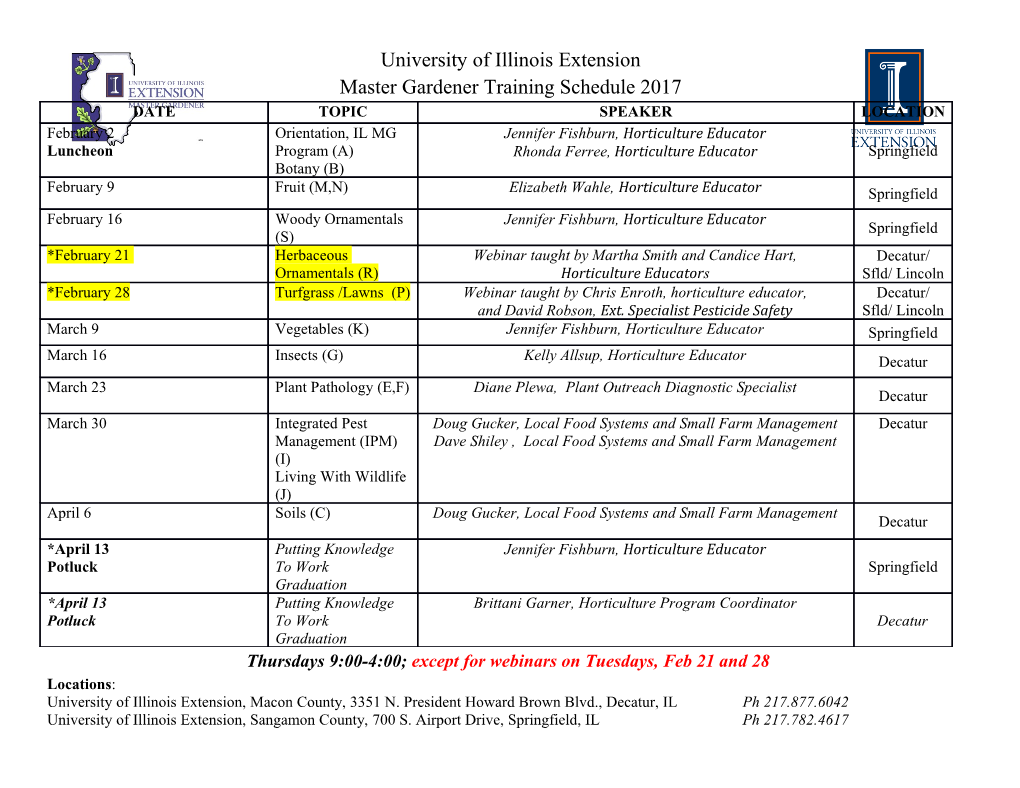
ESSAYS ON TECHNOLOGY-DRIVEN MARKETING Zijun (June) Shi Submitted to the Tepper School of Business in partial fulfillment of the requirements for the degree of DOCTOR OF PHILOSOPHY Dissertation Committee Kannan Srinivasan (Chair) Hui Li Xiao Liu Alan Montgomery Kaifu Zhang April, 2019 I © Coypyright by Zijun (June) Shi, 2019 All rights reserved. II Abstract: With the development of technology in business applications, new marketing problems emerge, creating challenges for both practitioners and researchers. In this dissertation, I investigate marketing issues that involve new technology or require research methodologies enabled by new technology. I take an interdisciplinary approach, combining structural modeling, analytical modeling, machine learning, and causal inference, to study problems on pricing, media hype, and branding in three essays. In the first essay, we examine the optimality of the freemium pricing strategy. Despite its immense popularity, the freemium business model remains a complex strategy to master and often a topic of heated debate. Adopting a generalized version of the screening framework à la Mussa and Rosen (1978), we ask when and why a firm should endogenously offer a zero price on its low-end product when users' product usages generate network externalities on each other. Our analysis indicates freemium can only emerge if the high- and low-end products provide asymmetric marginal network effects. In other words, the firm would set a zero price for its low- end product only if the high-end product provided larger utility gain from an expansion of the firm's user base. In contrast to conventional beliefs, a firm pursuing the freemium strategy might increase the baseline quality on its low-end product above the “efficient” level, which seemingly reduces differentiation. In the second essay, we study how misleading health information affects subsequent information generation from different sources, by examining publicly available health information about over-the-counter (OTC) weight loss products. We find that biased health information from celebrity doctors may initiate a media-hype, creating news waves on the recommended healthcare products. By analyzing the news content using natural language processing techniques, we find that the news articles generated after The Dr. Oz Show carry higher sentiment and more positive emotion, and they provide little correction for the hype news. While the government has been fighting against fake news in the healthcare domain, our finding suggests that even legitimate and genuine news articles respond to the hype news by propagating and magnifying the hype news, rather than correcting them. Research articles either react too slowly or do not respond to biased information. Though some user-generated content (UGC) provides correction, it is overwhelmed by the larger amount of UGC that supports the misleading information. Lastly, public information remained biased until the Federal Trade Commission hearing and investigation. Our findings have public policy implications on how to potentially regulate media content in the healthcare domain to protect consumers from misleading health information. In the third essay, we develop a dynamic structural model of fashion choices of brands and styles to investigate the implication of prohibiting fast fashion copycats (e.g., Zara, Forever 21), with the help of user-generated data from fashion-specific social media and deep learning methods on image analytics. We find that copycats can enhance high-end brands demand, contrary to III conventional wisdom, due to several novel mechanisms: first, the affordability of mixing low- end copycats with high-end brands boosts demand for high-end brands from financially constrained consumers; second, good styles from low-end brands can help a consumer to build up his popularity/likeability, which increases his value for high-end brands and reduces the cost. Substantively, our results shed light on copyright enforcement and have implications on how fashion brands should react to copycats. Methodically, we developed a framework to analyze consumer choices where visual features are important product attributes and peer feedback hugely affects the decision-making process. IV Acknowledgment: I am deeply indebted to the support and guidance of my dissertation committee members: Professor Kannan Srinivasan, Professor Kaifu Zhang, Professor Xiao Liu, Professor Alan Montgomery, and Professor Hui Li. Since my first sememster in Ph.D. program, I was constantly enlightened by the discussion with Professor Kannan Srinivasan. Throughout the writing of this dissertation, I have received a great deal of support and utmost encouragement from him. Professor Kaifu Zhang guided me on conducting analytical research in Marketing, with insightful