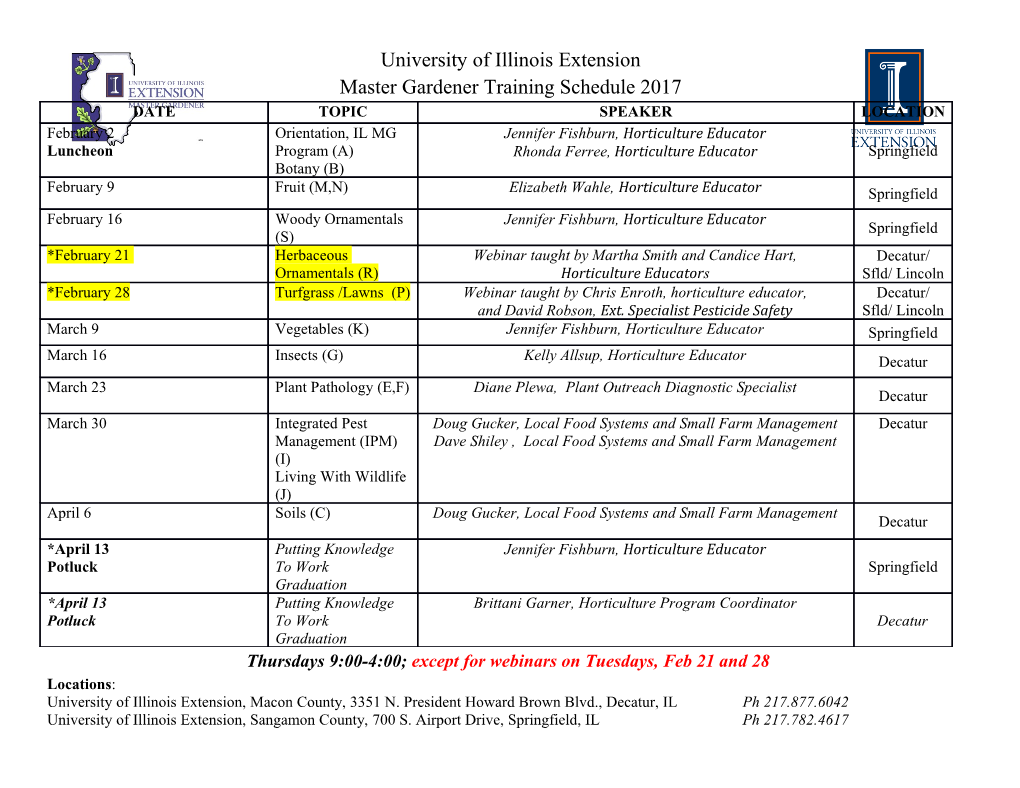
FAKULTAT¨ FUR¨ MATHEMATIK UNIVERSITAT¨ KARLSRUHE Optimisation of Clustering Algorithms for the Identification of Customer Profiles from Shopping Cart Data Optimierung von Clusterverfahren zur Identifikation von K¨auferprofilen aus Warenkorbdaten Diplomarbeit von: Stefanie Nagel Matrikel Nummer: 1190189 Karlsruhe, October 17, 2008 Ausgef¨uhrtunter der Leitung von Referentin/Betreuerin: Koreferent: Prof. Dr. Dorothea Wagner Prof. Dr. Willy D¨orfler Fakult¨atf¨urInformatik Fakult¨at f¨urMathematik Universit¨at Karlsruhe Universit¨at Karlsruhe betreuender Mitarbeiter: Robert G¨orke Fakult¨atf¨urInformatik Universit¨at Karlsruhe I Eidesstattliche Erkl¨arung Hiermit versichere ich, dass ich die vorliegende Diplomarbeit ohne Hilfe Dritter und nur mit den angegebenen Quellen und Hilfsmitteln angefertigt habe. Diese Arbeit hat in gleicher oder ¨ahnlicher Form noch keiner Pr¨ufungsbeh¨ordevorgelegen. Karlsruhe, February 25, 2009 Stefanie Nagel I Contents Eidesstattliche Erkl¨arung I Contents III List of Figures VII List of Tables IX 1 Introduction 1 2 Motivation 3 2.1 Finding Frequent Itemsets with FP-Growth . 4 2.2 Generating Association Rules . 10 2.3 Improving Association Rules . 10 2.4 Possibilities for Improving This Procedure . 11 3 Graph Modelling 13 3.1 Building the Weights . 13 3.2 Community . 14 3.3 The Number of Clusterings . 17 4 Quality Indices 19 4.1 Coverage . 19 4.2 Performance . 20 4.3 Modularity . 20 4.4 Extending Coverage . 21 4.5 Extending Performance . 21 4.6 Extending Modularity . 22 4.7 An Example . 26 5 Network Analysis 31 5.1 Visualisation of the Graph . 31 5.2 Degree Distribution . 32 5.3 Betweenness Centrality . 32 5.4 Clustering Coefficients . 34 5.5 k-Core Analysis . 36 III IV CONTENTS 6 Clustering Algorithms 41 6.1 CPM............................................. 41 6.1.1 The Idea . 41 6.1.2 Real-World Examples . 43 6.1.3 Algorithmic Implementation . 43 6.1.4 Existence of k-cliques . 45 6.1.5 The Realisation . 45 6.1.6 The Result . 45 6.1.7 Advantages and Disadvantages of the CPM . 48 6.2 CPMw............................................ 49 6.2.1 The Idea . 49 6.2.2 Clique Size and Intensity Threshold . 49 6.2.3 The Result . 50 6.2.4 Advantages and Disadvantages of the CPMw . 51 6.2.5 Comparison of CPM and CPMw . 51 6.3 CNM............................................. 52 6.3.1 The Idea . 52 6.3.2 Real-World Examples . 53 6.3.3 The Realisation . 54 6.3.4 Tuning the Efficiency . 55 6.3.5 The Result . 56 6.3.6 Advantages and Disadvantages of the CNM Algorithm . 56 6.4 Guillaume . 58 6.4.1 The Idea . 58 6.4.2 Real-World Examples . 58 6.4.3 The Realisation . 61 6.4.4 The Result . 61 6.4.5 Advantages and Disadvantages of Guillaume . 61 6.5 Fortunato . 63 6.5.1 The Idea . 63 6.5.2 Real-World Examples . 63 6.5.3 Improving the Effectiveness . 64 6.5.4 The Right Choice of α ............................... 