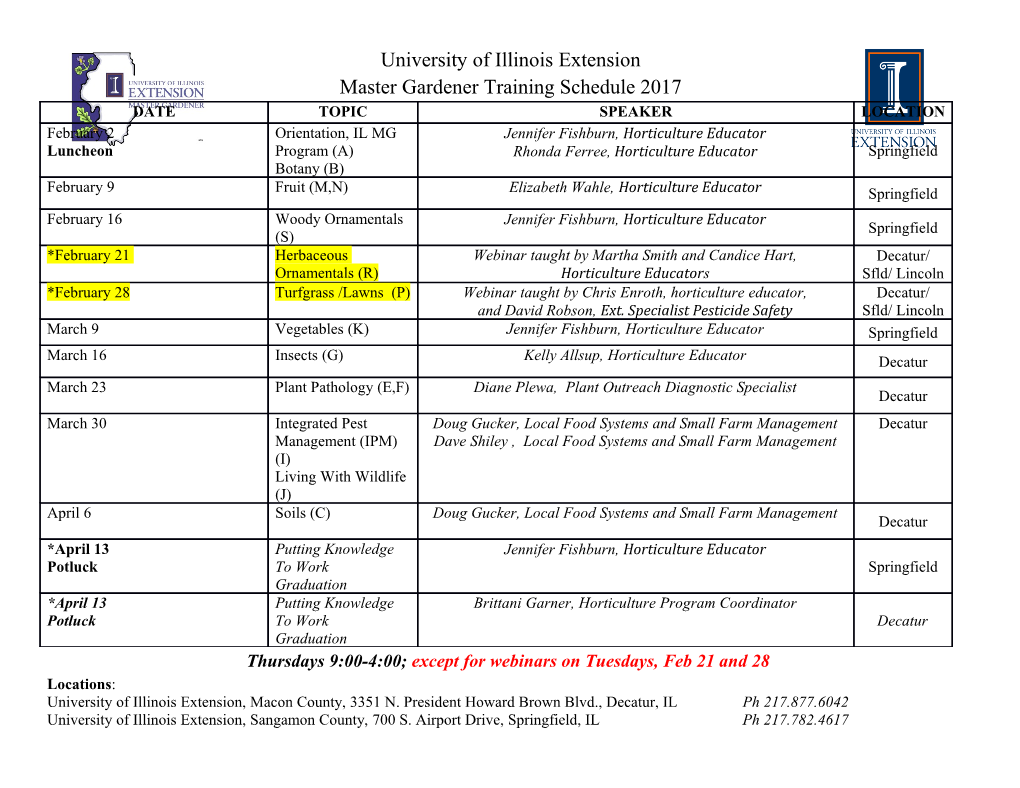
Frontier of Environmental Science December 2015, Volume 4, Issue 4, PP.91‐98 Surface Water Quality Assessment Using Multivariate Statistical Techniques: Case Study of Songhua River Basin, China Liyan Zheng 1, 2, Hongbing Yu 1†, Jianan Wang 2, Zhe Wang 2 1. College of Environmental Science and Engineering, Nankai University, Tianjin 300071, China 2. Department of Environmental Science and Engineering, Nankai University Binhai College, Tianjin 300270, China †Email: [email protected] Abstract Multivariate statistical techniques, such as principal component analysis (PCA), factor analysis (FA) and cluster analysis (CA), were applied to evaluating and interpreting the surface water quality datasets of the Songhua River Basin (SRB) in China, obtained during two years (2012-2013) of monitoring of 13 physicochemical parameters at 29 different sites. PCA assisted to recognize the factors or origins responsible for surface water quality variations and identified three latent factors and explained 83.79% of the total variance, standing for organic pollution, metal pollution and oil pollution, respectively. FA revealed that the SRB water chemistry was strongly affected by the discharge of industrial, agricultural and municipal sewage water, mining operations and petroleum exploitation. Hierarchical CA grouped 29 different sampling sites into three groups, i.e., relatively less polluted (LP), moderately polluted (MP) and highly polluted (HP) sites, based on the similarity of water quality characteristics. This study illustrates the usefulness of multivariate statistical techniques for the analysis and interpretation of huge and complex data sets, identification of pollution sources and better understanding variations in water quality for effective surface water management. Keywords: Songhua River Basin; Water quality; PCA; FA; CA 1 INTRODUCTION With increased understanding of the importance of drinking water quality to public health and raw water quality to aquatic life, there is a great need to assess water quality [1]. One of such critical efforts is the development of the surface water monitoring network [2]. However, long-term monitoring programs require monitoring of a wide range of physical, chemical and biological variables from many monitoring stations. This results in large and complex data sets that are often hard to explain and draw meaningful conclusions [3]. Further, in the surface water quality assessment, it is frequent to determine whether a variation in the concentration of measured parameters should be attributed to anthropogenic activities or to natural changes [4,5]. The problems of data reduction and interpretation, characteristic change in water quality parameters, and indicator parameter identification can be approached through the use of multivariate statistical techniques, such as principal component analysis (PCA), factor analysis (FA) and cluster analysis (CA) [2,3,5-8]. Many studies have applied PCA/FA and CA to river water and coastal water