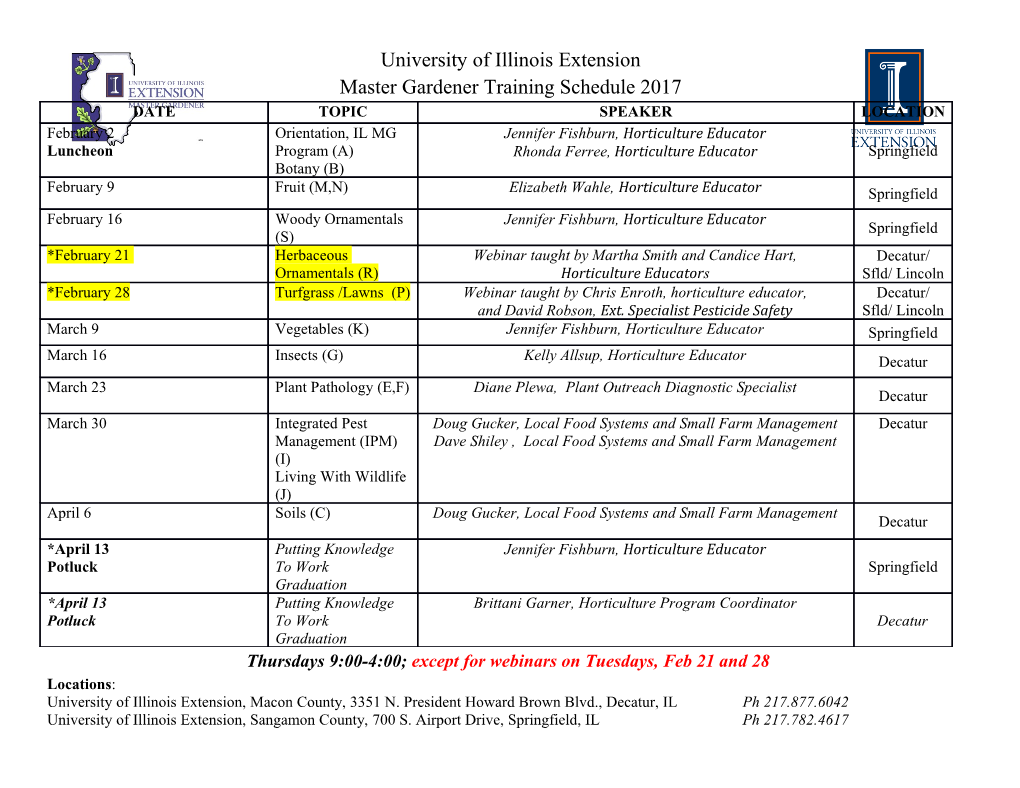
Research Collection Doctoral Thesis Leveraging Data Analytics Towards Activity-based Energy Efficiency in Households Author(s): Cao, Hông-Ân Publication Date: 2017-06 Permanent Link: https://doi.org/10.3929/ethz-b-000000236 Rights / License: In Copyright - Non-Commercial Use Permitted This page was generated automatically upon download from the ETH Zurich Research Collection. For more information please consult the Terms of use. ETH Library Diss. ETH No. 24173 Leveraging Data Analytics Towards Activity-based Energy Efficiency in Households A thesis submitted to attain the degree of DOCTOR OF SCIENCES of ETH ZURICH (Dr. sc. ETH Zurich) presented by Hông-Ân Cao ing. sys. com. dipl. EPF École Polytechnique Fédérale de Lausanne born on February 23, 1987 citizen of Monthey VS, Switzerland accepted on the recommendation of Prof. Dr. Friedemann Mattern, examiner Prof. Dr. Nuno Jardim Nunes, co-examiner Prof. Dr. Torben Bach Pedersen, co-examiner 2017 Có trí thì nên. — Vietnamese proverb To my parents. ABSTRACT Aiming for sustainable development means reconsidering the access to energy sources in industrialized countries, which are not faced with contingency scenarios that are implemented in emergent and newly-developed countries, to allow equal access to energy sources for all and thwart environmental degradation. The global penetration of renewable energy sources to replace fossil fuel and nuclear power plants means adjusting to stochastic energy production. The expected yield will be dependent on very different weather and landscape con- ditions and will represent a challenge for countries with continuous access to energy sources and where energy is often considered a pub- lic utility. Tracking wastage, improving the scheduling, and the pro- cesses that consume energy, would allow us to match the demand and the supply of energy. This will be particularly crucial during peak time, where meeting the high demand incurs the ramping up of mostly unclean additional power plants or introducing power system instability. The digitalization of the energy sector has started with the roll- out of smart meters to record the electricity consumption at a finer granularity and are aimed to replace the biannual or yearly dispatch of utility companies’ employees to read the meter. Considerable re- search efforts have been directed at analyzing aggregated loads from these smart meters or at developing algorithms for disaggregating households’ total electricity consumption to isolate single appliances’ traces. However, less focus has been set on assessing the potential of using sub-metered data for improving the energy efficiency in house- holds. This was primarily linked to the fact that the necessary datasets were not widely available, due to the difficulty and the costs in instru- menting households for acquiring the consumption data from appli- ances. The objective of this thesis is to investigate how to leverage and improve existing disaggregated datasets to develop data-driven techniques to improve the energy efficiency within residential homes. Starting from smart meter data, we segmented households into groups with similar electricity consumption pattern based on their peak consumption, to identify hurtful consumption patterns in the perspective of utility companies, for which they could launch targeted mitigation campaigns. However, improving the energy efficiency in the residential sector requires to change individuals’ relationship to- wards their electricity consumption. These behaviors are closely re- lated to the activities that are carried out throughout the day and can be supported by the usage of consumer electronics, such as appli- ances. Therefore, we turned to analyzing the behaviors inside house- i holds that triggered the usage of electricity by studying a large disag- gregated dataset and developed learning techniques to extract activ- ity patterns. We first addressed the challenge of determining when appliances are actively used by households’ residents, from when they are off or idle and incurring standby consumption by develop- ing GMMthresh, an automatic thresholding method, which is agnos- tic of the appliance’s type, brand and model, but instead relies on the statistical distribution of its power consumption. Due to the lack of event-based and activity labels in existing data- sets to allow us to validate our learning technique, we leveraged crowdsourcing concepts to provide an expert-annotated dataset to en- rich the existing datasets through our Collaborative Annotation Frame- work for Energy Datasets (CAFED). We conducted two in-depth studies to quantify the performance of regular users against expert users in la- beling energy data on CAFED. We provided analysis tools and meth- ods that can be generalized to crowdsourcing systems for improving the quality of the