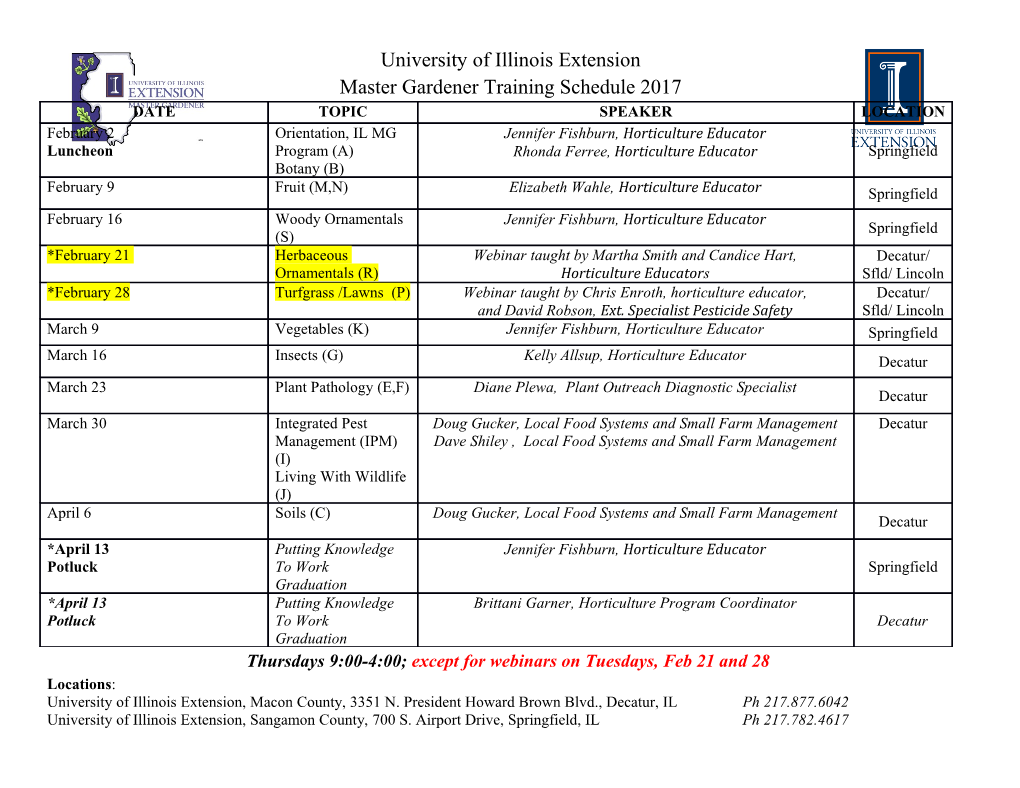
Evolutionary Computation Evolutionary Computation Tutorial: Multi-objective optimisation implementation using Java Shan He School for Computational Science University of Birmingham Modules 02411 and 22313: EC Evolutionary Computation Outline Outline of Topics Existing EA/MOEA libraries and toolboxes Java MOEA framework How to Evaluate MOEAs? Notable Companies used Evolutionary Computation Conclusion Evolutionary Computation Existing EA/MOEA libraries and toolboxes Existing EA/MOEA frameworks and toolboxes I Matlab I Matworks Global Optimisation Toolbox I Genetic Algorithm Optimization Toolbox (GAOT) I Java I ECJ: Java Evolutionary Computation Toolkit I Watchmaker Framework for Evolutionary Computation I JGAP: Java Genetic Algorithm Package I MOEA framework I C/C++ I ParadisEO I Evolving Objects (EO): an Evolutionary Computation Framework I Open BEAGLE I Python I PyGMO I DEAP I inspyred: Bio-inspired Algorithms in Python Evolutionary Computation Java MOEA framework What is MOEA framework I \A free and open source Java library for developing and experimenting with multiobjective evolutionary algorithms (MOEAs) and other general-purpose multiobjective optimization algorithms" from MOEA framework web page I Many state-of-the-art multiobjective evolutionary algorithms are included: I Genetic algorithms: NSGA-II I Differential evolution I Particle swarm optimization I Genetic programming and Grammatical evolution I Other features: I Easy to use: minimum effor to define a problem, a algorithm and a experiment I Modular design: constructing new optimization algorithms from existing components I Very good documentation Evolutionary Computation Java MOEA framework How to use MOEA? I Download the compiled binaries from Sourceforge I Unzip and add libraries to Eclipse I To define a new problem, extend the AbstractProblem class I Use Executor, Instrumenter and Analyzer classes I Executor: class for constructing and executing runs of an algorithm I Instrumenter: class for analyzing the performance of algorithms I Analyzer: class for analysing the resulting Pareto approximation set and how it compares against a known reference set. Evolutionary Computation Java MOEA framework Example: multi-objective optimization in structural design Problem: design of a four bar plan truss with two objectives: I Minimise the volume of the truss I Minimise its joint displacement Evolutionary Computation Java MOEA framework Example: multi-objective optimization in structural design Mathematical formulation: ( p p f1(x) = L(2x1 + 2x2 + x3 + x4) min p p f (x) = FL ( 2 + 2 2 − 2 2 + 2 ) 2 E x1 x2 x3 x4 such that: p(F /σ) ≤ x1 ≤ 3(F /σ) 2(F /σ) ≤ x ≤ 3(F /σ) p 2 (1) 2(F /σ) ≤ x3 ≤ 3(F /σ) (F /σ) ≤ x4 ≤ 3(F /σ) where xi , i = 1; 2; 3; 4 are the cross sectional areas of the four bars, the acting force F = 10kN, Young's modulus of elasticity E = 2 × 105 kN/cm2, the length L = 200cm, the only nonzero stress component σ = 10kN/cm2. Evolutionary Computation How to Evaluate MOEAs? MOEAs Test Functions I ZDT benchmark: 6 problems, e.g., ZDT1 - ZDT6 I DTLZ benchmark: 7 problems, e.g., DTLZ1 - DTLZ7 I WFG benchmark: 9 problems, e.g., WFG1 - WFG9 I CEC2009 competition benchmark: 10 problems, e.g., UF1 - UF10 I One example: ZDT1: f1 = x1 q f = g · 1:0 − f1 2 g (2) 9 Pn g(x2; ··· ; xn) = 1:0 + n−1 i=2 xi 0 ≤ xi ≤ 1; i = 1; ··· ; n Evolutionary Computation How to Evaluate MOEAs? MOEAs Test Functions: ZDT1 Pareto Front of ZDT1: Evolutionary Computation How to Evaluate