(x) accepts ] ≥ 1 − 4 1 x 62 L =) P[ A(x) accepts ] ≤ 4 Error reduction: 1 −nd I Can replace above with 2 for any d ≥ 1. 4 1 1 d I Can replace above with − 1=n for any d ≥ 1. 4 2 16/54 Complexity classes P, RP, and BPP P: class of languages L for which there exists a deterministic polynomial-time algorithm A such that x 2 L =)A(x) accepts x 62 L =)A(x) rejects RP: class of languages L for which there exists a randomized polynomial-time algorithm A such that x 2 L =) P[ A(x) accepts ] ≥ 1=2 x 62 L =) P[ A(x) accepts ] = 0 BPP: class of languages L for which there exists a randomized polynomial-time algorithm A such that x 2 L =) P[ A(x) accepts ] ≥ 3=4 x 62 L =) P[ A(x) accepts ] ≤ 1=4 17/54 Complexity classes: summary P: deterministic polynomial time RP: randomized polynomial time with one-sided error BPP: randomized polynomial time with bounded two-sided error NP: nondeterministic polynomial time (with efficiently verifiable solutions) #P: counting polynomial time 18/54 Outline 1. Randomized algorithms Complexity classes RP and BPP 2. Monte Carlo methods 3. Markov chain Monte Carlo 19/54 Suppose ' ⊆ Dk, and assume µ(D) < 1. Let F beJ theK σ-algebra of measurable subsets of Dk. Then P: F! [0; 1] given by µ(A) P(A) = µ(Dk) is a probability measure on Dk. Then mc(') = P( ' ) · µ(Dk). J K Model counting and probability Recall: The model count of a formula '(x1; : : : ; xk) is mc(') = µ( ' ). J K A logical theory T is measured if every ' is measurable. J K 20/54 Then mc(') = P( ' ) · µ(Dk). J K Model counting and probability Recall: The model count of a formula '(x1; : : : ; xk) is mc(') = µ( ' ). J K A logical theory T is measured if every ' is measurable. J K Suppose ' ⊆ Dk, and assume µ(D) < 1. Let F beJ theK σ-algebra of measurable subsets of Dk. Then P: F! [0; 1] given by µ(A) P(A) = µ(Dk) is a probability measure on Dk. 20/54 Model counting and probability Recall: The model count of a formula '(x1; : : : ; xk) is mc(') = µ( ' ). J K A logical theory T is measured if every ' is measurable. J K Suppose ' ⊆ Dk, and assume µ(D) < 1.
Details
-
File Typepdf
-
Upload Time-
-
Content LanguagesEnglish
-
Upload UserAnonymous/Not logged-in
-
File Pages73 Page
-
File Size-