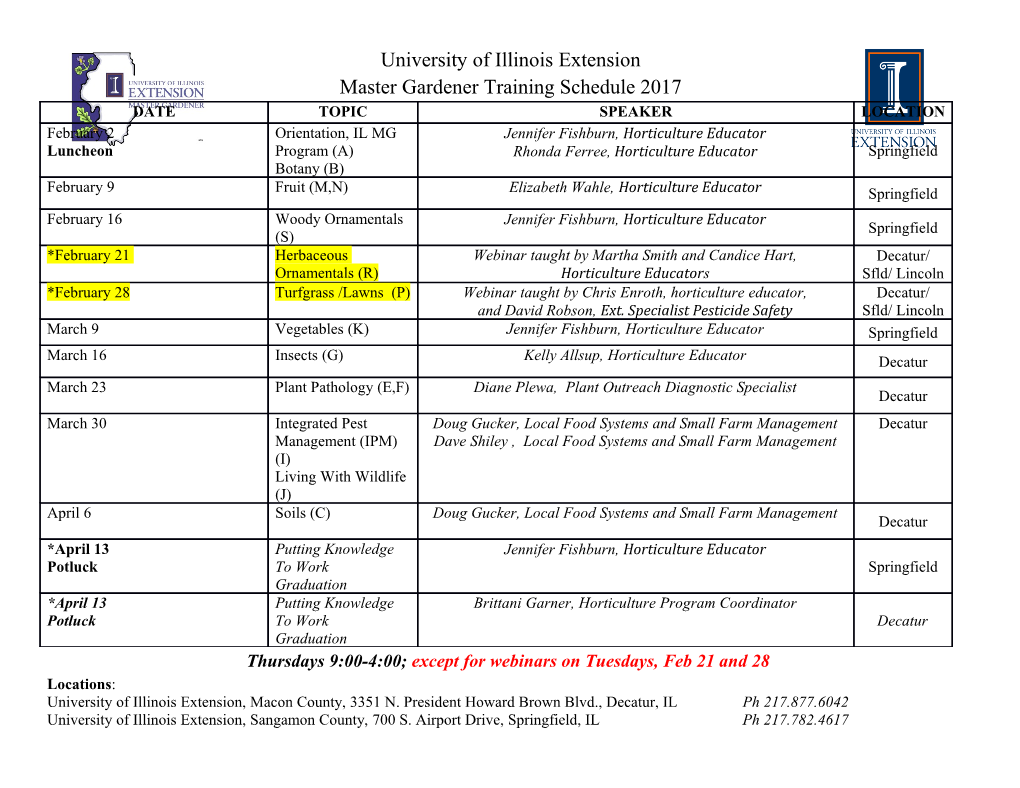
Numerical Multilinear Algebra I Lek-Heng Lim University of California, Berkeley January 5{7, 2009 L.-H. Lim (ICM Lecture) Numerical Multilinear Algebra I January 5{7, 2009 1 / 55 Hope Past 50 years, Numerical Linear Algebra played indispensable role in the statistical analysis of two-way data, the numerical solution of partial differential equations arising from vector fields, the numerical solution of second-order optimization methods. Next step | development of Numerical Multilinear Algebra for the statistical analysis of multi-way data, the numerical solution of partial differential equations arising from tensor fields, the numerical solution of higher-order optimization methods. L.-H. Lim (ICM Lecture) Numerical Multilinear Algebra I January 5{7, 2009 2 / 55 DARPA mathematical challenge eight One of the twenty three mathematical challenges announced at DARPA Tech 2007. Problem Beyond convex optimization: can linear algebra be replaced by algebraic geometry in a systematic way? Algebraic geometry in a slogan: polynomials are to algebraic geometry what matrices are to linear algebra. Polynomial f 2 R[x1;:::; xn] of degree d can be expressed as > > f (x) = a0 + a1 x + x A2x + A3(x; x; x) + ··· + Ad (x;:::; x): n n×n n×n×n n×···×n a0 2 R; a1 2 R ; A2 2 R ; A3 2 R ;:::; Ad 2 R . Numerical linear algebra: d = 2. Numerical multilinear algebra: d > 2. L.-H. Lim (ICM Lecture) Numerical Multilinear Algebra I January 5{7, 2009 3 / 55 Motivation Why multilinear: \Classification of mathematical problems as linear and nonlinear is like classification of the Universe as bananas and non-bananas." Nonlinear | too general. Multilinear | next natural step. Why numerical: Different from Computer Algebra. Numerical rather than symbolic: floating point operations | cheap and abundant; symbolic operations | expensive. Like other areas in numerical analysis, will entail the approximate solution of approximate multilinear problems with approximate data but under controllable and rigorous confidence bounds on the errors involved. L.-H. Lim (ICM Lecture) Numerical Multilinear Algebra I January 5{7, 2009 4 / 55 Tensors: mathematician's definition U; V ; W vector spaces. Think of U ⊗ V ⊗ W as the vector space of all formal linear combinations of terms of the form u ⊗ v ⊗ w, X αu ⊗ v ⊗ w; where α 2 R; u 2 U; v 2 V ; w 2 W . One condition: ⊗ decreed to have the multilinear property (αu1 + βu2) ⊗ v ⊗ w = αu1 ⊗ v ⊗ w + βu2 ⊗ v ⊗ w; u ⊗ (αv1 + βv2) ⊗ w = αu ⊗ v1 ⊗ w + βu ⊗ v2 ⊗ w; u ⊗ v ⊗ (αw1 + βw2) = αu ⊗ v ⊗ w1 + βu ⊗ v ⊗ w2: Up to a choice of bases on U; V ; W , A 2 U ⊗ V ⊗ W can be l;m;n l×m×n represented by a 3-hypermatrix A = aijk i;j;k=1 2 R . J K L.