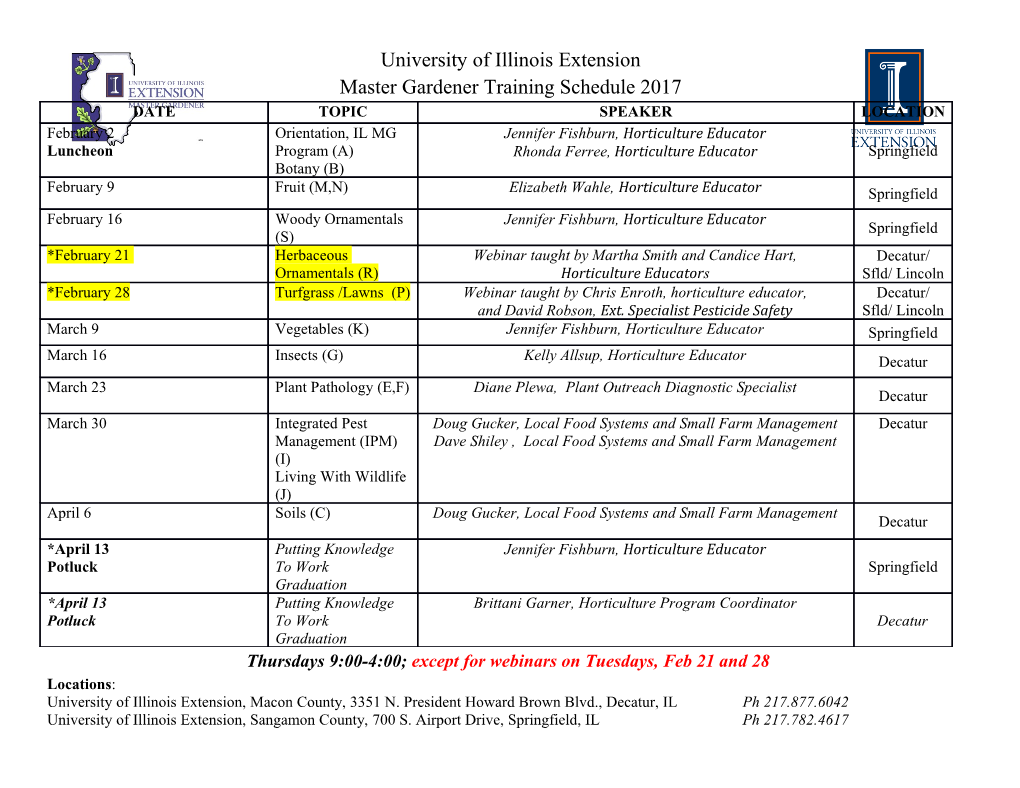
Computational Tools for Annotating Antibiotic Resistance in Metagenomic Data Gustavo Alonso Arango Argoty Dissertation submitted to the faculty of the Virginia Polytechnic Institute and State University in partial fulfillment of the requirements for the degree of Doctor of Philosophy in Computer Science and Applications Liqing Zhang, Chair, Amy Pruden Lenwood S. Heath Na Meng Weidong Xiao March 27, 2019 Blacksburg, Virginia Keywords: Metagenomics, Bioinformatics, Deep learning, Antibiotic resistance genes, Web services Computational Tools for Annotating Antibiotic Resistance in Metagenomic Data Gustavo Alonso Arango Argoty ABSTRACT Metagenomics has become a reliable tool for the analysis of the microbial diversity and the molecular mechanisms carried out by microbial communities. By the use of next generation sequencing, metagenomic studies can generate millions of short sequencing reads that are processed by computational tools. However, with the rapid adoption of metagenomics a large amount of data has been generated. This situation requires the development of computational tools and pipelines to manage the data scalability, accessibility, and performance. In this thesis, several strategies varying from command line, web-based platforms to machine learning have been developed to address these computational challenges. Interpretation of specific information from metagenomic data is especially a challenge for environmental samples as current annotation systems only offer broad classification of microbial diversity and function. Therefore, I developed MetaStorm, a public web-service that facilitates customization of computational analysis for metagenomic data. The identification of antibiotic resistance genes (ARGs) from metagenomic data is carried out by searches against curated databases producing a high rate of false negatives. Thus, I developed DeepARG, a deep learning approach that uses the distribution of sequence alignments to predict over 30 antibiotic resistance categories with a high accuracy. Curation of ARGs is a labor intensive process where errors can be easily propagated. Thus, I developed ARGminer, a web platform dedicated to the annotation and inspection of ARGs by using crowdsourcing. Effective environmental monitoring tools should ideally capture not only ARGs, but also mobile genetic elements and indicators of co-selective forces, such as metal resistance genes. Here, I introduce NanoARG, an online computational resource that takes advantage of the long reads produced by nanopore sequencing technology to provide insights into mobility, co-selection, and pathogenicity. Sequence alignment has been one of the preferred methods for analyzing metagenomic data. However, it is slow and requires high computing resources. Therefore, I developed MetaMLP, a machine learning approach that uses a novel representation of protein sequences to perform classifications over protein functions. The method is accurate, is able to identify a larger number of hits compared to sequence alignments, and is >50 times faster than sequence alignment techniques. Computational Tools for Annotating Antibiotic Resistance in Metagenomic Data Gustavo Alonso Arango Argoty GENERAL AUDIENCE ABSTRACT Antimicrobial resistance (AMR) is one of the biggest threats to human public health. It has been estimated that the number of deaths caused by AMR will surpass the ones caused by cancer on 2050. The seriousness of these projections requires urgent actions to understand and control the spread of AMR. In the last few years, metagenomics has stand out as a reliable tool for the analysis of the microbial diversity and the AMR. By the use of next generation sequencing, metagenomic studies can generate millions of short sequencing reads that are processed by computational tools. However, with the rapid adoption of metagenomics, a large amount of data has been generated. This situation requires the development of computational tools and pipelines to manage the data scalability, accessibility, and performance. In this thesis, several strategies varying from command line, web-based platforms to machine learning have been developed to address these computational challenges. In particular, by the development of computational pipelines to process metagenomics data in the cloud and distributed systems, the development of machine learning and deep learning tools to ease the computational cost of detecting antibiotic resistance genes in metagenomic data, and the integration of crowdsourcing as a way to curate and validate antibiotic resistance genes. ACKNOWLEDGMENTS I would like to thank my advisor Dr. Liqing Zhang for helping me through the learning process in my Ph.D. degree. Her knowledge, patience, guidance, and feedback were the core principles to identify and formulate novel solutions to different scientific