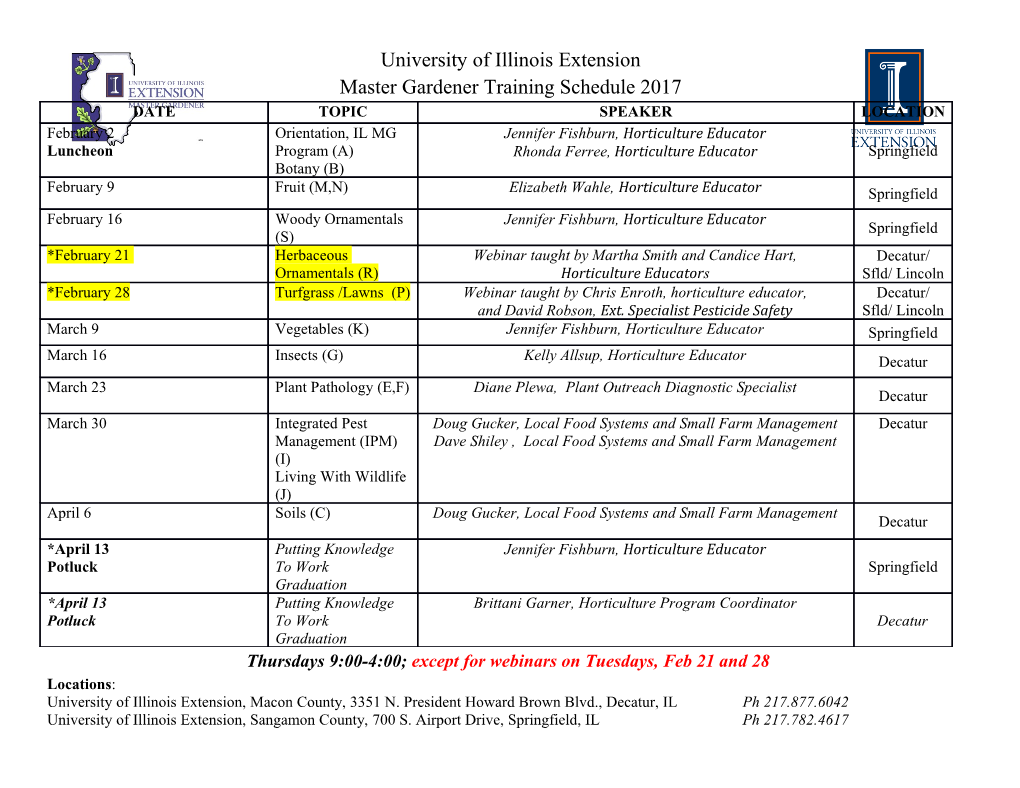
Polynomial Interpolation MAT 2310 Computational Mathematics Wm C Bauldry November, 2011 ASU-MSD 1 Contents 1. Polynomial Interpolation 2. Lagrange Interpolation 3. Interlude: Bernstein Polynomials 4. Newton Interpolation 5. Two Comparisons 6. Interlude: Splines 7. Exercises 8. Links and Others ASU-MSD 2 Polynomial Interpolation What is Polynomial Interpolation? An interpolating polynomial p(x) for a set of points is a polynomial S that goes through each point of . That is, for each point Pi = (xi; yi) S in the set, p(xi) = yi. Applications include: Approximating Functions TrueType Fonts (2nd deg) Fast Multiplication Cryptography PostScript Fonts (3rd deg) Data Compression Since each data point determines one polynomial coefficient, an n-point data set has an n 1 degree interpolating polynomial. − [ 2, 1] − [ 1, 1] 2 4 8 2 8 − − 9 p4(x)= x x +1 = > [0, 1] > 3 − 3 S > > <> [1, 1] => [2,−1] > > > > :> ;> ASU-MSD 3 Free Sample Finding an Interpolating Polynomial Let = [ 2; 1]; [ 1; 1]; [0; 1]; [1; 1]; [2; 1] . S f − − − − g 1. Since has 5 points, we compute a 4th degree polynomial S 2 3 4 p4(x) = a0 + a1x + a2x + a3x + a4x 2. Substitute the values of into p4; write the results as a system of linear equations. S 2 3 2 3 2 3 2 3 1 a0 − 2a1 + 4a2 − 8a3 + 16a4 1 −2 4 −8 16 a0 6−17 6 a0 − a1 + a2 − a3 + a4 7 61 −1 1 −1 1 7 6a17 6 7 6 7 6 7 6 7 6 17 = 6 a0 7 = 61 0 0 0 0 7 6a27 6 7 6 7 6 7 6 7 4−15 4 a0 + a1 + a2 + a3 + a4 5 41 1 1 1 1 5 4a35 1 a0 + 2a1 + 4a2 + 8a3 + 16a4 1 2 4 8 16 a4 3. Solve with your favorite method: p (x) = 2 x4 8 x2 + 1 4 3 − 3 ASU-MSD 4 Towards a Better Way Definition Knots or Nodes: The x-values of the interpolation points. Lagrange Fundamental Polynomial: Given a set of n + 1 knots, define Y x − xk Li(x) = xi − xk k=0::n k 6= i x − x x − x x − x x − x = 0 × · · · × i−1 × i+1 × · · · × n xi − x0 xi − xi−1 xi − xi+1 xi − xn Lagrange Interpolating Polynomial: Given a set of n + 1 data points (xk; yk), define n X pn(x) = yk Lk(x) k=0 ASU-MSD 5 Sampling a Better Way Example (Lagrange Fundamental Polynomials) The set of knots [ 2; 1; 0; 1; 2] gives − − L (x) = x−(−2) x−(−1) x−0 x−1 x−2 0 −2−(−2) · −2−(−1) · −2−0 · −2−1 · −2−2 delete L (x) = x−(−2) x−(−1) x−0 x−1 x−2 1 −1−(−2) · −1−(−1) · −1−0 · −1−1 · −1−2 delete x−(−2) x−(−1) x−0 