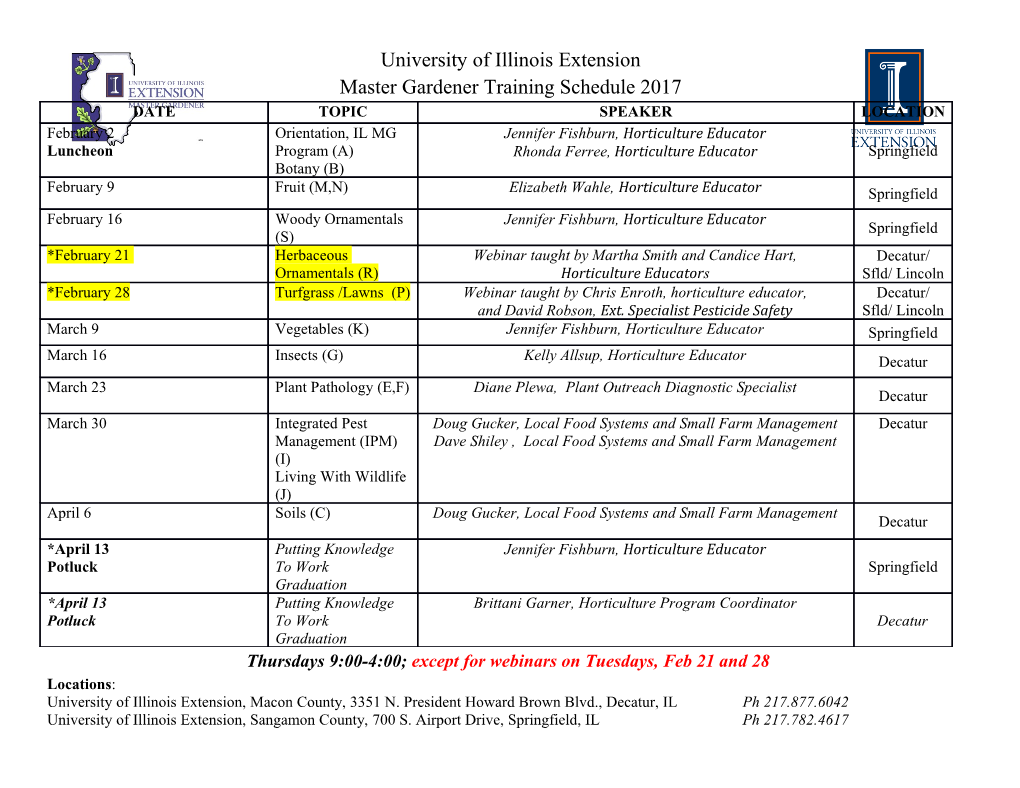
AN INTRODUCTION TO HILBERT SPACES AND THE HEISENBERG UNCERTAINTY PRINCIPLE YU XIAO Abstract. In this paper, we will focus on one particular application of Hilbert spaces in quantum mechanics. We start with simple definitions of certain kinds of vector spaces that lead up to Hilbert spaces and follow by defining the Hermitian operator. Relevant, basic postulates of quantum mechanics will be given. We prove the Heisenberg Uncertatinty Principle and finish with a brief account of Hilbert spaces' importance and wider applications. Contents 1. Hilbert Spaces 1 2. Hermitian Operator 4 3. The Five Postulates of Quantum Mechanics 8 4. Heisenberg Uncertainty Principle 11 5. Importance and Wider Applications 13 Acknowledgments 13 References 13 1. Hilbert Spaces Why are Hilbert spaces so important? Essentially, a Hilbert space is a space that allows geometry. Exact analogs of the Pythagorean Theorem and Parallelogram Law hold in Hilbert spaces. To satisfy those requirements, a Hilbert space naturally must have many restrictions. To define Hilbert spaces, we shall start with an inner product space as it is simply a vector space with the additional structure of inner product. Definition 1.1. (Inner Product) Let E be a complex vector space. A mapping h·; ·i : E × E ! C is called an inner product in E if for any x; y; z 2 E and α; β 2 C the following conditions are all satisfied: (1) hx; yi = hy; xi (the bar denotes the complex conjugate); (2) hαx + βy; zi = αhx; zi + βhy; zi; (3) hx; xi ≥ 0, and hx; xi = 0 implies x = 0. A space with an inner product is called an inner product space. It is also called a pre-Hilbert space. Its alternate name clearly signifies its importance in defining Hilbert spaces. Date: August 18, 2017. 1 2 YU XIAO Definition 1.2. (Norm) A function x ! jjxjj from a vector space E into R is a norm if it satisfies the following conditions: (1) jjxjj = 0 if and only if x = 0; (2) jjλxjj = jλjjjxjj for every x 2 E and λ 2 C; (3) jjx + yjj ≤ jjxjj + jjyjj for every x; y 2 E. A vector space with a norm is called a normed space. It turns out that every inner product space is also a normed space with the norm defined by jjxjj = phx; xi The norm above jjxjj = phx; xi is well defined because condition (3) of Definition 1.1 clearly implies that jjxjj = 0 if and only if x = 0. Moreover, q jjλxjj = phλx, λxi = λλ¯hx; xi = jλjjjxjj: Therefore, we only need to prove for condition (3) of Definition 1.2, which we will begin by proving the Schwarz's Inequality Theorem. Theorem 1.3. (Schwarz's Inequality) For any two elements x and y of an inner product space, we have (1.4) jhx; yij ≤ jjxjjjjyjj The equality jhx; yij = jjxjjjjyjj holds if and only if x and y are linearly dependent. Proof. If y = 0, then the theorem holds since both sides equal zero. If y 6= 0, then (1.5) 0 ≤ hx + αy; x + αyi = hx; xi +α ¯hx; yi + αhy; xi + jαj2hy; yi Now substitute α = −hx; yi=hy; yi, then we have 0 ≤ hx; xihy; yi − jhx; yij2 Clearly, jhx; yij ≤ jjxjjjjyjj, giving us the theorem of Schwarz's Inequality. If x and y are linearly dependent, then x = αy for some α 2 C. Therefore, jhx; yij = jhx; αxij = jα¯jhx; xi = jαjjjxjjjjxjj = jjxjjjjαxjj = jjxjjjjyjj Now, let x and y be such vectors that hx; yi = jjxjjjjyjj. Equivalently, hx; yihy; xi = hx; xihy; yi. We have 2 y; y x − x; y y; y; y x − x; y y = y; y x; x − y; y y; x x; y − x; y y; y y; x + x; y y; x y; y = 0 So y; y x − x; y y = 0, which means that x and y are linearly dependent, thus completing the proof. Using Theorem 1.3 proven above, we can obtain condition (3) of Definition 1.2 as a corollary. Corollary 1.6. (Triangle Inequality) For any two elements x and y of an inner product space, (1.7) jjx + yjj ≤ jjxjj + jjyjj AN INTRODUCTION TO HILBERT SPACES 3 Proof. Taking α = 1 in Equation 1.5 and applying Schwarz's Inequality, we have jjx + yjj2 = x + y; x + y = x; x + 2< x; y + y; y ≤ x; x + 2j x; y j + y; y ≤ jjxjj2 + 2jjxjjjjyjj + jjyjj2 = (jjxjj + jjyjj)2 Therefore, jjx + yjj ≤ jjxjj + jjyjj So a inner product space is also a normed space. Obtaining an inner product space is the important first step in the process of trying to define Hilbert spaces, partly because the Parallelogram Law already holds in an inner product space. Theorem 1.8. (Parallelogram Law) For any two elements x and y of an inner product space we have (1.9) jjx + yjj2 + jjx − yjj2 = 2(jjxjj2 + jjyjj2) Proof. We have jjx + yjj2 = hx + y; x + yi = hx; xi + hx; yi + hy; xi + hy; yi; hence (1.10) jjx + yjj2 = jjxjj2 + hx; yi + hy; xi + jjyjj2: Now replace y by −y in Equation 1.10, to obtain (1.11) jjx − yjj2 = jjxjj2 − hx; yi − hy; xi + jjyjj2 Adding Equations 1.10 and 1.11 together, we have the parallelogram law. Having seen the usefulness of inner product spaces, we shall also give a proposi- tion about the continuity of the inner product. Proposition 1.12. (Continuity of Inner Product) If (xn) and (yn) are se- quences of elements of the inner product space E such that jjxn − xjj ! 0 and jjyn − yjj ! 0 when n ! 1 with x; y 2 E, then hxn; yni ! hx; yi: Proof. Applying Schwarz's Inequality, we have jhxn; yni − hx; yij = jhxn; yn − yi + hxn − x; yij ≤ jhxn; yn − yij + jhxn − x; yij ≤ jjxnjjjjyn − yjj + jjxn − xjjjjyjj: Since jjxn − xjj ! 0 and jjyn − yjj ! 0 when n ! 1, jhxn; yni − hx; yij ! 0. So, hxn; yni ! hx; yi when n ! 1. Of course, the continuity of inner products can also be extended to norms. Now that we have proven that an inner product space is also a normed space and given a proposition of the inner product, we can move on to the next step of defining Hilbert spaces: defining the completeness of a space. Definition 1.13. (Cauchy Sequence) A sequence of vectors (xn) in a normed space is called a Cauchy sequence if for every > 0 there exists a number M such that jjxm − xnjj ≤ for all m; n > M. 4 YU XIAO Definition 1.14. (Banach Space) A normed space E is called complete if every Cauchy sequence in E converges to an element of E. A complete normed space is