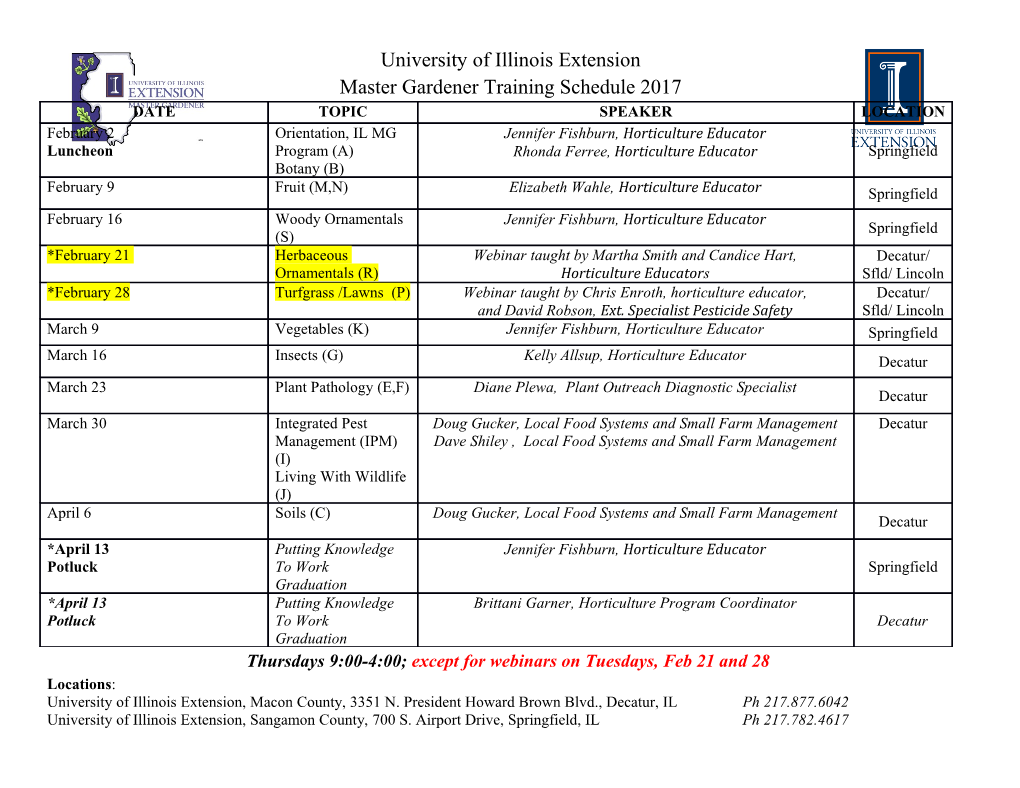
J. Hlavácovᡠ(Ed.): ITAT 2017 Proceedings, pp. 5–14 CEUR Workshop Proceedings Vol. 1885, ISSN 1613-0073, c 2017 S. Cinková, A. Vernerová Are Annotators’ Word-Sense-Disambiguation Decisions Affected by Textual Entailment between Lexicon Glosses? Silvie Cinková, Anna Vernerová Charles University, Faculty of Mathematics and Physics Institute of Formal and Applied Linguistics Malostranské námestíˇ 25 118 00 Praha 1 Czech Republic ufal.mff.cuni.cz {cinkova,vernerova}@ufal.mff.cuni.cz Abstract: We describe an annotation experiment com- verb on the one hand, and those related to the lexicograph- bining topics from lexicography and Word Sense Disam- ical design of PDEV, such as semantic relations between biguation. It involves a lexicon (Pattern Dictionary of En- implicatures within a lemma or denotative similarity of the glish Verbs, PDEV), an existing data set (VPS-GradeUp), verb arguments, on the other hand (see Section 3 for defi- and an unpublished data set (RTE in PDEV Implicatures). nitions and examples). The aim of the experiment was twofold: a pilot annota- This paper focuses on a feature related to PDEV’s de- tion of Recognizing Textual Entailment (RTE) on PDEV sign (see Section 2.3), namely on textual entailment be- implicatures (lexicon glosses) on the one hand, and, on tween implicatures in pairs of patterns of the same lemma the other hand, an analysis of the effect of Textual Entail- entry (henceforth colempats, see Section 3.1 for definition ment between lexicon glosses on annotators’ Word-Sense- and more detail). Disambiguation decisions, compared to other predictors, We pairwise compare all colempats, examining their such as finiteness of the target verb, the explicit presence scores in the graded decision annotation with respect to of its relevant arguments, and the semantic distance be- how much they compete to become the most appropriate tween corresponding syntactic arguments in two different pattern, as well as the scores of presence of textual entail- patterns (dictionary senses). ment between their implicatures. To quantify the compar- isons, we have introduced a measure of rivalry for each pair. The more the rivalry increases, the more appropri- 1 Introduction ate both colempats are considered for a given KWIC2 and A substantial proportion of verbs are perceived as highly the more similar their appropriateness scores are (see Sec- polysemous. Their senses are both difficult to determine tion 3.2). when building a lexicon entry and to distinguish in context We confirm a significant positive association between when performing Word Sense Disambiguation (WSD). To rivalry in paired colempats and textual entailment between tackle the polysemy of verbs, diverse lexicon designs and their implicatures. annotation procedures have been deployed. One alterna- tive way to classic verb senses (e.g. to blush - to redden, as 2 Related Work from embarrasment or shame1) is usage patterns coined in the Pattern Dictionary of English Verbs (PDEV) [9], which 2.1 Word Sense Disambiguation will be explained in Section 2.2. Previous studies [3], [4] have shown that PDEV represents a valuable lexical re- Word Sense Disambiguation (WSD)[18] is a traditional source for WSD, in that annotators reach good interanno- machine-learning task in NLP. It draws on the assump- tator agreement despite the semantically fine-grained mi- tion that each word can be described