x−1 x−2 L2(x) = 0−(−2) 0−(−1) 0−0 0−1 0−2 · · delete · · x−(−2) x−(−1) x−0 x−1 x−2 L3(x) = 1−(−2) 1−(−1) 1−0 1−1 1−2 · · · delete · x−(−2) x−(−1) x−0 x−1 x−2 L4(x) = 2−(−2) 2−(−1) 2−0 2−1 2−2 · · · · delete ASU-MSD 6 Sampling a Better Way Example Let = [ 2; 1]; [ 1; 1]; [0; 1]; [1; 1]; [2; 1] . S f − − − − g We have [xk] = [ 2; 1; 0; 1; 2] and [yk] = [1; 1; 1; 1; 1]. Then 4 − − − − p4(x) = yk Lk(x). So k X=0 p (x) = (1) x+1 x−0 x−1 x−2 4 · −2+1 · −2−0 · −2−1 · −2−2 + ( 1) x+2 x−0 x−1 x−2 − · −1+2 · −1−0 · −1−1 · −1−2 + (1) x+2 x+1 x−1 x−2 · 0+2 · 0+1 · 0−1 · 0−2 + ( 1) x+2 x+1 x−0 x−2 − · 1+2 · 1+1 · 1−0 · 1−2 + (1) x+2 x+1 x−0 x−1 · 2+2 · 2+1 · 2−0 · 2−1 Then p (x) = 2 x4 8 x2 + 1. 4 3 − 3 ASU-MSD 7 An ‘Easier’ Lk Formula Compact Expressions Y x − xk For a set [xk] of n + 1 knots, we defined Li(x) = . This formula xi − xk k=0::n k 6= i is computationally intensive. n Y Set !(x) = (x − xk). k=0 1. The numerator of Li is !(x)=(x − xi) 2. The denominator of Li is !(x)=(x − xi) evaluated at xi. Rewrite as !(x) !(x) − !(xi) = . Take the limit as x ! xi: x − xi x − xi !(x) − !(xi) 0 lim = ! (xi) x!xi x − xi !(x) Thus Li(x) = 0 . A very compact formula! (x − xi) ! (xi) ASU-MSD 8 Properties of the Lk’s Proposition n X For the Lagrange interpolating polynomial pn(x) = ykLk(x), k=0 1. pn(x) is the unique polynomial of the nth degree s.t. p(xk) = yk. ( 1 j = k 2. Lk(xj ) = δkj = 0 j 6= k n X 3. Lk(x) = 1 k=0 4. If q(x) is a polynomial of degree ≤ n with yk = q(xk), then pn ≡ q 5. The set fLk(x): k = 0::(n − 1)g is a basis of Pn−1 Theorem (Lagrange Interpolation Error) n+1 If f 2 C [a; b] and fxkg 2 [a; b], then (b − a)n+1 " = jf(x) − p (x)j ≤ max jf (n+1)(x)j n n (n + 1)! ASU-MSD 9 Drawbacks More Knots To decrease the error, use more knots. But . all the Lk(x) change. 1. Set fxkg = {−2; 1; 2g. Then x − 1 x − 2 L (x) = · = 1 x2 − 1 x + 1 0 −2 − 1 −2 − 2 12 4 6 x + 2 x − 2 L (x) = · = − 1 x2 + 4 1 1 + 2 1 − 2 3 3 x + 2 x − 1 L (x) = · = 1 x2 + 1 x − 1 2 2 + 2 2 − 1 4 4 2 2. Set fxkg = {−2; −1; 1; 2g. Then x + 1 x − 1 x − 2 L (x) = · · = − 1 x3 + 1 x2 + 1 x − 1 0 −2 + 1 −2 − 1 −2 − 2 12 6 12 6 x + 2 x − 1 x − 2 L (x) = · · = 1 x3 − 1 x2 − 2 x + 2 1 −1 + 2 −1 − 1 −1 − 2 16 6 3 3 x + 2 x + 1 x − 2 L (x) = · · = − 1 x3 − 1 x2 + 2 x + 2 2 1 + 2 1 + 1 1 − 2 6 6 3 3 x + 2 x + 1 x − 1 L (x) = · · = 