direction and invaluable advice. His expertise guided me especially on formulating the research topic and methodology. Professor Xiao Liu provided me with unreserved support since I moved on to the empirical research domain. I benefited a lot from every discussion with her. I also want to express my gratitude to Professor Alan Montgomery and Professor Hui Li. Their expertise and knowledge make the process of developing this dissertation rewarding and exciting. I would also like to thank Professor Param V. Singh. His invaluable advice and support are tremendously helpful for my research and the job market. I learned not only research skills but also precious experience from him. I would also like to thank other professors: Dokyun Lee, Christopher Y. Olivola, Peter Boatwright, Jeff Galak, and Tong (Joy) Lu, for taking the time and effort discussing research ideas and giving advice. Their support makes the past five years a more rewarding and pleasant journey. I thank my husband Siyu Lu. This dissertation would not have been possible without his endless support, unreserved love, and continued patience. I would also like to express my deepest gratitude to our parents for their love and support. Lastly, I thank my daughter, Yutong Thea Lu, for the great joy she brought to my life and for the distraction she injected into my work time. V For my dearest family VI Contents Chapter 1 Freemium as an Optimal Strategy for Market Dominant Firms ................................. 1 1.1 Introduction ........................................................................................................................ 1 1.2 Literature Review ............................................................................................................... 6 1.3 Model ................................................................................................................................. 8 1.4 Analysis............................................................................................................................ 13 1.5 Endogenous quality decisions .......................................................................................... 21 1.5.1 Endogenous quality ................................................................................................... 22 1.5.2 Endogenous quality and network effect .................................................................... 23 1.6 Concluding Remarks ........................................................................................................ 29 References ..................................................................................................................................... 33 Appendix A: Proofs. ................................................................................................................. 37 Appendix B ............................................................................................................................... 54 Chapter 2 Hype News May Drive Real News: The Oz Effect in Healthcare ............................ 58 2.1 Introduction ...................................................................................................................... 58 2.2 Literature review .............................................................................................................. 61 2.3 Data .................................................................................................................................. 62 2.3.1 Amazon Product Data ............................................................................................... 63 2.3.2 Products Included in Analysis .................................................................................. 64 2.3.3 Multi-media Data and Articles .................................................................................. 65 2.4 Methodology .................................................................................................................... 68 2.4.1 Textual Features to Extract ....................................................................................... 68 2.4.2 Informational Content Extraction with CNN ............................................................ 70 2.4.3 Language Features Extraction Leveraging NLP Model ........................................... 73 2.4.4 Econometric Model ................................................................................................... 76 2.4.5 Endogeneity .............................................................................................................. 78 2.5 Results .............................................................................................................................. 79 2.5.1 Model
Details
-
File Typepdf
-
Upload Time-
-
Content LanguagesEnglish
-
Upload UserAnonymous/Not logged-in
-
File Pages154 Page
-
File Size-