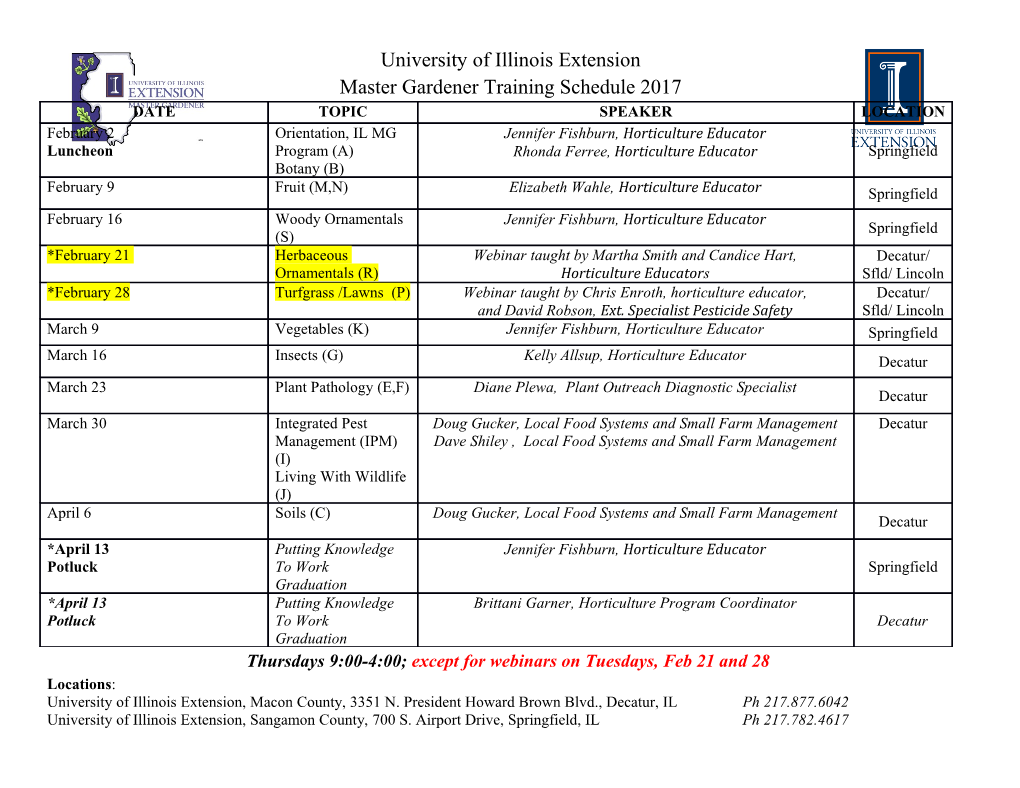
Available online at www.sciencedirect.com ScienceDirect Procedia CIRP 16 ( 2014 ) 3 – 8 Product Services Systems and Value Creation. Proceedings of the 6th CIRP Conference on Industrial Product-Service Systems Service innovation and smart analytics for Industry 4.0 and big data environment Jay Lee*, Hung-An Kao, Shanhu Yang NSF I/UCRC Center for Intelligent Maintenance Systems (IMS), University of Cincinnati, Cincinnati, OH 45221-0072, USA * Corresponding author. Tel.: +1-513-556-3412; fax: +1-513-556-4647. E-mail address: [email protected] Abstract Today, in an Industry 4.0 factory, machines are connected as a collaborative community. Such evolution requires the utilization of advance- prediction tools, so that data can be systematically processed into information to explain uncertainties, and thereby make more “informed” decisions. Cyber-Physical System-based manufacturing and service innovations are two inevitable trends and challenges for manufacturing industries. This paper addresses the trends of manufacturing service transformation in big data environment, as well as the readiness of smart predictive informatics tools to manage big data, thereby achieving transparency and productivity. © 2014 TheElsevier Authors. B.V. Pub Thislished is an by open Elsevier access B.V. article under the CC BY-NC-ND license Selection(http://creativecommons.org/licenses/by-nc-nd/3.0/ and peer-review under responsibility of the Internationa). l Scientific Committee of “The 6th CIRP Conference on Industrial Product- ServiceSelection Systems” and peer-review in the person under of the responsibility Conference ofChair the ProfessorInternational Hoda Scientifi ElMaraghy. c Committee of “The 6th CIRP Conference on Industrial Product-Service Systems” in the person of the Conference Chair Professor Hoda ElMaraghy” Keywords: Manufacturing servitization; predictive maintenance; industrial big data 1. Introduction machines, and consequently improves overall performance and maintenance management. Although the autonomous In today’s competitive business environment, companies computing methodology has been implemented successfully in are facing challenges in dealing with big data issues of rapid computer science, self-learning machines are still far from decision-making for improved productivity. Many implementation in current industries. Transformation from manufacturing systems are not ready to manage big data due today’s status into more intelligent machines requires further to the lack of smart analytic tools. Germany is leading a advancement in the science by tackling several fundamental transformation toward 4th Generation Industrial Revolution issues. These issues can be divided into five distinct categories (Industry 4.0) based on Cyber-Physical System-enabled as follows: manufacturing and service innovation. As more software and embedded intelligence are integrated in industrial products • Manager and Operator Interaction: Currently, operators and systems, predictive technologies can further intertwine control machines, managers design logistic schedules and intelligent algorithms with electronics and tether-free machines are only performing the assigned tasks. Although intelligence. These technologies will then be used to predict these tasks are usually optimized by expert operators and product performance degradation, and autonomously manage managers, a significantly important factor is missing in and optimize product service needs. these decisions: the health condition of the machine Nowadays, smart factories focus mostly on control-centric components. optimization and intelligence. Moreover, greater intelligence can be achieved by interacting with different surrounding • Machine Fleet: It is very common that similar or identical systems that have a direct impact to machine performance. machines (machine fleet) are being exposed to completely Achieving such seamless interaction with surrounding systems different working conditions for different tasks. In contrast, turns regular machines into self-aware and self-learning most predictive and prognostic methods are designed to 2212-8271 © 2014 Elsevier B.V. This is an open access article under the CC BY-NC-ND license (http://creativecommons.org/licenses/by-nc-nd/3.0/). Selection and peer-review under responsibility of the International Scientific Committee of “The 6th CIRP Conference on Industrial Product-Service Systems” in the person of the Conference Chair Professor Hoda ElMaraghy” doi: 10.1016/j.procir.2014.02.001 4 Jay Lee et al. / Procedia CIRP 16 ( 2014 ) 3 – 8 support a single or limited number of machines and section, previous research on these two topics will be working conditions. Currently, available prognostic and reviewed and discussed. health management methods are not taking advantage of considering these identical