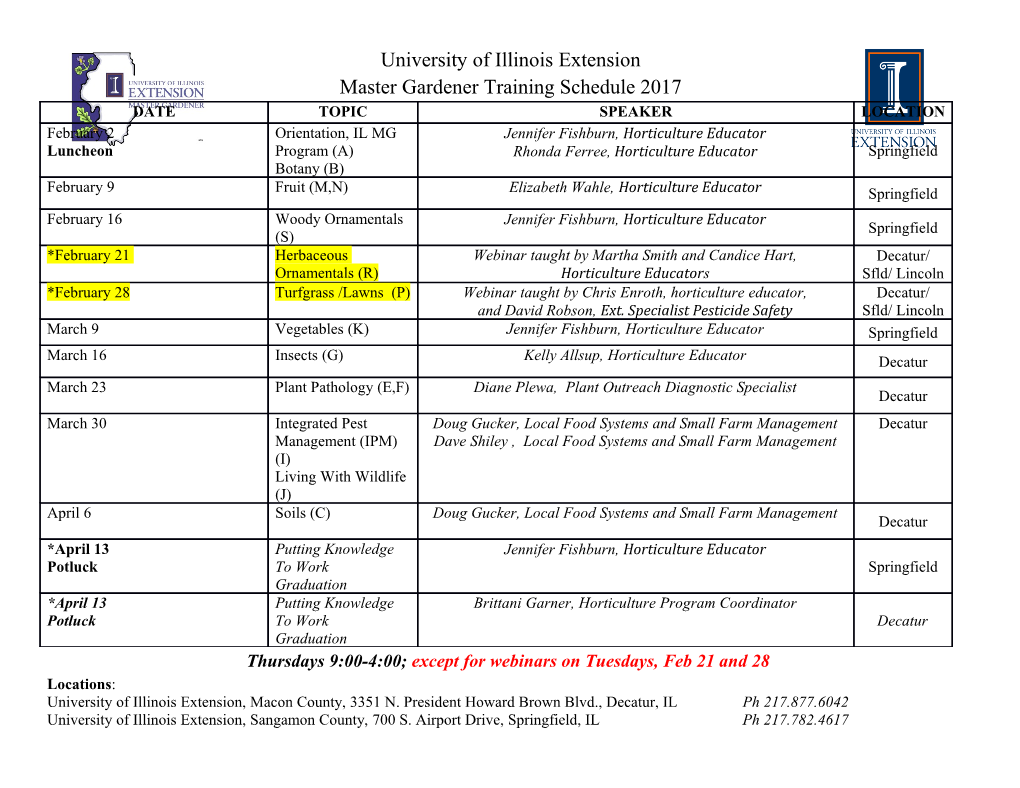
Predicate Representations and Polysemy in VerbNet Semantic Parsing James Gung∗ Martha Palmer University of Colorado Boulder University of Colorado Boulder [email protected] [email protected] Abstract Billy consoled the puppy PB Arg0 console.01 Arg1 Despite recent advances in semantic role la- beling propelled by pre-trained text encoders VN Stimulus amuse-31.1 Experiencer like BERT, performance lags behind when ap- plied to predicates observed infrequently dur- Billy walked the puppy ing training or to sentences in new domains. PB Arg0 walk.01 Arg1 In this work, we investigate how semantic role labeling performance on low-frequency VN Agent run-51.3.2-2-1 Theme predicates and out-of-domain data can be im- Table 1: Comparison of PropBank (PB) and VerbNet proved by using VerbNet, a verb lexicon that (VN) roles for predicates console and walk. VerbNet’s groups verbs into hierarchical classes based on thematic role assignments (e.g. Stimulus vs. Agent shared syntactic and semantic behavior and de- and Experiencer vs. Theme) are more dependent on fines semantic representations describing rela- the predicate than PropBank’s numbered arguments. tions between arguments. We find that Verb- Net classes provide an effective level of ab- straction, improving generalization on low- frequency predicates by allowing them to learn large gains in performance through the use of pre- from the training examples of other predicates trained text encoders like ELMo and BERT (Peters belonging to the same class. We also find et al., 2018; Devlin et al., 2019). Despite these ad- that joint training of VerbNet role labeling and vances, performance on low-frequency predicates predicate disambiguation of VerbNet classes and out-of-domain data remains low relative to for polysemous verbs leads to improvements in-domain performance on higher frequency predi- in both tasks, naturally supporting the extrac- cates. tion of VerbNet’s semantic representations. The assignment of role labels to a predicate’s 1 Introduction arguments is dependent upon the predicate’s sense. PropBank (Palmer et al., 2005) divides each pred- Semantic role labeling (SRL) is a form of shallow icate into one or more rolesets, which are coarse- semantic parsing that involves the extraction of grained sense distinctions that each provide a set predicate arguments and their assignment to consis- of core numbered arguments (A0-A5) and their tent roles with respect to the predicate, facilitating corresponding definitions. VerbNet (VN) groups the labeling of e.g. who did what to whom (Gildea verbs into hierarchical classes, each class defining and Jurafsky, 2000). SRL systems have been a set of valid syntactic frames that define a direct broadly applied to applications such as question correspondence between thematic roles and syntac- answering (Berant et al., 2014; Wang et al., 2015), tic realizations, e.g. Agent REL Patient (e.g. John machine translation (Liu and Gildea, 2010; Bazraf- broke the vase) or Patient REL (e.g. The vase broke) shan and Gildea, 2013), dialog systems (Tur and for break-45.1 (Schuler, 2005). Hakkani-Tur¨ , 2005; Chen et al., 2013), metaphor Recent PropBank (PB) semantic role labeling detection (Stowe et al., 2019), and clinical infor- models have largely eschewed explicit predicate mation extraction (Gung, 2013; MacAvaney et al., disambiguation in favor of direct prediction of se- 2017). Recent approaches to SRL have achieved mantic roles in end-to-end trainable models (Zhou ∗Work done prior to joining Amazon. and Xu, 2015; He et al., 2017; Shi and Lin, 2019). 