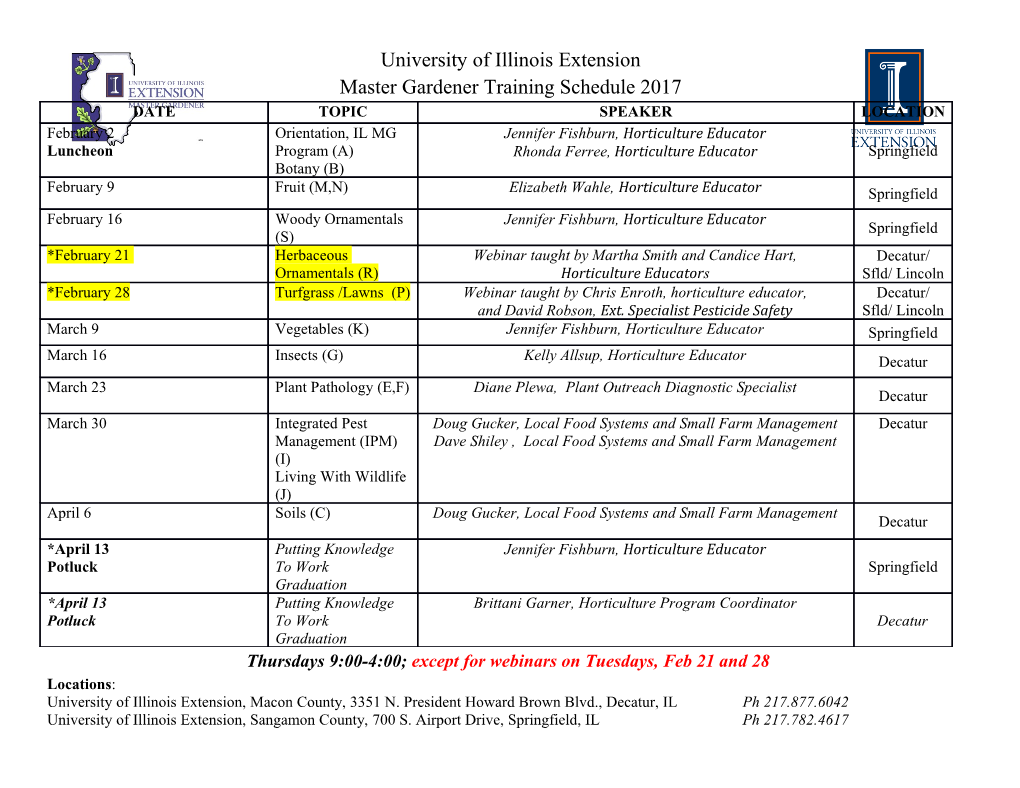
Math 217: Eigen Everything (c)2015 UM Math Dept Professor Karen E. Smith licensed under a Creative Commons By-NC-SA 4.0 International License. 1. Eigenvectors and Eigenvalues. Eigenvectors and eigenvalues are objects associated to a fixed linear transformation V !T V: Eigenvectors and eigenvectors always go together: each eigenvector of T has some associ- ated eigenvalue, and each eigenvalue has associated eigenvector(s). Definition 1.0.1. An eigenvector of a linear transformation V −!T V is any non-zero vector ~v 2 V such that T (~v) = λ~v for some scalar λ. The scalar λ is called the eigenvalue of the eigenvector ~v. Perhaps this is obvious, but it bears repeating: an eigenvector is a vector (in V ) and an eigenvalue is a scalar (in R). 1 d λx λx Example 1.0.2. Consider the differentiation map of C . Since dx e = λe for any real number λ, we see that every real number is an eigenvalue of the differentiation map, with λx corresponding eigenvectors fλ(x) = e : Caution: Not every linear transformation has an eigenvalues! ρ Example 1.0.3. Consider the map R2 −! R2 given by rotation counterclockwise through π=2. Since no non-zero vector is taken to a scalar multiple of itself, ρ has no eigenvectors. Definition 1.0.4. Let V −!T V be a linear transformation. An eigenbasis is a basis for V consisting of eigenvectors for T . Example 1.0.5. Consider the map 2 p 2 R −! R given by projection onto a subspace L. Since every point of L is taken to itself, we have p(~v) = ~v for all ~v 2 L. That is, every non-zero vector in L is an eigenvector with eigenvalue 1. Since every vector w 2 L? is send to 0 under p, we have p(~w) = 0~w for all ~w 2 L?. Thus every non-zero vector in L? is an eigenvector with eigenvalue 0. If we let ~v be a basis for L and ~w be a basis for L?, then (~v; ~w) is an eigenbasis for p. Caution: Not every linear transformation has an eigenbasis! Example 1.0.3 above is one that does not! Eigenvectors are important geometrically because they help us understand the map better— if we know ~v is an eigenvector with eigenvalue 2, we know that T is scaling vectors by 2 in the direction of ~v. 1 An Eigenbasis for a transformation T is important algebraically because its give us a coordinate system that is especially helpful for dealing with T . For example in Example 1.0.5, the matrix of p in the eigenbasis B = (~v; ~w) is 1 0 [p] = [p(~v)] [p(~w)] = : B B B 0 0 Imagine how much easier it is to work with this B-matrix instead of the standard or some other matrix of p! Proposition 1.0.6. Let V −!T V be a linear transformation of a finite dimensional vector space. Then B is an eigenbasis for T if and only if the matrix [T ]B is diagonal. In this case, the elements on the diagonal are the eigenvalues (possibly