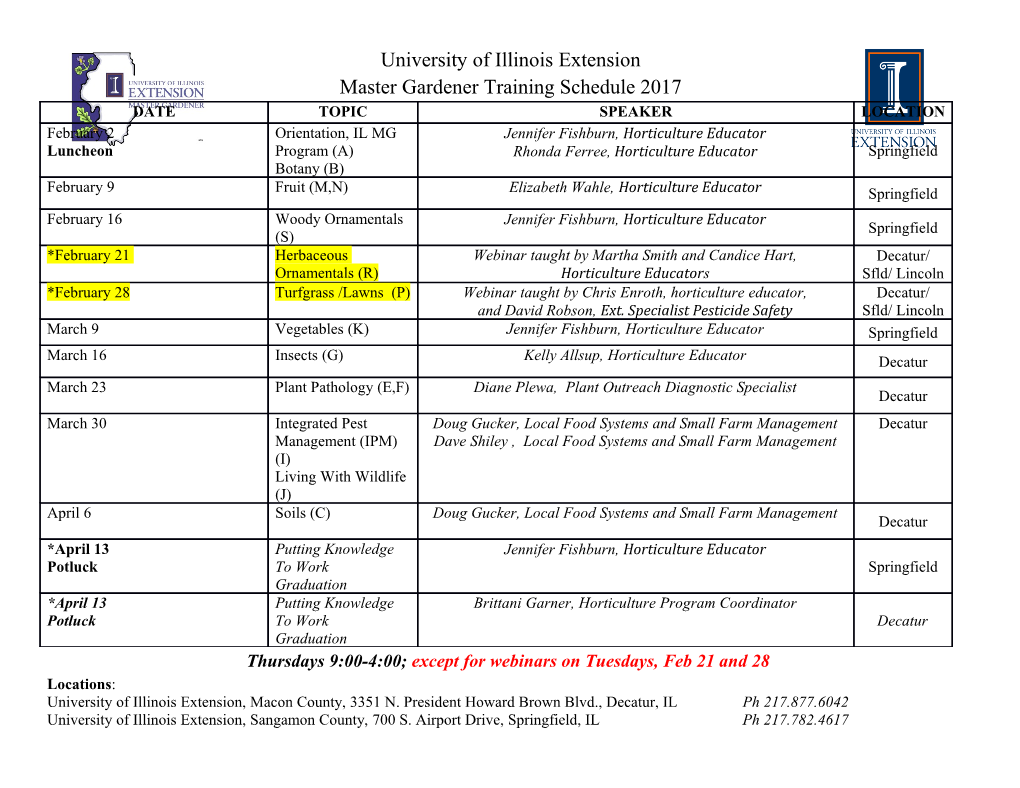
RS – Lecture 17 Lecture 8 Models for Censored and Truncated Data - Tobit Model 1 Censored and Truncated Data • In some data sets we do not observe values above or below a certain magnitude, due to a censoring or truncation mechanism. Examples : - A central bank intervenes to stop an exchange rate falling below or going above certain levels. - Dividends paid by a company may remain zero until earnings reach some threshold value. - A government imposes price controls on some goods. - A survey of only working women, ignoring non-working women. In these situations, the observed data consists of a combination of measurements of some underlying latent variable and observations that arise when the censoring/truncation mechanism is applied. 2 RS – Lecture 17 Censored and Truncated Data: Definitions • Y is censored when we observe X for all observations, but we only know the true value of Y for a restricted range of observations. Values of Y in a certain range are reported as a single value or there is significant clustering around a value, say 0. - If Y = k or Y > k for all Y, then Y is censored from below or left-censored. - If Y = k or Y < k for all Y, then Y is censored from above or right-censored. We usually think of an uncensored Y, Y*, the true value of Y when the censoring mechanism is not applied. We typically have all the observations for { Y,X }, but not {Y*,X }. • Y is truncated when we only observe X for observations where Y would not be censored. We do not have a full sample for { Y,X }, we exclude observations based on characteristics of Y. Censored and Truncated Data: Example 1 No censoring or truncation 10 8 6 y 4 2 0 0 2 4 6 x • We observe the full range of Y and the full range of X. RS – Lecture 17 Censored and Truncated Data: Example 2 Censored from above 10 8 6 y 4 2 0 0 2 4 6 x • If Y ≥ 6, we do not know its exact value. Example : A Central Bank intervenes if the exchange rate hits the band’s upper limit. => If S t ≥ Ē => S t= Ē . Censored and Truncated Data: Example 2 0.45 Pdf(S t) 0.40 0.35 Prob(S t≤ Ē) 0.30 observed part Prob(S t> Ē) 0.25 0.20 0.15 0.10 0.05 0.00 Ē St • The pdf of the exchange rate, S t, is a mixture of discrete (mass at St=Ē) and continuous (Prob[S t<Ē]) distributions. RS – Lecture 17 Censored and Truncated Data: Example 3 Censored from below 10 8 6 y 4 2 0 0 2 4 6 x • If Y ≤ 5, we do not know its exact value. Example : A Central Bank intervenes if the exchange rate hits the band’s lower limit. => If S t ≤ Ē => S t= Ē. Censored and Truncated Data: Example 3 PDF(y *) f(y*) Prob(y*<5) Prob(y*>5) 5 y* • The pdf of the observable variable, y, is a mixture of discrete (prob. mass at Y=5) and continuous (Prob[Y*>5]) distributions. 