65 6.5.5 The Computational Complexity . 67 6.5.6 The Realisation . 68 6.5.7 The Result . 68 6.5.8 Advantages and Disadvantages of Fortunato . 69 7 Comparison of the Results 71 7.1 Quality Indices Discussion . 72 7.1.1 Modularity . 72 7.1.2 Coverage . 73 7.1.3 Performance . 73 7.2 CPM and CPMw . 75 7.3 CNM............................................. 76 7.4 Guillaume . 77 7.5 Fortunato . 77 7.6 Visualisations for Gbuy ................................... 78 IV CONTENTS V 8 Transfer to Another Graph 81 8.1 Network Analysis . 81 8.1.1 Visualisation . 81 8.1.2 Degree Distribution . 81 8.1.3 Betweenness Centrality . 81 8.1.4 Clustering Coefficients . 81 8.1.5 Edge Weight Distribution . 83 8.1.6 k-Core Analysis . 83 8.2 Results . 84 8.3 Conclusion . 88 8.3.1 Using the Results . 88 8.3.2 Semantics in the Detected Clusters . 88 8.3.3 Next Steps . 88 9 Implementation Chapter 89 Appendix 93 A Visualisation of Gbuy 93 B Weight Threshold and Community Size of the CPM for Gbuy 95 C Distribution of the Community Sizes for Gbuy 97 D Distribution of the Community Sizes for Gbuy (Threshold = 0.1) 99 E Distribution of the Community Sizes for Gbuy (Threshold = 0.02) 101 F Modularity During the Application of the CNM Algorithm (Gbuy) 103 G Comment Concerning Guillaume’s Software 105 H Statistics of Fortunato’s Algorithm (Applied to Gbuy) 107 anotherShop I Visualisation of Gbuy 111 Bibliography 113 V List of Figures 2.1 Primitives for specifying a data mining task . 5 2.2 Mining an FP-tree with a single prefix path . 8 2.3 The FP-tree of the example in Table 2.1 . 9 2.4 The conditional FP-tree of vertex p in the example of Table 2.1 . 9 3.1 C1 and C3 are weak communities, C2 is not a weak community . 15 3.2 Example of an LS set . 16 4.1 Different clusterings of an example graph . 27 5.1 Degree distribution in Gbuy ................................. 33 5.2 Betweenness centrality in Gbuy ............................... 34 5.3 Clustering coefficients in Gbuy ............................... 35 5.4 Example of 0, 1, 2 and 3 core . 36 5.5 k-cores of Gbuy (LaNet-vi) . 37 5.6 k-cores of Gbuy (LunarVis) . 38 6.1 Rolling a 3-clique through a graph . 42 6.2 Local community structure in the neighbourhood of a randomly chosen vertex (CPM) 43 6.3 Edge weight distribution of Gbuy .............................. 45 6.4 Resulting community sizes of the CPM . 47 6.5 Comparison of the CPM and the CPMw . 51 6.6 Translation of a weighted network into a multigraph . 52 6.7 Dendrogram of the communities in the ”karate club” network (CNM) . 53 6.8 Dendrogram of the communities in the network of ”American college football teams” (CNM) . 54 6.9 Modularity during the application of the CNM algorithm in case of Gbuy ....... 56 6.10 Communities in the ”Belgian mobile phone” network (Guillaume) ..
Details
-
File Typepdf
-
Upload Time-
-
Content LanguagesEnglish
-
Upload UserAnonymous/Not logged-in
-
File Pages127 Page
-
File Size-