by a set of word crostructure of PDEV. This paper focuses on cases chal- senses in a reference lexicon and hence each occurrence lenging the interannotator agreement in WSD and consid- of a word in a given context can be assigned a word sense. ers the contribution of textual entailment (Section 2.3) to Bulks of texts have been manually annotated with word interannotator confusion. senses to provide training data. Nevertheless, the exten- We draw on a data set based on PDEV and annotated sive experience from many such projects has revealed that with graded decisions (cf. Section 2.4) to investigate fea- even humans themselves do not do particularly well in- tures suspected of blurring distinctions between the pat- terpreting word meaning in terms of lexicon senses, de- terns [1]. We have been preliminarily considering features spite specialized lexicons designed entirely for this task: related to language usage independently of the lexicon de- the English WordNet [8], PropBank [14], and OntoNotes sign, such as finiteness and argument opacity of the target 2KWIC = key word in context: a corpus line containing a match to 1http://www.dictionary.com/browse/blush a particular corpus query 6 S. Cinková, A. Vernerová Figure 1: PDEV entry with three patterns Word Senses [12], to name but a few. Although the anno- be inferred (entailed) from another text. More concretely, tators, usually language experts, have neither comprehen- the applied notion of textual entailment is defined as a sion problems nor are they unfamiliar with using lexicons, directional relationship between pairs of text expressions, their interannotator agreement has been notoriously low. denoted by T the entailing ‘text’ and by H the entailed This in turn makes the training data unreliable as well as ‘hypothesis’. We say that T entails H if, typically, a the evaluation of WSD systems harder. human reading T would infer that H is most probably Attempts have been made to increase the interannotator true”. So, for instance, the text Norway’s most famous agreement by testing each entry on annotators while de- painting, ‘The Scream’ by Edvard Munch, was recovered signing the lexicon [12], as well as word senses were clus- yesterday, almost three months after it was stolen from tered post hoc on the other hand (e.g. [17]), but even lex- an Oslo museum entails the hypothesis Edvard Munch icographers have been skeptical about lexicons with hard- painted ‘The Scream’ [5]. wired word senses for NLP([13, 15]). 2.4 Graded Decisions on Verb Usage Patterns: 2.2 Pattern Dictionary of English Verbs (PDEV) VPS-GradeUp The reasoning behind PDEV is that a verb has no meaning The VPS-GradeUp data set draws on Erk’s experiments in isolation; instead of word senses, it has a meaning po- with paraphrases (USim)[7]. VPS-GradeUp consists tential, whose diverse components and their combinations of both graded-decision and classic-WSD annotation of are activated by different contexts. To capture the mean- 29 randomly selected PDEV lemmas: seal, sail, distin- ing potential of a verb, the PDEV lexicographer manually guish, adjust, cancel, need, approve, conceive, act, pack, clusters random KWICs into a set of prototypical usage embrace, see, abolish, advance cure, plan, manage, exe- patterns, considering both their semantic and morphosyn- cute, answer, bid, point, cultivate, praise, talk, urge, last, tactic similarity. Each PDEV pattern contains a pattern