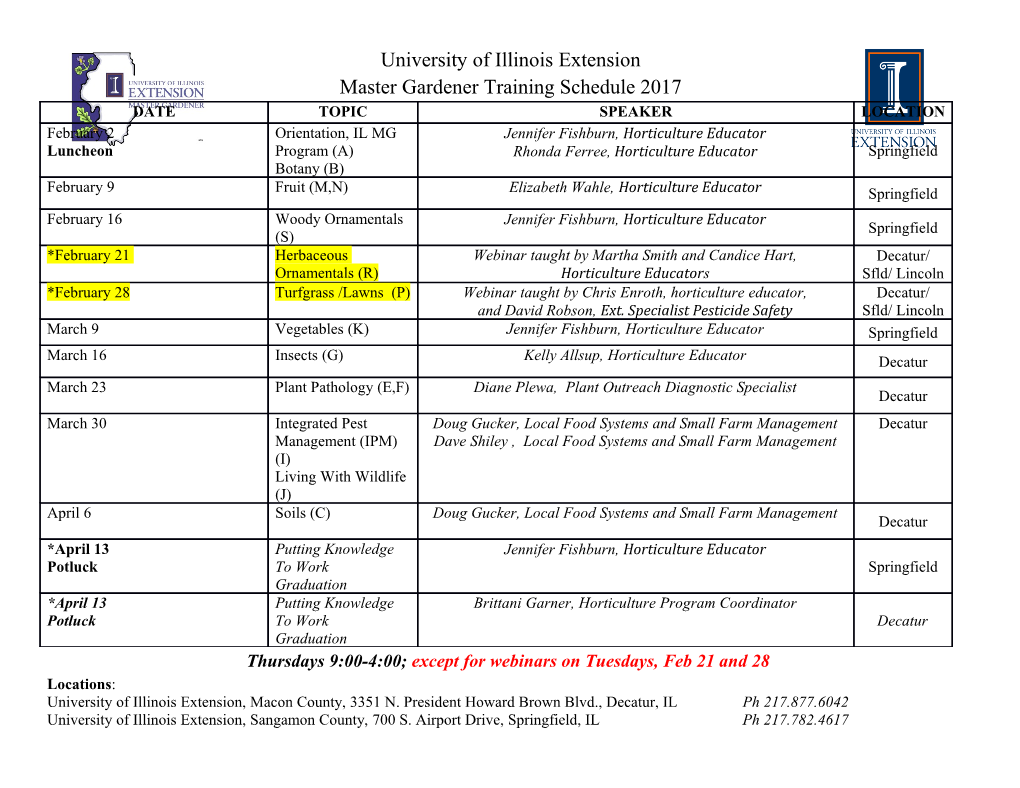
A Test of Media Capture by Using Machine Learning Techniques Evidence from Italian Television News 2010-2014 ∗ Andrea De Angelis y Alessandro Vecchiato September 9, 2016 VERY PRELIMINARY. PLEASE DO NOT CIRCULATE. Abstract A central question in political communication refers to the existence and extent of me- dia bias and how that could affect political stability and electoral outcomes. We address this question by using novel methodologies based on machine learning techniques and an origi- nal dataset collecting the entire corpus of national TV news outlets (including Rai, Mediaset, and “LA7” TV networks) in Italy from 2010 to 2014. Textual models can perform linguistic and substantive analysis of large corpus of text by exploiting variation in language use across and within authors, documents and time. In this paper we first estimate ideology scores for each TV outlet and analyze their change in the period under study. Secondly, we exploit the discontinuity in public TV ownership in our data, to determine existence of media bias in the news outlets as a way to make the public TV a more “favorable” environment for the incum- bent right-wing government. Finally, we identify key news topics and track their saliency over time and across networks. This methodology allows us to test whether political lean- ing in the news is due to strategic issue selection in news coverage or to differing frames in communication of the news stories. ∗ Andrea De Angelis, European University Institute, Dpt. of Political and Social Sciences, via dei Roccettini 9 I- 50014, San Domenico di Fiesole (Italy); [email protected]; y New York University, Wilf Family Dept. of Politics, 19 W 4th Street, Room 302, 10012 New York, NY; alessan- [email protected]; 1 1 Introduction Several papers find a remarkable correlation between the set up of the media landscape and political outcomes in the US and around the world (see e.g. Djankov et al.(2003); McMillan and Zoido(2004); Reinikka and Svensson(2005); Gentzkow and Shapiro(2010); Durante and Knight(2012); DellaVigna et al.(2016)). To understand the complex interaction between media outlet and political actors, both economists and political scientists have examined the specific factors that shape incentives to manipu- late the quality and content of news that reach voters: media owners can exploit political connections to reach funding resources outside the advertisment market, politicians bene- fit from a favorable media arena that reduces voters monitoring ability and therefore their accountability1 (Besley and Prat, 2006; Prat and Stromberg, 2013). One crucial problem is how the ownership structure affects news selection and framing to favor a specific political party Strömberg(2015). This paper follows the literature on media and explores the role of news and issue framing as an alternative measure of bias. Research in economics suggests that media outlet show a strong liberal leaning. Groseclose and Mi- lyo(2005) reports that in the universe of US news media, only Fox News and the Washington Times received scores at the right of the center. Bias can be driven from owners preferences or audience demand2. Media outlets can deliberately modify their language by including political slant in order to attract readers with similar political views Gentzkow and Shapiro (2010). Alternatively, they may follow the ideological sensibility of their ownership or a po- litical party their are trying to support. In this last case, bias emerges as a result of political capture of the media. We focus on this case and provide evidence of media capture by ex- ploiting quasi-exogenous variation in media ownership. To investigate this relationship we face several challenges. First, media ideological po- sition is very difficult to estimate. A number of papers have resorted to various techniques that detect ideological correlates in newspaper language usage. Groseclose and Milyo(2005) considers a group of 200 prominent think tanks or policy groups and counts the times a par- ticular member of the Congress cited one of them. They perform the same procedure for a number of newspapers and other media outlet and assign an ideological score to each of them on the basis of the frequency in which each think tank was nominated. This procedure allows them to link the ideological bias of media outlets to the one of others political actors and so derive an ideological measure of media. A more recent paper by Gentzkow and Shapiro (2010) focuses in a similar fashion on media slant. To assign a particular ideological leaning to specific language they refer to the Congressional Records and identify those set of phrases that are used much more frequently by one party than the other. Secondly, they calculate the number of time each outlet resorts to particular language that may sway voter to the left or the right of the political spectrum and assign to each of them the corresponding ideological score. However, while these measures may be able to capture newspapers ideological leaning, are focused on a specific way in which the media outlet may try to influence reporting. We overcome this difficulty by adopting a new unsupervised machine learning technique first introduced by Slapin and Proksch(2008). WORDFISH is a scaling algorithm that estimates policy positions based on word frequencies in texts. Following the naïve Bayes assumption 1For a comprehensive review of the results on media and politics read Strömberg(2015). 