repeated more than once). Definition 1.0.7. A linear transformation V −!T V is diagonalizable if V admits an eigenbasis for T . Equivalently,1 V −!T V is diagonalizable means that there is some basis for V in which the matrix of T is diagonal. Any such basis will be an eigenbasis for T . In terms of matrices, we have Definition 1.0.8. An n × n matrix is diagonalizable if it is similar to a diagonal ma- TA trix. This is equivalent to saying that the linear transformation Rn −! Rn (given by left multiplication by A) is diagonalizable. Proposition 1.0.9. Let A be an n × n matrix. Then A is similar to a diagonal matrix D if and only if the linear transformation given by left multiplication by A has an eigenbasis. Moreover, if f~v1; : : : ;~vng is the eigenbasis, with corresponding eigenvalues λ1; : : : ; λn, then 2λ1 0 ::: 0 3 0 λ ::: 0 −1 6 2 7 S AS = 6 . 7 ; 4 . :::::: . 5 0 0 : : : λn where S is the matrix ~v1 : : : ~vn whose columns are the vectors of the eigenbasis. T 2 3 Example 1.0.10. Consider the map 2 −! 2 given by left multiplication by : R R 0 −1 1 Note that T (~e ) = 2~e . Also, for ~v = , we have T (~v) = −~v. So 1 1 −1 1 1 B = ( ; ) 0 −1 is an eigenbasis and the matrix of T in this eigenbasis is 2 0 [T ] = : B 0 −1 Note that the elements on the diagonal are exactly the eigenvalues f2; −1g. 1 assuming V is finite dimensional so we can model it on Rn 2 2. Eigenspaces Proposition 2.0.1. Let λ be an eigenvalue of a linear transformation V −!T V . The set Vλ = f~v 2 V j T (~v) = λ~vg ⊂ V consisting of all λ-eigenvectors (together with ~0) is a subspace of V: Proof. You should prove this, using the standard technique for checking a subset of V is a subspace. Definition 2.0.2. Let V −!T V be a linear transformation with eigenvalue λ. The λ- eigenspace is the subspace Vλ = f~v 2 V j T (~v) = λ~vg: Its dimension is called the geometric multiplicity of the eigenvalue λ of T . The kernel of T is the 0-eigenspace of T . The geometric multiplicity of 0 is the nullity of T . T Example 2.0.3. The map R2×2 −! R2×2 sending A to A + AT is a linear map. Note that if A is symmetric, then T (A) = 2A, so the set of symmetric matrices is contained in the 2-eigenspace. Conversely, if T (A) = 2A; then A + AT = 2A, so A = AT and A is symmetric. Thus the 2-eigenspace of T is precisely the subspace of symmetric matrices. This space is dimension 3, with basis (E11;E22;E12 + E21). Are there any other eigenvalues and vectors? 