-H. Lim (ICM Lecture) Numerical Multilinear Algebra I January 5{7, 2009 5 / 55 Tensors: physicist's definition \What are tensors?" ≡ \What kind of physical quantities can be represented by tensors?" Usual answer: if they satisfy some `transformation rules' under a change-of-coordinates. Theorem (Change-of-basis) Two representations A; A0 of A in different bases are related by (L; M; N) · A = A0 with L; M; N respective change-of-basis matrices (non-singular). Pitfall: tensor fields (roughly, tensor-valued functions on manifolds) often referred to as tensors | stress tensor, piezoelectric tensor, moment-of-inertia tensor, gravitational field tensor, metric tensor, curvature tensor. L.-H. Lim (ICM Lecture) Numerical Multilinear Algebra I January 5{7, 2009 6 / 55 Tensors: data analyst's definition l;m;n l×m×n Data structure: k-array A = aijk i;j;k=1 2 R J K Algebraic structure: l×m×n 1 Addition/scalar multiplication: for bijk 2 R , λ 2 R, J K l×m×n aijk + bijk := aijk + bijk and λ aijk := λaijk 2 R J K J K J K J K J K 2 Multilinear matrix multiplication: for matrices p×l q×m r×n L = [λi 0i ] 2 R ; M = [µj0j ] 2 R ; N = [νk0k ] 2 R , p×q×r (L; M; N) · A := ci 0j0k0 2 R J K where Xl Xm Xn ci 0j0k0 := λi 0i µj0j νk0k aijk : i=1 j=1 k=1 Think of A as 3-dimensional hypermatrix.(L; M; N) · A as multiplication on `3 sides' by matrices L; M; N. Generalizes to arbitrary order k. If k = 2, ie. matrix, then (M; N) · A = MANT. L.-H. Lim (ICM Lecture) Numerical Multilinear Algebra I January 5{7, 2009 7 / 55 Hypermatrices Totally ordered finite sets: [n] = f1 < 2 < ··· < ng, n 2 N. Vector or n-tuple f :[n] ! R: > n If f (i) = ai , then f is represented by a = [a1;:::; an] 2 R . Matrix f :[m] × [n] ! R: m;n m×n If f (i; j) = aij , then f is represented by A = [aij ]i;j=1 2 R . Hypermatrix (order 3) f :[l] × [m] × [n] ! R: l;m;n l×m×n If f (i; j; k) = aijk , then f is represented by A = aijk i;j;k=1 2 R . J K X [n] [m]×[n] [l]×[m]×[n] Normally R = ff : X ! Rg. Ought to be R ; R ; R . L.-H. Lim (ICM Lecture) Numerical Multilinear Algebra I January 5{7, 2009 8 / 55 Hypermatrices and tensors Up to choice of bases n a 2 R can represent a vector in V (contravariant) or a linear functional in V ∗ (covariant). m×n ∗ ∗ A 2 R can represent a bilinear form V × W ! R (contravariant), a bilinear form V × W ! R (covariant), or a linear operator V ! W (mixed). l×m×n A 2 R can represent trilinear form U × V × W ! R (covariant), bilinear operators V × W ! U (mixed), etc. A hypermatrix is the same as a tensor if 1 we give it coordinates (represent with respect to some bases); 2 we ignore covariance and contravariance. L.-H. Lim (ICM Lecture) Numerical Multilinear Algebra I January 5{7, 2009 9 / 55 Basic operation on a hypermatrix m×n A matrix can be multiplied on the left and right: A 2 R , p×m q×n X 2 R , Y 2 R , > p×q (X ; Y ) · A = XAY = [cαβ] 2 R where Xm;n cαβ = xαi yβj aij : i;j=1 l×m×n A hypermatrix can be multiplied on three sides: A = aijk 2 R , p×l q×m r×n J K X 2 R , Y 2 R , Z 2 R , p×q×r (X ; Y ; Z) ·A = cαβγ 2 R J K where Xl;m;n cαβγ = xαi yβj zγk aijk : i;j;k=1 L.