51 Proceedings of the 14th International Conference on Computational Semantics, pages 51–62 June 17–18, 2021. ©2021 Association for Computational Linguistics This is possible for several reasons: First, Prop- roles that, unlike PB numbered arguments, main- Bank’s core roles and modifiers are shared across tain consistent meanings across different verbs and all predicates, allowing a single classifier to be classes. VN classes provide an enumeration of syn- trained over tokens or spans. Second, although tactic frames applicable to each member verb, de- definitions of PB roles are specific to the different scribing how the thematic roles of a VN class may senses of each predicate, efforts are made when be realized in a sentence. Every syntactic frame en- creating rolesets to ensure that A0 and A1 exhibit tails a set of low-level semantic representations properties of Dowty’s prototypical Agent and pro- (primitives) that describe relations between the- totypical Patient respectively (1991). Finally, PB matic role arguments as well as changes throughout rolesets are defined based on VN class membership, the course of the event (Brown et al., 2018). The with predicates in the same classes thus being as- close relationship between syntactic realizations signed relatively consistent role definitions (Bonial and semantic representations facilitates straightfor- et al., 2010). ward extraction of VN semantic predicates given Unlike PropBank, VerbNet’s thematic roles are identification of a VN class and corresponding the- shared across predicates and classes with consis- matic roles. VN primitives have been applied to tent definitions. However, VN roles are more de- problems such as machine comprehension (Clark pendent on the identity of the predicate (Zapirain et al., 2018) and question generation (Dhole and et al., 2008; Merlo and Van Der Plas, 2009). Ex- Manning, 2020). amples of PropBank and VerbNet roles illustrating this are given in Table1. Consequently, VN role Comparing VerbNet with PropBank Yi et al. labeling models may benefit more from predicate (2007) use VN role groupings to improve label features than PropBank. Furthermore, while it is consistency across verbs by reducing the overload- possible to identify PB or VN roles without classi- ing of PropBank’s numbered arguments like A2. fying predicate senses, linking the resulting roles Comparing SRL models trained on PB and VN, to their definitions or to the syntactic frames and Zapirain et al.(2008) find that their VerbNet model associated semantic primitives in VN does require performs worse on infrequent predicates than their explicit predicate disambiguation (Brown et al., PB model, and suggest that VN is more reliant on 2019). Therefore, predicate disambiguation is of- the identity of the predicate than PB based on exper- ten an essential step when applying SRL systems iments removing predicate-specific features from to real-world problems. their models. They suggest that the high consis- In this work, we evaluate alternative approaches tency of A0 and A1 enables PB to generalize better for incorporating VerbNet classes in English Verb- without relying on predicate-specific information. Net and PropBank role labeling. We propose a Merlo and Van Der Plas(2009) provide an joint model for SRL and VN predicate disambigua- information-theoretic perspective on