2See below for more extensive results on theoretical models of bias. 2 prevalent on text analysis literature (Eyheramendy et al., 2003), this algorithm represents a text as a vector of words counts. Individual words are assumed to be distributed at random, and word frequencies to be generated by a Poisson process. The procedure treats each piece of text as expression of a separate ideological position, and based on their word frequencies, estimates for each of them the relative weight of words in discriminating among ideological positions, together with the ideology score of the document. All parameters are estimated simultaneously for the entire corpus of text. The model can be expressed as follows: yijt ∼ P oisson(λijt) (1) λijt = exp(αit + j + βj · !it) (2) where: i indexes documents, j the tokens (i.e. words stems when only unigrams are used, or ngrams), and t to time. The only Poisson parameter λijt is modelled as a function of three latent components: αit are the document-specific fixed effect, j are word-specific fixed ef- fects (capturing the relative frequency of the words), βj are the word-specific discrimination weight parameters, capturing the ability of words to discriminate between ideological posi- tions, and finally !it estimate the latent position of the document. This process allows not only to estimate relative scores across political actor based on relative word frequencies, but also, by assuming independence across texts, over time. The omega scores thus represents measures of ideology within the spectrum of the available texts and are allowed to change over time. A second challenge comes from the intrinsic unobservable nature of media capturing practices. Politicians may influence the media through bribes, legal favoritisms or by ap- pointing sympathetic managers. McMillan and Zoido(2004), for instance, uses a secret po- lice account of government bribes to investigate corruption in Peru. They find that media and especially TV channels were receiving the largest shares of bribes during the Fujimori regime. Tella and Franceschelli(2011) uses government advertisement practices as a proxy for favoritism. They find that newspapers with government advertising are less likely to talk about government corruption, with one standard deviation increase in government adver- tising being associated with a decrease in coverage of corruption scandals of 0.23 of a front page per month. Nevertheless, these measure do not allow for a systematic study of capture given their rarity or their underestimation of the extent of corruption (advertisement may be only one of the strategies the government use to favor specific actors). Our setup provides a number of solutions to these challenges. The Italian media land- scape has been widely criticized for its general lack of impartiality, to the point of blatant political bias. The public ownership of three media channel, Rai 1, Rai 2 and Rai 3, gives the government the prerogative of nominating their CEOs and newscasts editors. Grasso(2004) describes the historical process that led to the politicization of the Italian State television in detail. In 1975, the government reformed3 the national television system, shifting the pre- rogative of nominating the TV managers from the government to the parliament. This led to a practice called ‘lottizzazione’ (lotting) that resulted in the consistent nomination as di- rectors and editors of figures that were politically affiliated with specific national parties. Thus, each channel had a well-defined (though not formally stated) political affiliation: Rai1 3Legge n. 103 del 14 aprile 1975 in matters of national television broadcasting. 3 Figure 1: Patrick Chiappori on Berlusconi Resignation. Source: New York Times typically supported the incumbent government (Democrazia Cristiana), Rai2 supported the Socialist Party, and Rai3 the Communist Party (or the far left). This practice evolved after 1993 toward a stronger focus for television newscasts. After each election, with a new parliament and government in place, the editors of the national channels (particularly Rai1 and Rai2) were typically replaced with journalists or political figures closer to the new establishment. This problem was exacerbated by the candidacy of Silvio Berlusconi, a media and real estate tycoon, who entered the political arena in the aftermath of the Mani Pulite scandal in 1993. When in power, Silvio Berlusconi, never completely divested its properties of three TV channels, Canale 5, Italia 1 and Rete 4, officially controlling either directly or indirectly 86% of the media market in Italy.
Details
-
File Typepdf
-
Upload Time-
-
Content LanguagesEnglish
-
Upload UserAnonymous/Not logged-in
-
File Pages29 Page
-
File Size-