1 x3 + 1 x2 − 1 x − 1 3 2 + 2 2 + 1 2 − 1 12 6 12 6 ASU-MSD 10 Bernstein Polynomials Definition (Bernstein Polynomials of f) n k n−k Bernstein Basis Polynomials: bn;k(x) = x (1 x) for k = 0::n k − Bernstein Polynomial of f: Let f :[0; 1] R. Then n ! k n k n−k Bn(f) = f x (1 x) n k − k X=0 Note: If g :[a; b] R, then use f(x) = g a + (b a)x ! − Example 3 n k3 k k n−k Let f(x) = x for x [0; 1]. Then Bn(f) = 3 x (1 x) 2 k=0 n n − P1 3 2 B1(x) = x B2(x) = 4 x + 4 x 1 2 2 1 3 1 9 2 3 3 B3(x) = 9 x + 3 x + 9 x B4(x) = 16 x + 16 x + 8 x ASU-MSD 11 Bernstein Basis Functions Bernstein Basis Functions, n = 3 3 2 k=0: b ; (x) = (1 x) k=1: b ; (x) = 3x(1 x) 3 0 − 3 1 − 2 3 k=2: b ; (x) = 3x (1 x) k=3: b ; (x) = x 3 2 − 3 3 ASU-MSD 12 Bernstein and Lagrange Example (f(x) = Heaviside(x 1 )) − 2 p1 B1(f) p4 B4(f) ASU-MSD 13 Newton Interpolation Newton Basis Polynomials In order to make it easy to add a new knot, we change the set of basis polynomials. Given a set of n + 1 knots, xk , set f g N0(x) = 1 N (x) = (x x ) 1 − 0 N (x) = (x x )(x x ) 2 − 0 − 1 N3(x) = (x x0)(x x1)(x x2) . − − − . Nn(x) = (x x )(x x )(x x ) (x xn− ) − 0 − 1 − 2 ··· − 1 Now let n Pn(x) = akNk(x) k X=0 ASU-MSD 14 The Newton Coefficients Calculating the ak’s For a set of n + 1 data points [xk; yk] , define the (forward) divided differences recursively as f g [y0] = y0 [y ] [y ] [y ; y ] = 1 − 0 0 1 x x 1 − 0 [y ; y ] [y ; y ] [y ; y ; y ] = 1 2 − 0 1 0 1 2 x x . 2 − 0 . Then the Newton interpolating polynomial is Pn(x) = [y ] + [y ; y ](x x ) + [y ; y ; y ](x x )(x x ) 0 0 1 − 0 0 1 2 − 0 − 1 + [y0; y1; y2; y3](x x0)(x x1)(x x2) + ::: n − − − = [y0; : : : ; yk]Nk(x) k X=0 ASU-MSD 15 First Sample A Used Polynomial Let S = f[−2; 1]; [−1; −1]; [0; 1]; [1; −1]; [2; 1]g. Begin by building a difference tableau. x −2 −1 0 1 2 y 1 −1 1 −1 1 [y0] 1 −1 1 −1 1 [y0; y1] −22 −2 2 [y0; y1; y2] 2 −2 2 4 4 [y0; y1; y2; y3] − 3 3 2 [y0; y1; y2; y3; y4] 3 n P Then P4(x) = [y0; : : : ; yk]Nk(x) k=0 4 2 = 1−2(x+2)+2(x+2)(x+1)− 3 (x+2)(x+1)(x)+ 3 (x+2)(x+1)(x)(x−1) 8 2 2 4 P4(x) = 1 − 3 x + 3 x ASU-MSD 16 Second Sample The Heaviside Function 1 2 3 4 Set S = [0; 0]; [ 5 ; 0]; [ 5 ; 0]; [ 5 ; 1]; [ 5 ; 1]; [1; 1] .
Details
-
File Typepdf
-
Upload Time-
-
Content LanguagesEnglish
-
Upload UserAnonymous/Not logged-in
-
File Pages22 Page
-
File Size-