called a Banach space. Definition 1.15. (Hilbert Space) A complete inner product space is called a Hilbert space. As the standard formulations of quantum mechanics require the Hilbert spaces used to be separable, we will here give the further definition of separable Hilbert spaces as well. A Hilbert space is separable if and only if it admits a countable orthonormal basis. Definition 1.16. (Orthogonal Vectors) Two vectors x and y in an inner product space are called orthogonal if hx; yi=0, denoted by x ? y. Definition 1.17. (Countable Set)A countable set is a set that has the same cardinality (number of elements) as some subbset of the set of all natural numbers. Definition 1.18. (Orthonormal Basis) Let E be an inner product space. A basis S of E with jjxjj = 1 for all x 2 S and x ? y for any two distinct elements of S is called an orthonormal basis. Definition 1.19. (Separable Space) A Hilbert space is called separable if it admits a countable orthonormal basis. Finite dimensional Hilbert spaces are separable, and orthogonal bases are used often when dealing with finite dimensional Hilbert spaces. It is only natural and sensible that we wish to still have them when working with infinite dimensions. 2. Hermitian Operator Linear maps are functions between two vector spaces that preserve the operations of vector addition and scalar multiplication. An operator (or transformation) is generally a mapping that maps elements of a space to produce elements of the same space. Linear operators on a normed vector space are widely used to represent physical quantities, hence they are very important in applied mathematics and mathematical physics. The most important operators include differential, integral, and matrix operators. For now, we are interested in the bounded linear operators on Hilbert space, particularly adjoint and self-adjoint (Hermitian) operators. Let us give some basic definitions that will be of use in the following definitions of adjoint and self-adjoint Hilbert operators and the relevant proofs. Definition 2.1. (Bounded Operator) An operator A is called bounded if there is a number K such that jjAxjj ≤ Kjjxjj for every x in the domain of A. Definition 2.2. (Linear Functional) A linear functional is a linear map from a vector space to its field of scalars. For vector space V over field C, a linear functional is a function f : V ! C that satisfies the following: (1) f(v + w) = f(v) + f(w) for all v; w 2 V ; (2) f(αv) = αf(v) for all v 2 V and α 2 C And based on the above definitions, we can prove our first simple and quite straightforward lemma, the continuity of bounded linear functionals. Lemma 2.3. Any bounded linear functional is continuous. AN INTRODUCTION TO HILBERT SPACES 5 Proof. For any bounded linear functional L on a Hilbert space H, there exists a number K such that jjL(x)jj ≤ Kjjxjj for any x 2 H.
Details
-
File Typepdf
-
Upload Time-
-
Content LanguagesEnglish
-
Upload UserAnonymous/Not logged-in
-
File Pages13 Page
-
File Size-