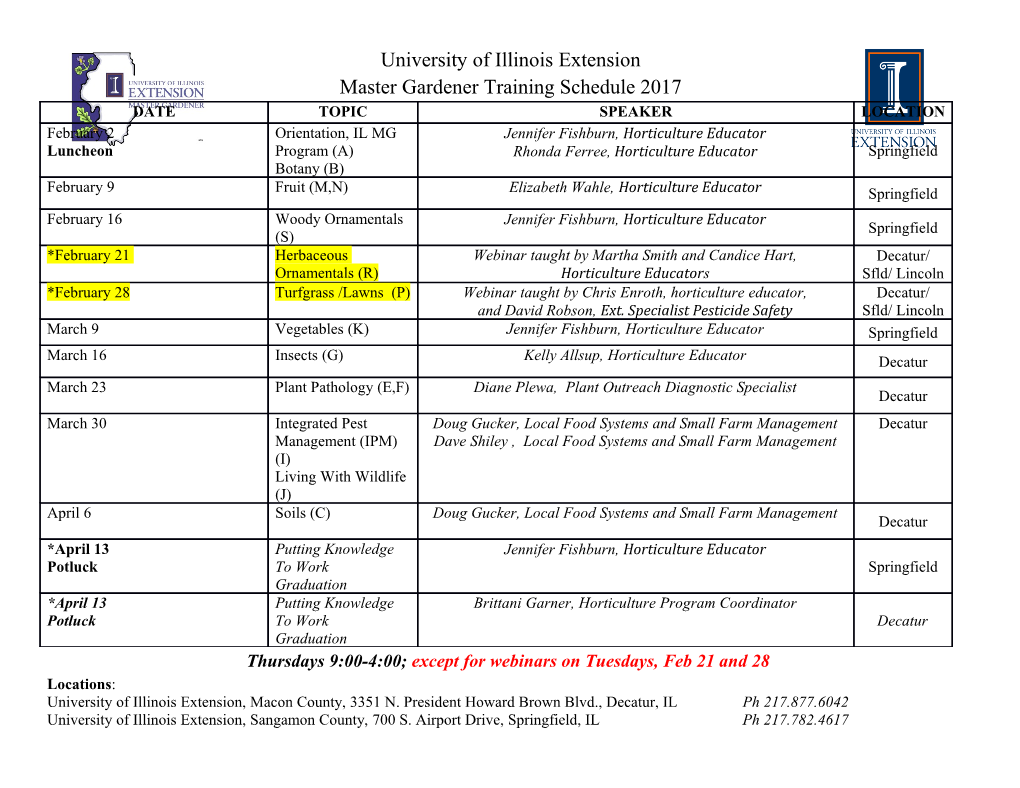
Linear transformations and determinants Math 40, Introduction to Linear Algebra Monday, February 13, 2012 Matrix multiplication as a linear transformation Primary example of a = matrix linear transformation ⇒ multiplication Given an m n matrix A, × define T (x)=Ax for x Rn. ∈ Then T is a linear transformation. Astounding! Matrix multiplication defines a linear transformation. This new perspective gives a dynamic view of a matrix (it transforms vectors into other vectors) and is a key to building math models to physical systems that evolve over time (so-called dynamical systems). A linear transformation as matrix multiplication Theorem. Every linear transformation T : Rn Rm can be → represented by an m n matrix A so that x Rn, × ∀ ∈ T (x)=Ax. More astounding! Question Given T, how do we find A? Consider standard basis vectors for Rn: Transformation T is 1 0 0 0 completely determined by its . e1 = . ,...,en = . action on basis vectors. 0 0 0 1 Compute T (e1),T(e2),...,T(en). Standard matrix of a linear transformation Question Given T, how do we find A? Consider standard basis vectors for Rn: Transformation T is 1 0 0 0 completely determined by its . e1 = . ,...,en = . action on basis vectors. 0 0 0 1 Compute T (e1),T(e2),...,T(en). Then || | is called the T (e ) T (e ) T (e ) 1 2 ··· n standard matrix for T. || | denoted [T ] Standard matrix for an example Example x 3 2 x T : R R and T y = → y z 1 0 0 1 0 0 T 0 = T 1 = T 0 = 0 1 0 0 0 1 100 2 A = What is T 5 ? 010 − 12 2 2 100 2 A 5 = 5 = ⇒ − 010− 5 12 12 − Composition T : Rn Rm is a linear transformation with standard matrix A Suppose → S : Rm Rp is a linear transformation with standard matrix B. → n m p R R R T S v w = T (v) u = S(w)=S(T (v)) (S T )(v) ◦ S ⸰T The composition S T : Rn Rp is a linear transformation and ◦ → since A is standard matrix of transformation T (S T )(x)=S(T (x)) = S(Ax)=B(Ax)=BAx =[S][T ] x = [S T ]=[S][T ] ◦ ⇒ ◦ since B is standard matrix of transformation S Inverse We say S and T are inverse linear transformations if S T = I and T S = I, ◦ ◦ where I is the identity transformation. Let T : Rn Rn be an invertible linear transformation. → Then its standard matrix [T ] is an invertible matrix, and 1 1 [T − ]=[T ]− . The matrix of the inverse is the inverse of the matrix! Introduction to determinants: 2x2 case The determinant is only defined for a square matrix. 