hire, prescribe, and murder. Each lemma comes with definition (a finite clause template where important syn- 50 KWICs processed by three annotators3 in parallel. tactic slots are labeled with semantic types) and an impli- In the graded-decision part, the annotators judged each cature to explain or paraphrase its meaning, which also pattern for how well it described a given KWIC, on a Lik- is a finite clause (Fig. 1). The PDEV implicature corre- ert scale4. In the WSD part, each KWIC was assigned one sponds to gloss or definition in traditional dictionaries. best-matching pattern. The entire data set contains WSD The semantic types (e.g. Human, Institution, Rule, Pro- judgments on 1,450 KWICs, corresponding to 11,400 cess, State_of_Affairs) are the most typical syntactic slot graded decisions (50 sentences 29 lemmas sum of × × fillers, although the slots can also contain a set of collo- patterns). A more detailed description of VPS-GradeUp is cates (a lexical set) and semantic roles complementary to given by Baisa et al.[1]. semantic types. The semantic types come from an ap- Fig. 2 shows a VPS-GradeUp sample of three KWICs proximately 250-item shallow ontology associated with of the verb abolish (see Fig. 1 to refer to the lexicon entry). PDEV and drawing on the Brandeis Semantic Ontology Columns 1, 2, and 3 identify the pattern ID, lemma, and (BSO), [19]. The notion of semantic types, lexical sets, sentence ID, respectively. Columns 4-6 and 7-9 contain and semantic roles (altogether dubbed semlabels) is, in the graded and WSD decisions by the three annotators, this paper, particularly relevant for Section 3.5. respectively. Column 10 contains the annotated KWIC, which for Sentence 1 reads: President Mitterrand said yes- 2.3 Recognizing Textual Entailment (RTE) terday that the existence of two sovereign German states Recognizing Textual Entailment (RTE) is 3 a computational-linguistic discipline coined by Da- linguists, professional but non-native English speakers 4Likert scale is a psychometric scale used in opinion surveys. It en- gan et al. [5]. The task of RTE is to determine, “given ables the respondents to scale their agreement/disagreement with a given two text fragments, whether the meaning of one text can opinion. Are Annotators’Word-Sense-Disambiguation Decisions Affected by Textual Entailment between Lexicon Glosses? 7 ● 20 ● ●● ● ●● ●● ●● ●●● ●● 15 ●● ●●●● ●● ●●● ●●● ●● ●●● ●●●● ●●● ●●●● ●●● ●●●●● 10 ●● ●●● ●●● ●●●● ●● appropriateness ●●● ●● ●● ●● ● ●● 5 ●● ● Figure 2: A VPS-GradeUp annotation sample ● 1−1−1 1−1−3 1−2−2 2−2−2 3−3−3 4−4−4 5−5−5 6−6−6 7−7−7 possible judgment triples sorted according to appropriateness could not be ‘ABOLISHED at a stroke’. On the third ta- ble row, Pattern 3 was judged as maximally appropriate by Figure 3: Shape of the appropriateness function Annotator 1 and 2; Annotator 3 gave one point less. In the WSD part, Annotator 1 voted for Pattern 2, while Annota- tors 2 and 3 preferred Pattern 3. 3.2 Appropriateness 3 Important Concepts The appropriateness of a pattern for a given KWIC line is based on the triple of graded annotation judgments, con- flating their sum and standard deviation in this formula: 3.1 Lempats and Colempats To begin with, we introduce the concept of lempats and Appropriateness = x sd(x)/3.5 ∑ − colempats.
Details
-
File Typepdf
-
Upload Time-
-
Content LanguagesEnglish
-
Upload UserAnonymous/Not logged-in
-
File Pages10 Page
-
File Size-