-H. Lim (ICM Lecture) Numerical Multilinear Algebra I January 5{7, 2009 10 / 55 Basic operation on a hypermatrix Covariant version: A· (X >; Y >; Z >) := (X ; Y ; Z) ·A: Gives convenient notations for multilinear functionals and multilinear l m n operators. For x 2 R ; y 2 R ; z 2 R , Xl;m;n A(x; y; z) := A· (x; y; z) = aijk xi yj zk ; i;j;k=1 Xm;n A(I ; y; z) := A· (I ; y; z) = aijk yj zk : j;k=1 L.-H. Lim (ICM Lecture) Numerical Multilinear Algebra I January 5{7, 2009 11 / 55 Segre outer product l m n l m n If U = R , V = R , W = R , R ⊗ R ⊗ R may be identified with l×m×n R if we define ⊗ by l;m;n u ⊗ v ⊗ w = ui vj wk i;j;k=1: J K l×m×n A tensor A 2 R is said to be decomposable if it can be written in the form A = u ⊗ v ⊗ w l m n for some u 2 R ; v 2 R ; w 2 R . The set of all decomposable tensors is known as the Segre variety in algebraic geometry. It is a closed set (in both the Euclidean and Zariski sense) as it can be described algebraically: l m n l×m×n Seg(R ; R ; R ) = fA 2 R j ai1i2i3 aj1j2j3 = ak1k2k3 al1l2l3 ; fiα; jαg = fkα; lαgg L.-H. Lim (ICM Lecture) Numerical Multilinear Algebra I January 5{7, 2009 12 / 55 Symmetric hypermatrices n×n×n Cubical hypermatrix aijk 2 R is symmetric if J K aijk = aikj = ajik = ajki = akij = akji : Invariant under all permutations σ 2 Sk on indices. k n S (R ) denotes set of all order-k symmetric hypermatrices. Example Higher order derivatives of multivariate functions. Example Moments of a random vector x = (X1;:::; Xn): »Z Z –n ˆ ˜n mk (x) = E(xi1 xi2 ··· xik ) = ··· xi1 xi2 ··· xik dµ(xi1 ) ··· dµ(xik ) : i1;:::;ik =1 i1;:::;ik =1 L.-H. Lim (ICM Lecture) Numerical Multilinear Algebra I January 5{7, 2009 13 / 55 Symmetric hypermatrices Example Cumulants of a random vector x = (X1;:::; Xn): 2 3n „ « „ « X p−1 Q Q κk (x) = 4 (−1) (p − 1)!E xi ··· E xi 5 : i2A1 i2Ap A1t···tAp =fi1;:::;ik g i1;:::;ik =1 For n = 1, κk (x) for k = 1; 2; 3; 4 are the expectation, variance, skewness, and kurtosis. Important in Independent Component Analysis (ICA). L.-H. Lim (ICM Lecture) Numerical Multilinear Algebra I January 5{7, 2009 14 / 55 Inner products and norms 2 n > Pn ` ([n]): a; b 2 R , ha; bi = a b = i=1 ai bi . 2 m×n > Pm;n ` ([m] × [n]): A; B 2 R , hA; Bi = tr(A B) = i;j=1 aij bij . 2 l×m×n Pl;m;n ` ([l] × [m] × [n]): A; B 2 R , hA; Bi = i;j;k=1 aijk bijk . In general, `2([m] × [n]) = `2([m]) ⊗ `2([n]); `2([l] × [m] × [n]) = `2([l]) ⊗ `2([m]) ⊗ `2([n]): Frobenius norm Xl;m;n kAk2 = a2 : F i;j;k=1 ijk Norm topology often more directly relevant to engineering applications than Zariski toplogy.
Details
-
File Typepdf
-
Upload Time-
-
Content LanguagesEnglish
-
Upload UserAnonymous/Not logged-in
-
File Pages37 Page
-
File Size-