quality data: for example the Gomti River in India [5]; the Pisuerga River in Spain [6]; the Suquia River in Argentina [9]; the Mahanadi River and estuary in India [10]; and the Fuji River in Japan [11]. In this study, the Songhua River Basin in China was chosen for water quality assessment. We mainly used correlation analysis that conducted to evaluate the relationship of water quality parameters, PCA/FA to find the most important factors which describe the natural and anthropogenic influences and CA to identify several zones with different water quality. The overall aim of the present study is to provide useful information for water resources - 91 - http://www.ivypub.org/fes management at the watershed scale. 2 MATERIALS AND METHODS 2.1 Study Area The Songhua River Basin (SRB) is the third biggest basin in China, with a drainage area of 556800 km2, which includes multiple tributaries. The Basin lies between longitude of 119º52’ and 132º31’E and latitude of 41º40’ and 51º38’N. The location of the SRB is shown in FIG. 1. FIG. 1 MAP OF THE STUDY AREA AND SURFACE WATER QUALITY SAMPLING SITES IN THE SRB, CHINA. 2.2 Data According to characteristics of environmental hydromechanics and land use types, we selected 29 sampling sites located in the SRB (FIG. 1) and 13 representative parameters (TABLE 1). Selected sites were sampled every month for two years (2012-2013) following the Chinese National Standards for Scientific Sampling (Ministry of Environmental Protection of China, 2002). TABLE 1 THE WATER QUALITY PARAMETERS ASSOCIATED WITH THEIR ABBREVIATIONS AND UNITS USED IN THIS STUDY Variable Abbreviation Units pH pH pH units Dissolved oxygen DO mg/l Permanganate index CODMn mg/l Chemical oxygen demand COD mg/l 5-day biological oxygen demand BOD5 mg/l Ammonia nitrogen NH3-N mg/l Total nitrogen TN mg/l Total phosphorus TP mg/l Lead Pb mg/l Mercury Hg mg/l Zinc Zn mg/l Volatile Phenols VPhs mg/l Petroleum hydrocarbon Oil mg/l - 92 - http://www.ivypub.org/fes 2.3 Principal Component Analysis (PCA)/Factor Analysis (FA) PCA starts with the covariance matrix describing the dispersion of the original variables, and extracting the eigenvalues and eigenvectors. An eigenvector is a list of coefficients by which we multiply the original correlated variables to obtain new uncorrelated variables, called principal components (PCs), which are weighted linear combinations of the original variables [6,12]. Eigenvalues of 1.0 or greater are considered significant [11]. FA follows PCA. FA can be achieved by rotating the axis defined by PCA, and constructing new groups of variables, also called varifactors (VFs) [11]. Classification of factor loadings is “strong”, “moderate” and “weak”, corresponding to absolute loading values of >0.75, 0.75-0.50 and 0.50-0.30, respectively [13]. 