0 1 Well, the kernel is the zero-eigenspace. The matrix can be easily seen to span the −1 0 kernel, hence it is a basis for the zero-eigenspace. So B = (E11;E22;E12 + E21;E12 − E21) 22 0 0 03 60 2 0 07 is an eigenbasis for T . The corresponding diagonal B-matrix is [T ]B = 6 7 : This 40 0 2 05 0 0 0 0 transformation has two eigenspaces. The 2-eigenspace is the space of symmetric matrices. The 0-vector space is the subspace of skew-symmetric matrices. Note that the geometric multiplicity of the eigenvalue 2 is 3 and geometric multiplicity of the eigenvalue 0 is 1. Remark 2.0.4. We often abuse terminology, referring to "eigenvalues and eigenvectors of a matrix"—this means the eigenvalues and eigenvectors of the corresponding map Rn −! Rn given by left multiplication by A. Accordingly, the eigenvalues of A are the scalars λ for which there exists a non-zero column vector ~v such that A~v = λ~v: The set of all such column vectors ~v is the λ-eigenspace of A. 3 3. Finding Eigenvalues and Eigenvectors. Definition 3.0.1. Consider an n × n matrix A. The characteristic polynomial of A is the degree n polynomial χA(x) = det(xIn − A): 0 −1 Example 3.0.2. Let A = . Then the characteristic polynomial of A is the deter- 1 0 x 1 minant of the matrix . Thus χ (x) = x2 + 1. −1 x A Lemma 3.0.3. Similar matrices have the same characteristic polynomial. That is, if A and B are n × n matrices for which there exists an n × n matrix S with B = S−1AS; then χA(x) = χB(x). Lemma 3.0.3 ensures that the following definition makes sense: Definition 3.0.4. Let V −!T V be a linear transformation on a vector space V of finite dimension n. The characteristic polynomial of T is the degree n polynomial χT (x) = det(xIn − A) where A is the matrix of T in any basis for V . Theorem 3.0.5. Let V −!T V be a linear transformation on a finite dimensional vector space. The eigenvalues of T are precisely the roots of the characteristic polynomial of T . Proof. Fix a scalar c. Consider the linear transformation2 V −!Φ V ~v 7! T (~v) − c~v: Note that c is an eigenvalue if and only if this transformation Φ has a non-zero kernel. By rank-nullity, Φ has a non-zero kernel if and only if it is not invertible, which happens if and only if the determinant of Φ is zero. We can compute the determinant of Φ by computing the determinant of its B for any basis. Fix any basis B for V . We compute the B-basis for Φ by thinking of Φ as T − cIV , where IV is the identity map on V . Let A be the B-matrix of T . Note that the B-matrix of cIV is cIn (where n = dim V ). So [Φ]B = [T − cIV ]B = [T ]B − [cIV ]B = A − cIn: Its determinant is precisely the result of plugging c into the polynomial det(A − xIn). Of course, det(A − xIn) is n n det(−1)(xIn − A) = (−1) det(xIn − A) = (−1) χT (x): This means that c is an eigenvalue if and only if c is a root of the characteristic polynomial. 2As a good reader of mathematics, you realize you should check yourself that the given map is really a linear transformation 4 Corollary 3.0.6.
Details
-
File Typepdf
-
Upload Time-
-
Content LanguagesEnglish
-
Upload UserAnonymous/Not logged-in
-
File Pages8 Page
-
File Size-