8 RS – Lecture 17 Censored and Truncated Data: Example 3 PDF(y *) Prob(y*<5) Prob(y*>5) 5 Y • Under censoring we assign the full probability in the censored region to the censoring point, 5. 9 Censored and Truncated Data: Example 4 Truncated 10 8 6 y 4 2 0 0 2 4 6 x • If Y < 3, the value of X (or Y) is unknown. (Truncation from below.) Example : If a family’s income is below certain level, we have no information about the family’s characteristics. RS – Lecture 17 Censored and Truncated Data: Example 4 PDF(Y) 0.45 0.40 0.35 Prob[Y>3] < 1.0 0.30 0.25 0.20 0.15 0.10 0.05 0.00 1 5 93 13 17 21 25 29 33 37 41 45Y 49 • Under data censoring, the censored distribution is a combination of a pmf plus a pdf. They add up to 1. We have a different situation under truncation. To create a pdf for Y we will use a conditional pdf. Censored Normal • Moments: Let y*~ N(µ*, σ2) and α = ( c – µ*)/ σ. Then - Data: yi = yi* if yi* ≥ c = c if yi* ≤ c - Prob(y = c |x) = Prob(y* ≤ c |x) = Prob[(y*- µ*)/σ ≤ (c - µ*)/σ|x] = Prob[z ≤ (c - µ*)/σ|x] = Φ(α) - Prob(y > c |x) = Prob(y* > 0|x) = 1- Φ(α) - First Moment - Conditional: E[y|y*> c] = µ*+ σ λ(α) - Unconditional: E[y] = Φ(α) c + (1- Φ(α)) [µ*+ σ λ(α)] 12 RS – Lecture 17 Censored Normal • To get the first moment, we use a useful result –proven later- for a truncated normal distribution. If v is a standard normal variable and the truncation is from below at c, a constant, then φ(c) E(v | v > c) = F −1 f = 1− Φ(c) - In our conditional model, c = -(xi’β). (Note that the expectation is also conditioned on x, thus x is treated as a constant.). -1 - Note : The ratio Fi fi (a pdf dividided by a CDF) is called Inverse Mill’s ratio , usually denoted by λ(.) –the hazard function . If the truncation is from above: φ(c) λ(c) = − 1− Φ(c) 13 Censored Normal • To get the first moment, we use a useful result –proven later- for a truncated normal distribution. If v is a standard normal variable and the truncation is from below at c, a constant, then φ(c) φ(−c) E(v | v > c) = F −1 f = = 1− Φ(c) Φ(−c) - In our conditional model, c = -(xi’β). (Note that the expectation is also conditioned on x, thus x is treated as a constant.). -1 - Note : The ratio Fi fi (a pdf dividided by a CDF) is called Inverse Mill’s ratio , usually denoted by λ(.) –the hazard function . If the 0.45 truncation is from above: 0.40 0.35 0.30 φ(c) 0.25 λ(c) = − 0.20 1− Φ(c) 0.15 0.10 0.05 0.00 14 RS – Lecture 17 Censored Normal • Moments (continuation): - Unconditional first moment: E[y] = Φ(α) c + (1- Φ(α)) [µ*+ σ λ(α)] If c =0 => E[y] = (1- Φ(–µ*/ σ)) [µ*+ σ λ(α)] = Φ(µ*/ σ)) [µ*+ σ λ(α)] - Second Moment (1 − δ ) Vary2 1 ()()=σ() −Φ α 2 +()() α−λ Φ α whereδ=λ2 −λα 0 ≤δ≤ 1 15 Censored Normal – Hazard Function • The moments depend on λ(c), the Inverse Mill’s ratio or hazard rate evaluated at c: φ(c) φ(−c) λ(c) = = 1− Φ(c) Φ(−c) It is a monotonic function that begins at zero (when c = -∞) and asymptotes at infinity (when c = -∞). See plot below for (–c). 