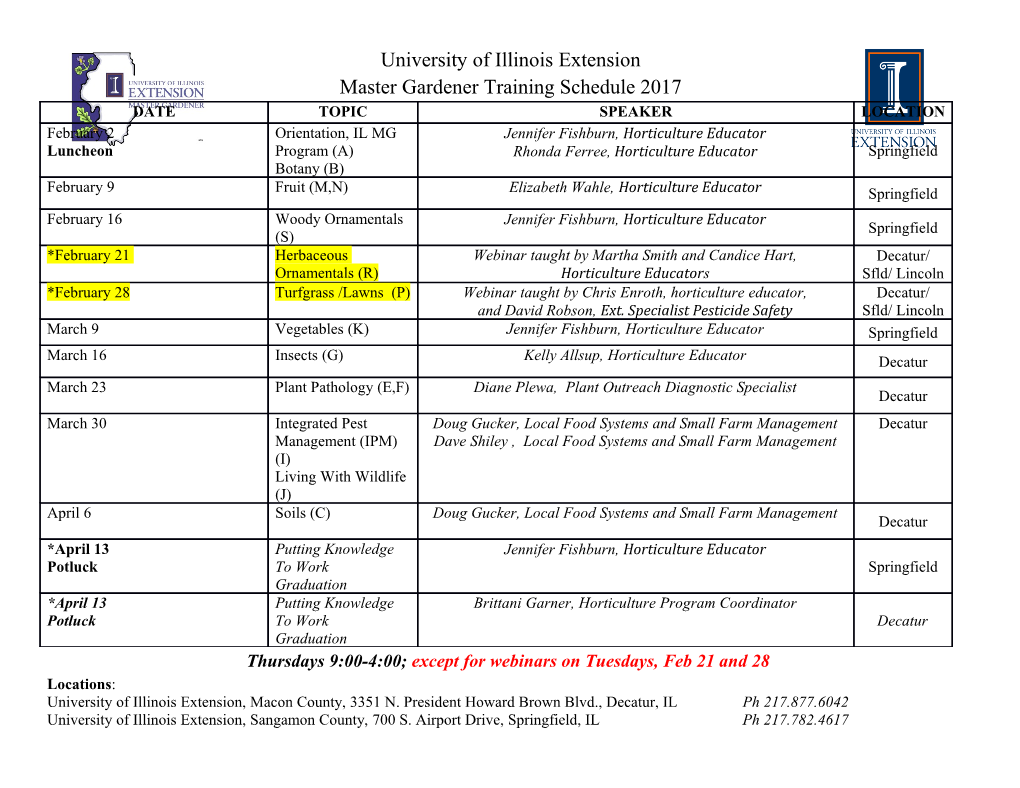
Transfer Learning Approach for Occupancy Prediction in Smart Buildings Mohamad Khalil Stephen McGough Zoya Pourmirza School of Engineering School of Computing School of Engineering Newcastle University Newcastle University Newcastle University Newcastle, Uk Newcastle, UK Newcastle, UK [email protected] [email protected] [email protected] Mehdi Pazhoohesh Sara Walker Faculty of Technology School of Engineering De Montfort University Newcastle University Leicester, UK Newcastle, UK [email protected] [email protected] Abstract— Accurate occupancy prediction in smart I. INTRODUCTION buildings is a key element to reduce building energy consumption and control HVAC systems (Heating – Ventilation In buildings the term of occupancy information refers to and– Air Conditioning) efficiently, resulting in an increment of occupants’ presence or absence and their movements. human comfort. This work focuses on the problem of occupancy Accurate real-time occupancy information in smart prediction modelling (occupied / unoccupied) in smart buildings buildings can help HVAC (Heating – Ventilation and– Air using environmental sensor data. A novel transfer learning Conditioning) control systems to optimize their usage and approach was used to enhance occupancy prediction accuracy minimize building energy consumption [1]. In the past years, when the amounts of historical training data are limited. The occupancy prediction modelling in buildings has been studied proposed approach and models are applied to a case study of by many researchers using different types of data such as air three office rooms in an educational building. The data sets used temperature, sound, door status, relative humidity, camera, in this work are actual data collected from the Urban Sciences motion and light. Building (USB) in Newcastle University. The results of the Recently, Machine Learning (ML) and Deep Learning proposed transfer learning approach have been compared with (DL) models are becoming more popular in the applications the models from Support Vector Machine and Random Forest of building energy such as forecasting building energy algorithms. The final results demonstrate that the most accurate consumption and occupancy prediction [2,3]. model in this study to predict occupancy status was produced by The present work focuses on the challenging task of stacked Long-Short-Term-Memory with a transfer learning framework. occupancy prediction modelling (occupied / unoccupied) when the amounts of historical training data are limited by Keywords—(Occupancy Prediction, Machine Learning, using a transfer learning framework. Transfer Learning, Deep Learning, Smart Buildings) The rest of this paper is organized as follows: Section II, introduces a brief summary on ML and DL applications in Nomenclature related works. Section III, discusses data collection and data ML Machine learning pre-processing steps in this paper. Section IV, introduces the DL Deep learning proposed approach and algorithms. Section V, shows the SVM Support vector machine results. Finally, Section VI, presents the conclusion. RF Random forest II. RELATED WORK LSTM Long-Short-Term-Memory DT Decision trees Occupancy prediction modelling (occupied / unoccupied) TL Transfer learning can be categorized as a classification task, which means that HVAC Heating, ventilation and air conditioning each row in a data set assigns to a class. MLP Multi-Layer Perceptron Recently, researchers have used different approaches of BPN Back-Propagation Network ML and DL to predict occupancy status (absence / presence). LDA Linear discriminant analysis The reviewed literature has been categorized and presented CNN Convolutional neural network in Table I based on the following: year, classification KNN K-Nearest Neighbour algorithms, buildings type, type of features used, size of AMI Advanced metering infrastructure historical data and reported accuracy. CART Classification and Regression Trees Occupancy prediction (occupied / unoccupied) in a PIR Passive infrared sensor domestic environment was presented by Vafeiadis et al., 2021 12th International Renewable Engineering Conference (IREC) | 978-1-6654-2548-3/20/$31.00 ©2021 IEEE DOI: 10.1109/IREC51415.2021.9427869 ReLu Rectified linear unit (2017) in [4]. The authors used decision trees (DT), support D Domain vector machine (SVM), back-propagation network (BPN), X Feature space and random forest (RF), along with the AdaBoost algorithm (X) Marginal probability distribution to predict occupancy status. These ML models used data from T Task water and energy consumption. It was found that RF and DT Y Label space outperformed other classification techniques with respect to F ( ) Objective predictive function the accuracy. 