2 x 2 matrices Definition For 2 2 matrix ab ,thedeterminant of A is × cd det(A)=ad bc. − a b also denoted |A| or c d Key A invertible det(A)=ad bc =0 property: ⇐⇒ − Introduction to determinants: 3x3 case abc 3 x 3 matrices If A = def, then we have ghi abc ab c A = def EROs A = 0 ae bd af cd −−−→ − − A invertible ghi 00 a∆ assume a =0 and ae bd =0 ∆ =0 − where ∆ =(aei ahf ) (bdi bgf )+(cdh cge) − − − − ef df de Observe that ∆ = a b +c How do these hi− gi gh relate to A? abc A , abc A12, abc 11 submatrix formed def submatrix formed def def A by deleting by deleting 13 ghi row 1, col 1 ghi row 1, col 2 ghi Definition of determinant 3 x 3 matrices ∆ = a det A11 b det A12 + c det A13 { { { − a11 a12 a13 n x n matrices For an n x n matrix, the determinant of A is det(A)=a det A a det A + +( 1)n+1a det A 11 11 − 12 12 ··· − 1n 1n n = ( 1) j+1 a 1j det A1j So the j=1 − determinants of determinant is signs of (n-1) x (n-1) defined entries of terms submatrices formed 1st row of A recursively. alternate by deleting 1st row and jth col Example of computing the determinant 123 56 46 45 456=1 2 +3 89− 79 78 789 = (5 9 6 8) 2(4 9 6 7) + 3(4 8 5 7) · − · − · − · · − · = 3 + 12 9 − − matrix is not = 0 = ⇒ invertible Amazing facts about determinants det A can be found by “expanding” along any row or any column original def’n expands across row 1 n i+j i is fixed, use row i = det A = ( 1) aij det Aij ⇒ − j varies j=1 n i+j j is fixed, use col j = det A = ( 1) aij det Aij ⇒ − i varies i=1 Amazing facts about determinants det A can be found by “expanding” along any row or any column sign pattern i+j + + + ( 1) det Aij − − ··· − + + { − − + + (i, j)-cofactor of A − . + .. − + det A = aijCij . . negative when indices sum to odd Amazing facts about determinants det A can be found by “expanding” along any row or any column 217 Example A = 132 − 010 Why do you love this 27 fact? det A =0 ∗∗ 1 +0 ∗∗ − 12 ∗∗ − ∗∗ = (2 2 7( 1)) − · − − = 11 − Amazing facts about determinants det A can be found by “expanding” along any row or any column Theorem. The determinant of a triangular Consequence: matrix is the product of its diagonal entries. 123 4 056 7 A = det(A)=1 5 8 10 = 400 008 9 · · · 0 0 0 10 Amazing facts about determinants EROs barely change the determinant, and they do so in a predictable way. EROs effect on det A Strategy to compute swap two rows changes sign det A more quickly for general matrices A = multiply row multiply det ⇒ by scalar c by scalar c Perform EROs to get REF of A and add c ຘ row i no change at all! compute det A based to row j on det of REF.
Details
-
File Typepdf
-
Upload Time-
-
Content LanguagesEnglish
-
Upload UserAnonymous/Not logged-in
-
File Pages8 Page
-
File Size-