workers’ contributions. Using the expert-annotated labels, we validated GMMthresh with expert manually labeled data. Then, we developed a method for learning temporal association rules for identifying activities involving the usage of appliances within households. Our pipeline includes our thresholding algorithm and a novel search algorithm for determining time windows for the asso- ciation rules efficiently and in a data-driven manner. The contributions of this thesis rely on exploiting energy data and developing novel techniques towards identifying activity patterns and their scheduling, which could then become part of an ambient intel- ligence system that would smarten existing homes. The methods we developed are not restricted to the energy research, as they can be ap- plied to sensor data, where for example inertial sensors also require machine learning algorithms to filter out background noise from ac- tual movement. Similarly, our work on the crowdsourcing of time series opens new perspectives for extending the range of data that can be annotated by the crowd and provides design insights and mit- igation techniques for improving the quality of the labeling on col- laborative platforms. Finally, our temporal association rules mining framework is not limited to energy time series but can be applied to search for temporal windows and understanding the scheduling of any time series dataset. ii RÉSUMÉ Pour permettre le développement durable à tous et éviter les dé- sastres environnementaux, les pays industrialisés doivent reconsidé- rer leurs rapports aux resources énergétiques, bien que n’étant pas actuellement exposés à des plans de contingence comme dans les pays émergents et en voie de développement. La pénétration des éner- gies renouvelables à l’échelle mondiale qui remplaceront à terme les sources fossiles et les centrales nucléaires entraîne un ajustement à la nature stochastique de la production d’énergie propre. Le rende- ment attendu dépendra fortement des conditions météorologiques et de la situation géographique des installations et représentera un défi majeur pour les pays qui ont bénéficié jusqu’à présent d’un accès continu aux sources d’énergie, en faisant de celle-ci une commodité. Identifier les sources de gaspillage, améliorer l’ordonnancement et les processus qui consomment de l’énergie, permettraient de faire correspondre la demande à la production d’énergie. Ceci sera parti- culièrement crucial aux heures de pointe, où répondre à la forte de- mande occassionne l’inclusion de centrales électriques additionnelles souvent polluantes ou le risque d’introduire de l’instabilité dans le réseau électrique. La digitalisation du secteur de l’énergie a commencé avec l’intro- duction des compteurs intelligents qui permettent de mesurer la con- sommation électrique à une granularité plus fine, et ainsi de rem- placer le passage d’employés pour le relevé de compteur semestriel ou annuel. Des efforts considérables de recherche ont été entrepris pour analyser les charges aggrégées enregistrées par ces compteurs intelligents ou pour développer des algorithmes pour désagréger la consommation d’électricité totale des résidences pour en déduire les charges individuelles des appareils électroménagers. Cependant, moins d’accent a été porté à évaluer le potentiel d’exploiter les données ob- tenues des charges individuelles détaillées pour améliorer l’efficacité énergétique des clients résidentiels. Ceci a été au préalable dû au fait que les ensembles de données nécessaires n’étaient pas généralement disponibles, à cause de la difficulté et des coûts pour instrumenter les foyers pour collecter les données de consommation des appareils ménagers. L’objectif de cette thèse et d’examiner comment exploiter et améliorer les ensembles de données désagrégées existants et de dé- velopper des méthodes fondées sur l’analyse de données pour amé- liorer l’efficacité énergétique au sein des foyers. Nous commençons par considérer les données de compteurs intel- ligents pour segmenter les foyers en groupes avec des profils de con- sommation d’électricité similaire, sur la base de leur consommation aux heures de pointes, pour identifier les profils nuisibles aux compa- iii gnies électriques, qui pourront conduire des campagnes de sensibili- sation pour y remédier. Cependant, améliorer l’efficacité energétique dans le secteur résidentiel implique l’adoption de changement de comportement face à sa propre consommation énergétique. Ces com- portements sont étroitement liés aux activités qui ont lieu à travers la journée et qui peuvent inclure l’utilisation d’appareils électroniques, tels que les appareils ménagers. Par conséquent, nous avons entrepris d’analyser les comportements dans les foyers
Details
-
File Typepdf
-
Upload Time-
-
Content LanguagesEnglish
-
Upload UserAnonymous/Not logged-in
-
File Pages169 Page
-
File Size-