challenges. I am really grateful for her support as she motivates not only the intellectual but also the personal success. I would like to thank Dr. Amy Pruden and Dr. Peter Vikesland for their valuable support, patience, and guidance towards an interdisciplinary research, Dr. Heath, for his helpful computational advice on the different projects I carried out, and Dr. Na Meng and Dr. Weidong Xiao for their support and their valuable suggestions for completing my degree. I am also grateful to Mohammad, Tihi, Min, Zhahoa, Hong and Dhoha, my lab mates, for their productive feedback during our meeting sessions as well as their experiences and wisdom at Virginia Tech that made the doctorate journey much easier. I would like to thank the support given by my siblings Manuel, Miguel, Martha and friends for their support through this long journey, because, without their support it wouldn’t be possible. iv TABLE OF CONTENTS CHAPTER 1 INTRODUCTION ............................................................................................... 1 1.1 Problems ...........................................................................................................................1 1.2 Overall aim .......................................................................................................................4 1.3 Specific aims .....................................................................................................................4 CHAPTER 2 CUSTOMIZABLE METAGENOMICS ANNOTATION .............................................. 5 2.1 Introduction......................................................................................................................5 2.2 Materials and Methods .....................................................................................................6 2.2.1 Required data types ................................................................................................................. 6 2.2.2 Reference database ................................................................................................................. 7 2.2.3 Web-based submission ............................................................................................................ 7 2.2.4 Analysis pipeline ...................................................................................................................... 8 2.2.4.1 Assembly pipeline ......................................................................................................................... 9 2.2.4.2 Read matching pipeline .............................................................................................................. 10 2.2.5 Sample normalization and comparison ................................................................................. 11 2.2.6 Visualization of taxonomic abundance .................................................................................. 11 2.2.7 Visualization of functional abundance .................................................................................. 12 2.2.8 Visualization of sample comparison ...................................................................................... 13 2.3 Data access...................................................................................................................... 14 2.4 Results and Discussion .................................................................................................... 14 2.5 Conclusion ...................................................................................................................... 14 CHAPTER 3 IMPROVING ANTIBIOTIC RESISTANCE ANNOTATION ...................................... 16 3.1 Introduction.................................................................................................................... 16 3.2 Materials and Methods ................................................................................................... 19 3.2.1 Database Merging .................................................................................................................. 19 3.2.2 ARG Annotation of CARD and ARDB ...................................................................................... 19 3.2.3 UNIPROT Gene Annotation .................................................................................................... 19 3.2.4 Deep Learning ........................................................................................................................ 22 3.3 Results and Discussion .................................................................................................... 24 3.3.1 Antibiotic Resistance Database.............................................................................................
Details
-
File Typepdf
-
Upload Time-
-
Content LanguagesEnglish
-
Upload UserAnonymous/Not logged-in
-
File Pages132 Page
-
File Size-