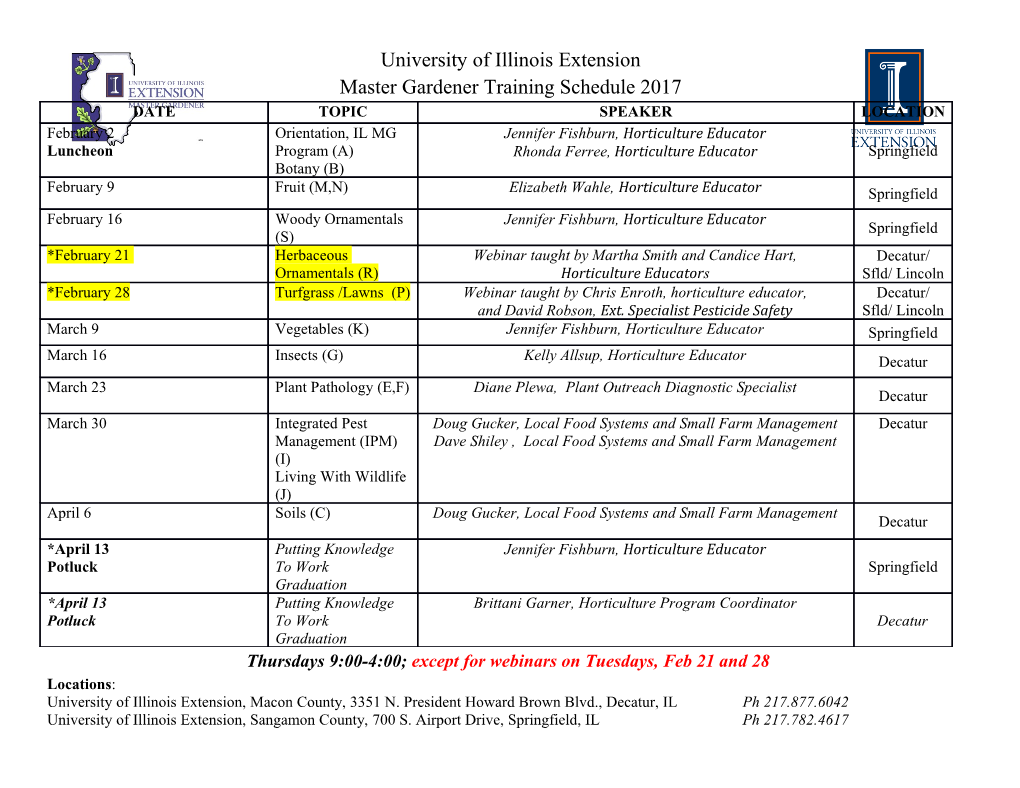
Wilop et al. Journal of Hematology & Oncology (2016) 9:78 DOI 10.1186/s13045-016-0308-8 RESEARCH Open Access A three-gene expression-based risk score can refine the European LeukemiaNet AML classification Stefan Wilop1, Wen-Chien Chou2,3, Edgar Jost1, Martina Crysandt1, Jens Panse1, Ming-Kai Chuang2, Tim H. Brümmendorf1, Wolfgang Wagner4,5, Hwei-Fang Tien3 and Behzad Kharabi Masouleh1* Abstract Background: Risk stratification based on cytogenetics of acute myeloid leukemia (AML) remains imprecise. The introduction of novel genetic and epigenetic markers has helped to close this gap and increased the specificity of risk stratification, although most studies have been conducted in specific AML subpopulations. In order to overcome this limitation, we used a genome-wide approach in multiple AML populations to develop a robust prediction model for AML survival. Methods: We conducted a genome-wide expression analysis of two data sets from AML patients enrolled into the AMLCG-1999 trial and from the Tumor Cancer Genome Atlas (TCGA) to develop a prognostic score to refine current risk classification and performed a validation on two data sets of the National Taiwan University Hospital (NTUH) and an independent AMLCG cohort. Results: In our training set, using a stringent multi-step approach, we identified a small three-gene prognostic scoring system, named Tri-AML score (TriAS) which highly correlated with overall survival (OS). Multivariate analysis revealed TriAS to be an independent prognostic factor in all tested training and additional validation sets, even including age, current cytogenetic-based risk stratification, and three other recently developed expression-based scoring models for AML. Conclusions: The Tri-AML score allows robust and clinically practical risk stratification for the outcome of AML patients. TriAS substantially refined current ELN risk stratification assigning 44.5 % of the patients into a different risk category. Abbreviations: AML, Acute myeloid leukemia; TCGA, Tumor Cancer Genome Atlas; NTUH, National Taiwan University Hospital; TriAS, Tri-AML score; CN-AML, Cytogenetically normal AML; FDR, False discovery rate; GO, Gene ontology; RFS, Relapse-free survival; pts, Points; OS, Overall survival; ELN, European LeukemiaNet; HCC, Hepatocellular carcinoma; ALL, Acute lymphoblastic leukemia Key points Background The biological heterogeneity of acute myeloid leukemia TriAS improves risk stratification in AML (AML) in combination with patient-related risk factors TriAS is robust in multivariate analysis compared to such as age or co-morbidities result in a wide range of established risk factors clinical outcomes making it a continuous challenge for clinicians to assess individual patients’ risk. Currently applied risk-prognostication models mainly rely on a combination of pre-treatment karyotype and molecular mutations. Recent improvements have been made in prog- * Correspondence: [email protected] 1Department of Hematology, Oncology, Hemostaseology and Stem Cell nostication, e.g., by adding individual molecular markers Transplantation, Medical Faculty, RWTH Aachen University, Aachen, Germany to conventional cytogenetics—particularly in patients with Full list of author information is available at the end of the article © 2016 The Author(s). Open Access This article is distributed under the terms of the Creative Commons Attribution 4.0 International License (http://creativecommons.org/licenses/by/4.0/), which permits unrestricted use, distribution, and reproduction in any medium, provided you give appropriate credit to the original author(s) and the source, provide a link to the Creative Commons license, and indicate if changes were made. The Creative Commons Public Domain Dedication waiver (http://creativecommons.org/publicdomain/zero/1.0/) applies to the data made available in this article, unless otherwise stated. Wilop et al. Journal of Hematology & Oncology (2016) 9:78 Page 2 of 10 normal karyotype AML. The large variability of outcomes model of each training subset. Selection of those genes within these individual risk groups suggests that more with significant impact on OS in uni- and multivariate ana- sophisticated approaches including epigenetics [1, 2], lysis including age in both subsets of the training set with microRNA [3], or scoring models based on individual the same effect direction and expression of the transcripts genes [4, 5] are required to provide a more personalized in all patient samples led to a candidate list of 30 genes. risk assessment. While these studies represent a great leap forward, several of these studies contain certain limita- Development of expression-based scoring models tions, often analyzing only a specific AML subset [3, 5], In order to systematically evaluate the prediction ability such as cytogenetically