machines as a fleet by gathering 2.1. Manufacturing servitization and innovation worthwhile knowledge from different instances. Many advanced countries whose economic base is the • Product and Process Quality: As the final outcome of the manufacturing industry have made efforts to transform their manufacturing process, product quality can provide much economy and reinvigorate the industry. They suffer threats insight on machine condition via backward reasoning from emerging markets and the global manufacturing supply algorithms. Product quality can provide feedback for chain. Therefore, manufacturing firms not only seek system management, which can be used to improve manufacturing technique innovation, but are also beginning to production scheduling. Currently, such feedback loop does focus on induction and impetus of service. This way, the not exist and needs further research. fuzzy boundary of the manufacturing industry and service industry drive will stimulate the development of • Big Data and Cloud: Data management and distribution in manufacturing servitization. Big Data environment is critical for achieving self-aware Servitization was proposed by Vandermerve and Rada in and self-learning machines. The importance of leveraging 1988 [1]. They emphasized the concept of customer focus; additional flexibility and capabilities offered by cloud combining products, services, support, and knowledge are the computing is inevitable, but adapting prognostics and most important elements. Furthermore, the authors also health management algorithms to efficiently implement asserted that not only service industries, but also current data management technologies requires further manufacturing industries should focus on innovative value- research and development. added service development in order to quickly enhance their core competencies. Baines defined manufacturing • Sensor and Controller Network: Sensors are the machine’s servitization as innovation of organizational capabilities and gateway to sense its surrounding physical environment. processes, from product sales to integrated product services However, sensor failure and degradation may pass wrong [2]. and inaccurate readings to decision-making algorithms, Servitization is defined as the strategic innovation of an which will result in an incorrect outcome. organization’s capabilities and processes to shift from selling products, to selling an integrated product and service offering With these issues in mind, the objective of the paper is to that delivers value in use, i.e. a Product-Service System [3]. review how current manufacturing industries evolve for the The concept of a Product Service-System (PSS) is a special upcoming industrial big data environment, and propose the case of servitization. Mont defines PSS as a system of key technology for sustainable innovative service. The paper products, services, supporting networks, and infrastructure is organized as follows: Section 2 focuses on trends of service that is designed to be competitive, satisfy customers' needs, innovation in manufacturing industries and unmet needs of an and have a lower environmental impact than traditional Industry 4.0 factory; Section 3 describes the proposed self- business models [4]. In the PSS business model, industries aware and self-maintenance machine systems based on develop products with value-added services, instead of a industrial big data analysis; Section 4 presents two case single product itself, and provide their customers with studies that have been conducted to demonstrate the services that are needed. In this relationship, the market goal feasibility of the proposed framework; and Section 5 of manufacturers is not one-time product selling, but concludes the paper with some perspectives. continuous profit from customers by total service solution, which can satisfy unmet customers’ needs. 2. Trends and unmet needs for Industry 4.0 era 2.2. Industrial big data environment The discovery of new technologies has escorted industry development from the early adoption of mechanical systems, Recently, big data becomes a buzzword on everyone’s to support production processes, to today’s highly automated tongue. It has been in data mining since human-generated assembly lines, in order to be responsive and adaptive to content has been a boost to the social network. It has also been current dynamic market requirements and demands. Under the called the web 2.0 era since late 2004 [5]. Lots of research Industry 4.0 concept, astounding growth in the advancement organizations and companies have devoted themselves to this and adoption of information technology and social media new research topic, and most of them focus on social or networks
Details
-
File Typepdf
-
Upload Time-
-
Content LanguagesEnglish
-
Upload UserAnonymous/Not logged-in
-
File Pages6 Page
-
File Size-