2.4 Cluster Analysis (CA) The purpose of CA is to partition a set of objects into two or more groups based upon the similarity of the objects with respect to a chosen set of characteristics, so that similar objects are in the same class [14]. Hierarchical cluster analysis (HCA) was presented on the normalized data set using the Ward’s method as agglomeration technique and squared Euclidean distance as a measure of similarity. The Ward’s method yields the most meaningful clusters and has been proven to be an extremely powerful grouping mechanism. The Euclidean distance is reported as Dlink/Dmax, which represents the quotient between the linkage distance divided by the maximal distance [15]. 3 RESULTS AND DISCUSSION 3.1 Descriptive Statistics Statistical summary of the 13 measured variables in the river water samples from 29 sites in the SRB is presented in TABLE 2. Coefficient variation (CV) showed that all the variables except pH fluctuate significantly along the river basin. According to the Grade III standard under Environmental Quality Standards for Surface Water (GB 3838- 2002), it must be emphasized that average concentrations of some variable such as DO, COD, CODMn, BOD, NH3-N, TN and TP are higher than the standards, therefore the water resource is not adequate for human consumption or industrial purposes and needs to be purified. TABLE 2 STATISTICAL DESCRIPTIVE OF WATER QUALITY IN THE SRB, CHINA Variable Min Max Mean Std. Dev. CV(%) pH 6.59 8.47 7.20 0.34 4.7 DO 2.95 11.28 7.85 3.12 39.8 CODMn 1.73 20.9 6.17 3.23 52.4 BOD5 1.03 26.67 5.01 6.01 119.9 NH3-N 0.15 8.87 1.61 2.32 144.1 COD 11.09 89.40 25.35 20.73 81.8 TN 0.61 21.33 3.55 4.22 118.9 TP 0.03 3.40 0.30 0.65 216.7 Hg 0.00 0.00009 0.00002 0.00002 100.0 Pb 0.00 0.005 0.0020 0.0015 75.0 Zn 0.00 0.116 0.035 0.03 85.1 Oil 0.001 0.430 0.080 0.11 137.5 VPhs 0.0007 0.0431 0.0042 0.01 238.1 - 93 - http://www.ivypub.org/fes 3.2 Correlation of Water Quality Parameters Data in TABLE 3 provides the Pearson’s correlation matrix of the water quality parameters obtained from PCA. According to the results of correlation matrices, some clear hydro-chemical relationships can be readily inferred: TABLE 3 CORRELATION MATRIX OF THE 13 PHYSICOCHEMICAL PARAMETERS DETERMINED pH DO CODMn BOD5 NH3-N Oil VPhs Hg Pb COD TN TP Zn pH 1.00 DO 0.67 1.00 CODMn -0.59 -0.89 1.00 BOD5 -0.32 -0.78 0.79 1.00 NH3-N -0.60 -0.83 0.94 0.85 1.00 Oil -0.05 -0.30 0.37 0.64 0.56 1.00 VPhs -0.21 -0.59 0.32 0.49 0.40 0.34 1.00 Hg -0.54 -0.33 0.17 0.33 0.18 0.01 0.43 1.00 Pb -0.22 -0.31 0.21 0.49 0.21 0.36 0.49 0.55 1.00 COD -0.45 -0.85 0.78 0.94 0.83 0.62 0.71 0.47 0.53 1.00 TN -0.41 -0.68 0.83 0.73 0.95 0.59 0.34 0.02 0.02 0.74 1.00 TP -0.17 -0.34 0.56 0.53 0.70 0.79 0.10 -0.14 -0.12 0.50 0.85 1.00 Zn 0.05 -0.05 -0.03 0.25 -0.01 0.24 0.60 0.57 0.51 0.37 -0.08 -0.18 1.00 The river water pH value had relatively weak to fair correlations, i.e., most of the correlation coefficients are less than 0.7 (absolute value) with other parameters. There was a positive correlation between pH and DO (0.67); and negative correlations existed between pH and CODMn (-0.59), pH and NH3-N (-0.60), pH and Hg (-0.54).
Details
-
File Typepdf
-
Upload Time-
-
Content LanguagesEnglish
-
Upload UserAnonymous/Not logged-in
-
File Pages8 Page
-
File Size-