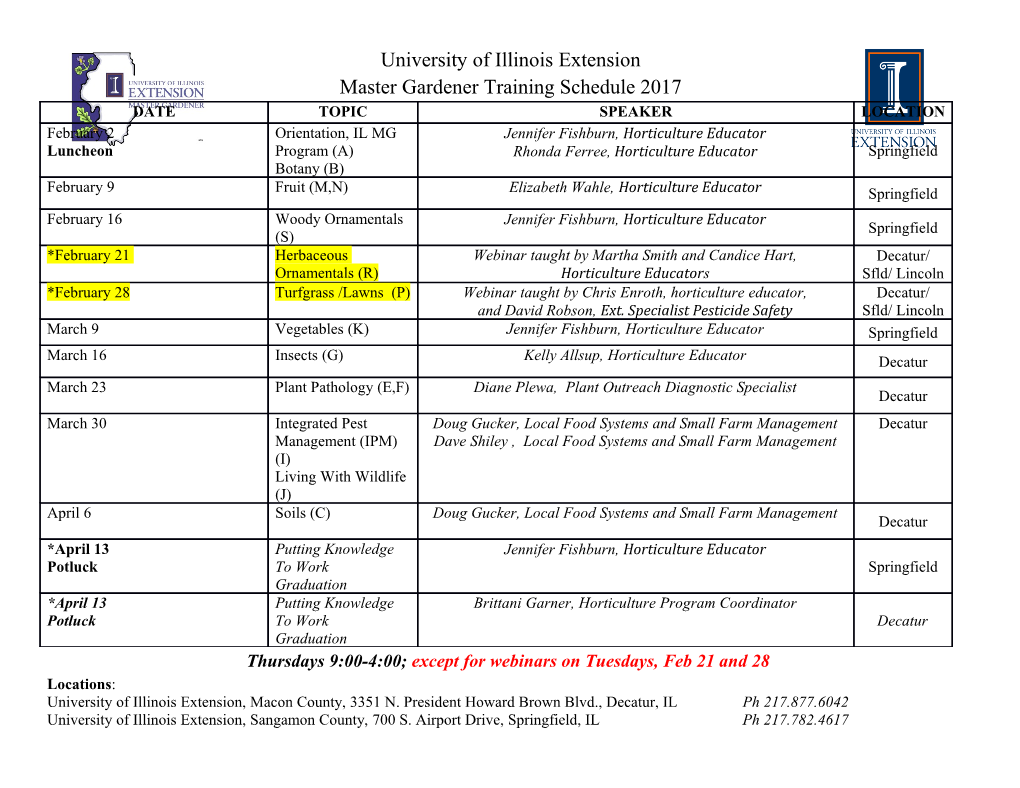
PHYSTAT-LHC Workshop June 27-29, 2007 ' $ Bayesian analysis: objectivity, multiplicity and discovery James O. Berger Duke University Statistical and Applied Mathematical Sciences Institute & %1 PHYSTAT-LHC Workshop June 27-29, 2007 ' $ Relevant SAMSI Programs • Astrostatistics/Phystat Program: January – July, 2006 (Jogesh Babu and Louis Lyons were Program Leaders) • Multiplicity and Reproducibility in Scientific Studies: July 10-28, 2006 • Future program on Discovery or ?. & %2 PHYSTAT-LHC Workshop June 27-29, 2007 ' $ Outline • Objective Bayesian Analysis • Bayesian Hypothesis Testing • Multiplicity and Discovery & %3 PHYSTAT-LHC Workshop June 27-29, 2007 ' Objective Bayesian Analysis $ • In subjective Bayesian analysis, a prior distribution π(θ) for an unknown θ represents personal beliefs or knowledge. – But: If a prior distribution reflects consensus belief of science, should we call that subjective or objective? • In objective Bayesian analysis, prior distributions represent ‘neutral’ knowledge. Many types: – Maximum entropy – Minimum description length (or minimum message length) – Jeffreys / Reference – Uniform in local-location parameterizations – Right-Haar and left-Haar (structural) – Fiducial distributions and other specific invariance – Matching priors – Admissible priors & %4 PHYSTAT-LHC Workshop June 27-29, 2007 ' $ Why Objective Bayes? • It is the oldest and most used form of Bayesianism (Laplace,...) • Some believe it to be the ultimate inferential truth (e.g. Jaynes), or at least the correct answer to precise definitions of ‘letting the data speak for itself.’ • Good versions are argued to yield better frequentist answers than asymptotic frequentist methods. • Numerous difficult problems, such as dealing with multiplicity, become straightforward to handle. • One is automatically solving the otherwise very difficult problem of proper conditioning; in this community called ‘Bayesian credibility’ - Joel’s, Eilam’s, and Paul’s talks. & %5 PHYSTAT-LHC Workshop June 27-29, 2007 'Artificial example of coverage and ‘Bayesian credibility’: $ Observe X1 and X2 where θ + 1 with probability 1/2 Xi = θ − 1 with probability 1/2. Consider the confidence set (a singleton) for θ 1 2 (X1 + X2) if X1 6= X2 C(X1,X2)= X1 − 1 if X1 = X2. Unconditional coverage: Pθ(C(X1,X2) contains θ) = 0.75. Bayesian credibility: If one uses the objective prior π(θ)=1, C(X1,X2) has Bayesian credibility of 50% if x1 6= x2 and 100% if x1 = x2, which is obviously the right answer in this example. The point: Unconditional frequentistism can be practically silly for particular data in the absence of ‘Bayesian credibility.’ & %6 PHYSTAT-LHC Workshop June 27-29, 2007 ' $ Comments on Bayesian Credibility • Is it the case that coverage errors are more important than credibility errors? (Harrison’s point) • What priors should be chosen for assessing credibility? – Ideally, credibility would be measured by a prior that is nearly exactly frequentist matching (although then the ‘right answer’ is known). – Alternatively, one might choose a reasonable class of priors and compute the credibility range over the class (robust Bayesian analysis). & %7 PHYSTAT-LHC Workshop June 27-29, 2007 ' $ A psychiatry diagnosis example (with Mossman, 2001) The Psychiatry Problem: • Within a population, p0 = Pr(Disease D). • A diagnosic test results in either a Positive (P) or Negative (N) reading. • p1 = Pr(P | patient has D). • p2 = Pr(P | patient does not have D). • It follows from Bayes theorem that p p θ ≡ Pr(D|P )= 0 1 . p0p1 + (1 − p0)p2 & %8 PHYSTAT-LHC Workshop June 27-29, 2007 ' $ The Statistical Problem: The pi are unknown. Based on (independent) data Xi ∼ Binomial(ni,pi) (arising from medical surveys of ni individuals), find a 100(1 − α)% confidence set for θ. Suggested Solution: Assign pi the Jeffreys-rule objective prior −1/2 −1/2 π(pi) ∝ pi (1 − pi) (not the full reference prior!). By Bayes theorem, the posterior distribution of pi given the data, xi, is −1/2 −1/2 n xi ni−xi p (1 − pi) × p (1 − pi) π(p | x )= i xi i , i i −1/2 n x p (1 − p )−1/2 × p i (1 − p )ni−xi dp i i xi i i i R 1 1 which is the Beta(xi + 2 , ni − xi + 2 ) distribution. & %9 PHYSTAT-LHC Workshop June 27-29, 2007 ' $ Finally, compute the desired confidence set (formally, the 100(1 − α)% equal-tailed posterior credible set) through Monte Carlo simulation from the posterior distribution by 1 1 • drawing random pi from the Beta(xi + 2 , ni − xi + 2 ) posterior distributions, i = 0, 1, 2; • computing the associated θ = p0p1/[p0p1 + (1 − p0)p2]; • repeating this process 10, 000 times, yielding θ1,θ2,...,θ10,000; α • using the 2 % upper and lower percentiles of these generated θ to form the desired confidence limits. & %10 PHYSTAT-LHC Workshop June 27-29, 2007 ' $ n0 = n1 = n2 (x0, x1, x2) 95% confidence interval 20 (2,18,2) (0.107, 0.872) 20 (10,18,0) (0.857, 1.000) 80 (20,60,20) (0.346, 0.658) 80 (40,72,8) (0.808, 0.952) Table 1: The 95% equal-tailed posterior credible interval for θ = p0p1/[p0p1 + (1 − p0)p2], for various values of the ni and xi. & %11 PHYSTAT-LHC Workshop June 27-29, 2007 'Unconditional frequentist performance of the objective Bayes $ procedure: The goal was to find confidence sets for p p θ = Pr(D P )= 0 1 . | p0p1 + (1 p0)p2 − Consider the frequentist percentage of the time that the 95% Bayesian sets miss on the left and on the right (ideal would be 2.5%) for the indicated parameter values when n0 = n1 = n2 = 20. (p0,p1,p2) O-Bayes Log Odds Gart-Nam Delta 1 3 1 ( 4 , 4 , 4 ) 2.86,2.71 1.53,1.55 2.77,2.57 2.68,2.45 1 9 1 ( 10 , 10 , 10 ) 2.23,2.47 0.17,0.03 1.58,2.14 0.83,0.41 1 9 1 ( 2 , 10 , 10 ) 2.81,2.40 0.04,4.40 2.40,2.12 1.25,1.91 Conclusion: By construction, reasonable ‘Bayesian credibility’ is guaranteed; unconditional frequentist performance is clearly fine (and the expected lengths of the Bayesian intervals were smallest). & %12 PHYSTAT-LHC Workshop June 27-29, 2007 'What is Frequentism in the Basic HEP Problem? $ Model: Ns+b ∼ Poisson(Ns+b | s + b), where s is the unknown signal mean and b the unknown background mean. Goal: Upper confidence