normal AML (CN-AML), which in the combined training set (TCGA + GSE570) while only counts for 40 to 50 % of adult and 25 % of pediatric reducing the number of genes in our model, we calculated AML patients [6, 7]. each best predicting combination of n =30,n =29… down In this regard, improved risk stratification is still an to n = 1 genes using multivariate Cox regression analysis unmet clinical need also in elderly AML patients with still keeping age >65 years and the cytogenetic risk group poor long-term overall survival (OS) [8]. In order to over- within the model. The model with the highest likelihood come some of these limitations, we used an unbiased score was identified using the branch-and-bound algo- genome-wide approach to identify reliable genetic markers rithm of Furnival and Wilson as included in the SAS and developed a prognostic scoring system named Tri- software package version 9.3. AML score (TriAS). An individual score was then created for each n-gene- combination: For each of the n-genes included in the Methods score, 1 point was added if expression of the gene was Patients and treatment above the median expression of the data set in case of In total, four data sets were used in this study. Two inde- genes with a hazard ratio >1 in the multivariate n-gene- pendent data sets comprising of total 242 patients served model, whereas 1 point was subtracted in case of a hazard as training sets, including 163 patients from the TCGA ratio <1 in the multivariate n-gene-model. portal investigated using RNAseq technology [9] and 79 patients from which 62 were enrolled in the German Gene ontology studies AML Cooperative Group (AMLCG) 1999 trial [10], while Gene ontology studies were analyzed using the software 17 had received therapy outside of the trial [4] using the platform Cytoscape (Version 3.2.1) [12] and the plugin Affymetrix 133 Plus 2.0 platform (GSE12417-GPL570). BiNGO (Version 2.4.4) [13]. Testing for significant path- Two additional independent validation sets were derived way enrichment in BiNGO was performed using hypergeo- from either 227 patients at the National Taiwan University metric distribution testing and multiple testing correction Hospital (NTUH) [11] (validation set 1) using the Illumina by Benjamini & Hochberg False Discovery Rate (FDR) with HumanHT-12 v4 Expression BeadChip platform as well as a significance cutoff value of p = 0.05. a second set derived from additional 163 patients enrolled in the AMLCG 1999 trial (GSE12417-GPL96A and B, Computing previously published AML scores (Marcucci validation set 2) using the Affymetrix 133 Plus 2.0 plat- et al., Chuang et al., Li et al.) form. Clinical characteristics and survival endpoints were For each data set, three recently published expression- used as described in the individual gene expression data based AML scores have been additionally calculated as sets [4, 9, 11]. Cytogenetic risk groups were available for previously described: the dichotomized Marcucci score [5], all data sets, even though the AMLCG data set included the continuous Chuang score[11], and the dichotomized Li CN-AML patients only. score [14]. Identification of prognostic genes Statistical analysis We used a multi-step approach in order to identify the Gene expression data of each patient was dichotomized most reliable combination of expression-based markers based either on a higher (GeneHI) or lower (GeneLOW) (Fig. 1). In order to facilitate generation and validation of a expression value compared to the median of the cohort of score, only transcripts were included in the analysis where the individual data set as a cutoff. For the identification of the corresponding gene was available in all four data sets. prognostic genes, univariate Cox regression analysis of First, univariate Cox regression analysis using the dicho- overall survival was performed for each single gene using tomized expression (higher or lower compared to the the dichotomized expression of each gene. The median median of the corresponding data set) was conducted to expression for each gene of each microarray data set identify all genes with significant impact on OS in the served as the cutoff between high and low expression. training sets (TCGA and GSE570). Next, age was included Additionally, multivariate Cox regression was performed as a confounding factor into a multivariate Cox regression including the dichotomized expression and age. Wilop et al. Journal of Hematology & Oncology (2016) 9:78 Page 3 of 10 Training set Validation set 1 Validation set 2 163 AML patients 79 AML patients 224 AML patients 163 AML patients TCGA data set Metzeler GSE12417-GPL570 Chuang HumanHT-12 v4 Metzeler GSE12417-GLP96 54.675 sequences 47.162 sequences 44.759
Details
-
File Typepdf
-
Upload Time-
-
Content LanguagesEnglish
-
Upload UserAnonymous/Not logged-in
-
File Pages10 Page
-
File Size-