978-1-6654-2548-3/21/$31.00 ©2021 IEEE Authorized licensed use limited to: De Montfort University. Downloaded on May 17,2021 at 10:24:53 UTC from IEEE Xplore. Restrictions apply. Three statistical classification algorithms which are linear accuracy when the amounts of historical training data are discriminant analysis (LDA), classification and regression limited. trees (CART) and RF were used by Candanedo and Feldheim Transfer learning is a subset of ML. The main idea of (2016) in [1] to predict occupancy in an office environment. transfer learning is that the knowledge that has already been Their results have shown that appropriate selection of learned from a related source domain can be transferred to features can significantly improve the prediction improve model performance in a target domain [10]. Gao, performance. Ruan, Fang, and Yin (2020) in [11] utilized two DL models Sensor data from light, carbon dioxide (CO 2), passive which are a sequence-to-sequence and a two-dimensional infrared motion (PIR), sound and electrical current were used convolutional neural network with transfer learning to predict to train a decision tree model by Hailemariam , Goldstein, building energy consumption. Their results indicate that Attar, and Khan (2011) [5]. transfer learning could improve the prediction accuracy. Wang, Chen, and Hong (2018) in [6] used the following The objective of this study is to determine whether ML models: k-nearest neighbor (KNN), SVM and BPN. transfer learning approach could improve the occupancy These models were trained with environmental and Wi-Fi prediction performance of target rooms with limited data by data to classify occupancy pattern. It was found that fused transferring the knowledge learned from a similar room with data sets can improve the reliability of these techniques. enough historical data. A deep neural network to predict occupancy status was presented by Abedia and Jazizadehb (2019) in [7], and the III. CASE STUDY data used in this study were collected from two cost-effective The Urban Science Building (USB) studied in this paper sensors: doppler radar sensors (DRS) and infrared thermal is a university educational building situated in City-Of- array (ITA). Newcastle in the United Kingdom, Fig. 1 provides a picture Feng, Mehmani, and Zhang (2020) in [8] used advanced showing the building. USB is one of the largest repositories of metering infrastructure (AMI) data to train a DL model which publicly held data in the UK. This building has six floors and consists of convolutional neural network (CNN) and it mainly comprises of office and teaching rooms. The office bidirectional LSTM. The average reported accuracy of this hours of this building approximately run from 08:00 to 18:00 model was around 90%. every weekday. Three office rooms (A, B and C) within the A study of real-time occupancy detection in an office third and fourth floors were selected in our experiments. These rooms run a similar business schedule throughout the environment using CO data was presented by Zhou. et al., 2 year. The rooms differ in the size of the area covered (room (2019) in [9]. The ML model used in this study was gcForest. A: 31 m 2, room B: 30 m 2, room C: 15m 2 ). However, according to these studies ML and particularly DL models rely on large amounts of historical training data to provide satisfactory prediction and forecasting accuracy, A. Data collection but sometimes this data are difficult to collect or very expensive. In fact, a group of newly monitored buildings do This paper used data collected from environmental sensors not have enough amounts of historical observations and such as temperature, relative humidity and CO 2 , in addition to motion sensor. This use of environmental sensor data can measurements to predict occupancy status accurately. In light avoid privacy concerns of using internal cameras for real-time of this challenge, a novel transfer learning approach for occupancy detection. occupancy prediction modelling (occupied / unoccupied) in an office environment is presented to obtain a desirable Table I. Comparison of the reviewed studies Reference Year Classification Environment Data type Size of Accuracy No algorithms used type historical data [1] 2016 LDA, CART, RF Office Environmental: temp, humidity, 1 month Ranging from light, CO 2, humidity ratio 32.68% to 99.31% [4] 2017 SVM, RF, DT, BPN, Domestic Smart meter: Lights, refrigerator, 1 month Ranging from AdaBoost TV, Washing Machine, Dryer, 75.87% to 80.53% Cold Water – Kitchen, Hot Water Kitchen, Dishwasher - Water, Washing Machine - Water [5] 2011 DT Office Environmental: light, CO 2, PIR, Seven day Ranging from electrical current, sound 81.01% to 98.44% [6] 2018 BPN, KNN, SVM Office Environmental (indoor air Nine days NA temperature,
Details
-
File Typepdf
-
Upload Time-
-
Content LanguagesEnglish
-
Upload UserAnonymous/Not logged-in
-
File Pages6 Page
-
File Size-