limit for s. Proper prior density for b: π(b), arising from either • Case 1: sideband data Nb ∼ Poisson(Nb | b) • Case 2: known physical randomness • Case 3: agreed scientific beliefs Objective prior density for s: πo(s | b) Bayesian analysis: construct upper confidence limit U for s from the posterior distribution π(s | N ) ∝ Poisson(N | s + b)πo(s | b)π(b)db. s+b Z s+b & %13 PHYSTAT-LHC Workshop June 27-29, 2007 ' o $ Case 1: With the sideband data Nb, π(b) ∝ Poisson(Nb | b)π (b), where πo(b) is an objective prior. Natural frequentist goal: Frequentist coverage w.r.t the joint distribution of Ns+b and Nb, i.e. P (s < U(Ns+b, Nb) s, b) | ∞ ∞ X X = 1 Poisson(Ns+b s + b)Poisson(Nb b) . {s<U(Ns+b,Nb)} | | Ns+b=0 Nb=0 Objective Bayesian solution: Find a reference prior πo(s,b) (see Luc’s work), that has excellent frequentist coverage properties (except possibly at the boundaries). If this is too hard, find adhoc objective priors that work; also hard, alas. But achieving ‘Bayesian credibility’ is at least as hard, since a frequentist must establish this for all possible data. & %14 PHYSTAT-LHC Workshop June 27-29, 2007 'Case 2: π(b) describes the physical randomness of the (otherwise $ unmeasured) background from experiment to experiment. Natural frequentist goal: Frequentist coverage w.r.t. the marginal density Ê f(Ns+b s)= Poisson(Ns+b s + b)π(b)db, i.e., coverage as | | ∞ X P (s < U(Ns+b) s)= 1 f(Ns+b s) . | {s<U(Ns+b)} | Ns+b=0 Frequentist Principle (Neyman): In repeated actual use of a statistical procedure, the average actual error should not be greater than (and should ideally equal) the average reported error. Objective Bayesian solution: Find the reference prior corresponding to f(Ns+b s), which is the Jeffreys prior | ∞ X 2 J Ô d π (s)= I(s) , I(s)= f(Ns+b s) log f(Ns+b s) . − | ds2 | Ns+b=0 This should have excellent frequentist coverage properties (except possibly at the boundary s = 0). & %15 PHYSTAT-LHC Workshop June 27-29, 2007 'Case 3: π(b) encodes accepted scientific beliefs. $ Natural frequentist goal: Unclear! One could • insist that, for every given s and b, we control ∞ P (s<U(Ns+b) | s,b)= 1{s<U(Ns+b)}Poisson(Ns+b | s+b) NsX+b=0 (actually not possible here, but let’s pretend it is); • again simply control coverage w.r.t. f(Ns+b | s), i.e. ∞ P (s<U(Ns+b) | s) = 1{s<U(Ns+b)}f(Ns+b | s) NsX+b=0 = P (s<U(N ) | s,b)π(b)db. Z s+b Objective Bayesian solution: None for the first criterion; indeed, what does a frequentist do with π(b)? Second criterion: as before. & %16 PHYSTAT-LHC Workshop June 27-29, 2007 ' Bayesian Hypothesis Testing $ Key issue 1: Is the hypothesis being tested believable? Example: Test of H0 : s = 0, where s is the mean signal. Here s = 0 should be plausible (e.g., no Higgs). Key issue 2: There is no need to assign prior probabilities to hypotheses; one can give Bayes factors, and sometimes useful bounds on Bayes factors that are completely independent of priors.
Details
-
File Typepdf
-
Upload Time-
-
Content LanguagesEnglish
-
Upload UserAnonymous/Not logged-in
-
File Pages35 Page
-
File Size-