MOEAs? Multiobjective optimisation performance assessment I We need to design performance metrics or indicators to evaluate the algorithms' performance. I What characteristics should good Pareto optimal solutions have: I Minimize the distance of the Pareto front produced by the algorithm with respect to the true Pareto front if it is known I Maximize the spread of solutions found, so that we can have a distribution of vectors as smooth and uniform as possible. I Maximize the amount of elements of the Pareto optimal set found. I A myriad of ways of metrics, but can be classified as three kinds for measuring: I Convergence: converge to true Pareto front I Spread: maintain diversity of solution mainly in objective space I Both Convergence and Spread Evolutionary Computation How to Evaluate MOEAs? Multiobjective optimisation performance assessment I A few popular indicators I The hypervolume indicator: defines the size of multidimensional space that a set of solutions cover, without counting the same area twice. I Spread: calculates how uniformly spread across the objective space the solutions are within their set. I The cover function: calculates the proportion of one solution set that is dominated by another set. Thus, if C(X ; Y ) = 0:9, 90% of solutions in Y are dominated by solutions in X. I The Purity function: unifies the solutions returned by a set of algorithms on a particular problem, and returns the proportion of each that remain nondominated in the set. I A comprehensive indicators are listed in this paper: Yen, G.G. and He, Z.N. Performance Metric Ensemble for Multiobjective Evolutionary Algorithms, IEEE Trans on Evolutionary Computation, 18(1), Feb. 2014 (link to the paper) Evolutionary Computation How to Evaluate MOEAs? Example: the hypervolume indicator I Also called S metrics, essentially calculates how much of the objective space is dominated by a given nondominated set A I Formal definition: Let X be set of decision vectors for the considered problem and A = fx1; x2;; xtg 2 X a set of t decision vectors. The hypervolume indicator or S metrics S(A) gives the volume enclosed by the union of the polytopes p1; p2; ··· ; pt , where each pi is formed by the intersection of the following hyperplanes arising out of xi, along with the axes: for each axis in the objective space there exist a hyperplane perpendicular to the axis and passing through the point (f1(xi ); f2(xi ); ··· ; fk (xi )). Evolutionary Computation How to Evaluate MOEAs? Example: the hypervolume indicator Pareto Front of ZDT1: f1(x) Minimising f1(x) and f2(x) A B C PA D E PC PD PE f2(x) Evolutionary Computation Notable Companies used Evolutionary Computation Notable Companies used Evolutionary Computation I SolveIT Software: I Founded by Zbigniew Michalewicz, author of Genetic Algorithms + Data Structures I Uses Genetic Algorithm, PSO and other metaheurstic algorithms for advanced planning and scheduling optimization I Widely used in industries of wine, grain handling and mining such as Rio Tinto Group and BHP Billiton I 150 employees and 5 international offices I Acquired by Schneider Electric in 2012 but price was not divulged I Their Hybrid Optimisation System I Red Cedar Technology I DecisionVis Evolutionary Computation Conclusion Conclusion I Use library to solve your optimisation problems I Use performance metrics to evaluate your algorithm and solutions.
Details
-
File Typepdf
-
Upload Time-
-
Content LanguagesEnglish
-
Upload UserAnonymous/Not logged-in
-
File Pages15 Page
-
File Size-