the compari- tion (VN classification), finding that joint training son of PropBank and VerbNet, demonstrating how leads to improvements in VN classification and the identity of the predicate is more important to role labeling for out-of-domain predicates. We also VN SRL than for PB by comparing the conditional evaluate VN classes as predicate-specific features. entropy of roles given verbs as well as the mutual Using gold classes, we observe significant improve- information of roles and verbs. In multilingual ments in both PB and VN SRL. We also observe BERT probing studies comparing several SRL for- improvements in VN role labeling when using pre- malisms, Kuznetsov and Gurevych(2020) find that dicted classes and features that incorporate all valid layer utilization for predicates differs between PB classes for each predicate1. and VN. PB emphasizes the same layers used for syntactic tasks, while VN uses layers associated 2 Background and Related Work with tasks used more prevalently in lexical tasks. These findings reinforce the importance of predi- VerbNet VerbNet is a broad-coverage lexicon cate representations to VerbNet. that groups verbs into hierarchical classes based on shared syntactic and semantic behavior (Schuler, SRL and Predicate Disambiguation Previous 2005). Each VN class is assigned a set of thematic work has investigated the interplay between pred- 1Our code is available at https://github.com/ icate sense disambiguation and SRL. Dang and jgung/verbnet-parsing-iwcs-2021. Palmer(2005) improve verb sense disambiguation 52 (VSD) using features based on semantic role labels. ing of a sentence is produced using the target pred- Moreda and Palomar(2006) find that explicit verb icate as the Sent. B input to BERT. For example, senses improve PB SRL for verb-specific roles like the sentence I tried opening it is processed as: A2 and A3, but hurt on adjuncts. Yi(2007) find CLS I tried opening it SEP opening SEP that using gold standard PB roleset IDs as features in an SRL model improves performance only on for the verb open. This enables BERT to incorpo- highly polysemous verbs. Dahlmeier et al.(2009) rate the identity of the predicate in the encoding of propose a joint probabilistic model for preposition each word while clearly delineating it from tokens disambiguation and SRL, finding an improvement in the original sentence. over independent models. To simplify notation, we’ll treat LM(a; b) 2 T ×D Predicate disambiguation plays a critical role R a LM as shorthand for the final layer BERT en- in FrameNet (Baker et al., 1998) parsing, in part coding for a pair of sentences a = w1; :::; wTa and b = w ; :::; w T T because FrameNet’s role inventory is more than 1 Tb with a and b words respectively, an order of magnitude larger than that of PB and where DLM gives BERT’s hidden size. This is pro- VN. This richer, more granular role inventory lends duced by applying WordPiece tokenization (WP) advantages to approaches that constrain role iden- to each word in each sentence and concatenating tification to the set of valid roles for the predicted the resulting sequences of token IDs with standard frame (Das et al., 2014; Hermann et al., 2014), BERT-specific IDs: or that jointly encode argument and role represen- w = CLS; WP(a); SEP; WP(b); SEP tations given identified frames (FitzGerald et al., 2015).
Details
-
File Typepdf
-
Upload Time-
-
Content LanguagesEnglish
-
Upload UserAnonymous/Not logged-in
-
File Pages12 Page
-
File Size-