16 RS – Lecture 17 Truncated Normal • Moments: Let y*~ N(µ*, σ2) and α = ( c – µ*)/ σ. - First moment: E[y*|y> c] = µ* + σ λ(α) <= This is the truncated regression. => If µ>0 and the truncation is from below –i.e., λ(α) >0–, the mean of the truncated variable is greater than the original mean Note : For the standard normal distribution λ(α) is the mean of the truncated distribution. - Second moment: - Var[y*|y> c] = σ2[1 - δ(α)] where δ(α) = λ(α) [λ(α)- α] => Truncation reduces variance! This result is general, it applies to upper or lower truncation given that 0 ≤ δ(α) ≤ 1 17 Truncated Normal * Model: yi = X iβ + εi Data: y = y* | y* > 0 f(y|y *>0,X) F(0|X ) f(y*|X) 0 β Xi Xiβ+σλ • Truncated regression model: * E(y i| yi > 0,X i) = X iβ + σλ i 18 RS – Lecture 17 Censored and Truncated Data: Intuition • To model the relation between the observed y and x, we consider a latent variable y* that is subject to censoring/truncation. A change in x affects y only through the effect of x on y*. - Model the true, latent variable: y* = f(x ) = X β + error - Observed Variable: y = h(y*) • Q: What is this latent variable? Considered the variable “Dividends paid last quarter”? - For all the respondents with y=0 ( left censored from below), we think of a latent variable for “excess cash” that underlies “dividends paid last quarter.” Extremely cash poor would pay negative dividends if that were possible. Censored and Truncated Data: Problems • In the presence of censoring/truncation, we have a dependent variable, y, with special attribute(s): (1) constrained and (2) clustered of observations at the constraint. Examples : - Consumption (1, not 2) - Wage changes (2, not 1) - Labor supply (1 & 2) • These attributes create problems for the linear model. RS – Lecture 17 Censored and Truncated Data: Problems • Censoring in a regression framework (from Ruud). • If y is constrained and if there is clustering – OLS on the complete sample biased and inconsistent. – OLS on the unclustered part biased and inconsistent. Data Censoring and Corner Solutions • The dependent variable is partly continuous, but with positive mas at one or more points. Two different cases: (1) Data censoring (from above or below) - Surveys for wealth (upper category $250,000 or more) - Duration in unemployment - Demand for stadium tickets => Y* is not observed because of constraints, selection technique or measuring method (data problem) - We are interested in the effect of x on y*: E( y*| x). =>If yi* = x i’ β + εi, βj measures the effect of a change of xj on y*.
Details
-
File Typepdf
-
Upload Time-
-
Content LanguagesEnglish
-
Upload UserAnonymous/Not logged-in
-
File Pages26 Page
-
File Size-