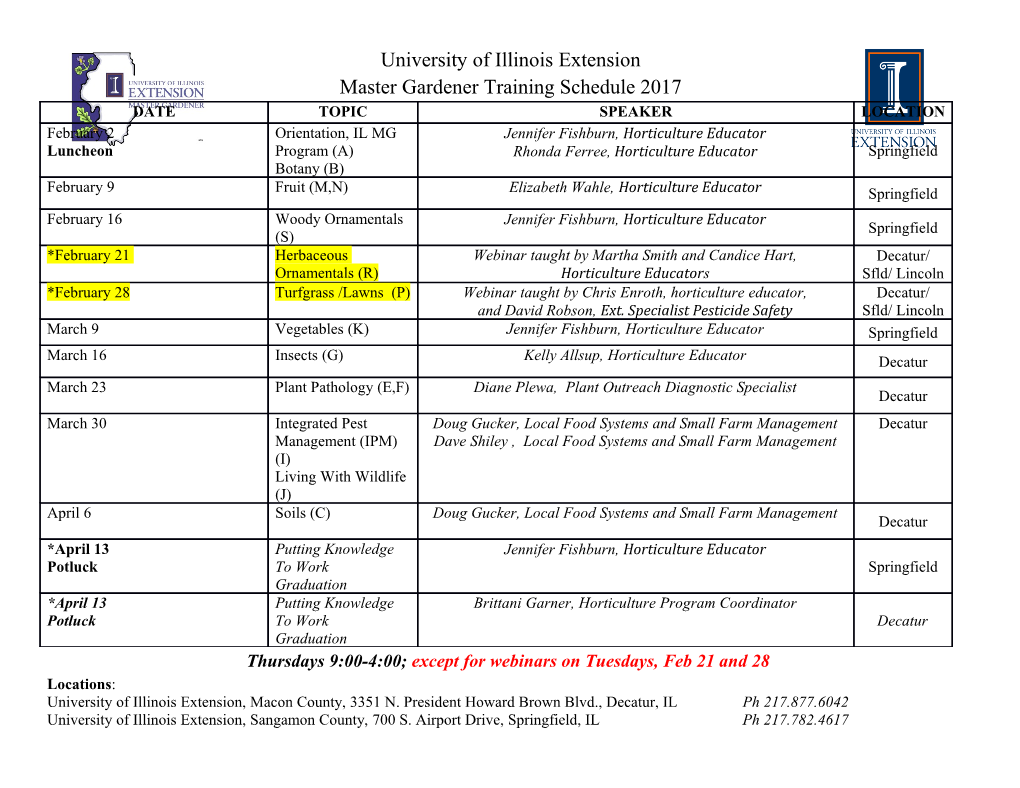
Maximum Likelihood Attitude and Position Estimation from Pseudo-Range Measurements using Geometric Descent Optimization A. Alcocer, P. Oliveira, A. Pascoal, and J. Xavier Landmark Abstract— This paper addresses the problem of estimating j Beacon i, bias ci the attitude and the position of a rigid body when the available measurements consist only of pseudo-ranges between a set of body fixed beacons and a set of earth fixed landmarks. To this dij effect, a Maximum Likelihood (ML) estimator is formulated as an optimization problem on the parameter space Θ = b Rp i SE(3) × corresponding to the attitude and position of the pj rigid body as well as a set of biases present in the pseudo-range equations. Borrowing tools from optimization on Riemannian {B} p manifolds, intrinsic gradient and Newton-like algorithms are derived to solve the problem. The rigorous mathematical set- up adopted makes the algorithms conceptually simple and elegant; furthermore, the algorithms do not require the ar- tificial normalization procedures that are recurrent in other {I} R estimation schemes formulated in Euclidean space. Supported by recent results on performance bounds for estimators on Riemannian manifolds, the Intrinsic Variance Lower Bound Fig. 1. Geometry of estimation problem. (IVLB) is derived for the problem at hand. Simulation results are presented to illustrate the estimator performance and to validate the tightness of the IVLB in a wide range of signal to noise ratio scenarios. It is also common to find in the literature work addressing Index Terms— Navigation systems, attitude/positioning sys- tems, maximum likelihood estimation, optimization on Rieman- the problem of estimating the attitude (that is the relative nian manifolds orientation of a reference frame with respect to another reference frame) with vector observations [2],[3]. In some I. INTRODUCTION applications, vector observations can be obtained from range Joint attitude and position estimation systems based on observations by making the planar waveform appoximation pseudo-range measurements are becoming popular and have [4],[5]. Despite the fact that the attitude and positioning received the attention of the research community as an alter- problems are strongly coupled, a simultaneous treatment of native to more complex, expensive, and sophisticated Inertial the problem is seldom encountered (see [6] for an example Navigation Systems. An advantage of such systems is that based on line of sight measurements). they are drift-less and insensible to magnetic disturbances. This paper addresses the problem of simultaneous attitude Examples of applications include multiple GPS receiver and position estimation with pseudo-range measurements. systems, indoor wireless network positioning systems, and To this effect, a Maximum Likelihood (ML) estimator is acoustic systems to determine the attitude/position of a body formulated by solving an optimization problem on a param- underwater. Range measurements are usually obtained by eter space containing the attitude and the position of the measuring the time it takes an electromagnetic or acoustic rigid body as well as a set of bias terms present in the signal to travel between an emitter and a receiver given pseudo-range equations. Intrinsic gradient and Newton-like that the speed of propagation of the signals is assumed algorithms are derived borrowing tools from optimization to be known. Pseudo-range measurements arise when the on Riemannian manifolds. The rigorous mathematical set- emitter and receiver are not synchronized, resulting in an up adopted makes the algorithms conceptually simple and unknown bias term in the range measurements. The range elegant. Furthermore, the algorithms do not require artificial only positioning problem has been extensively treated in the normalization procedures that are recurrent in other estima- literature, see for example [1] and the references therein. tion schemes formulated in Euclidean space. The authors are with Instituto Superior Técnico, Institute for Systems II. PROBLEM FORMULATION and Robotics, Av. Rovisco Pais 1, 1049-001 Lisbon, Portugal. E-mail: Suppose that one is interested in estimating the configura- {alexblau,pjcro,antonio,jxavier}@isr.ist.utl.pt This work was partially supported by Fundação para a Ciência e a tion (that is, position and attitude) of a rigid body in space. Tecnologia (ISR-IST plurianual funding) through the POS-Conhecimento Define a reference frame attached to the rigid body and Program that includes FEDER funds and by projects RUMOS of the an Inertial reference frame{B} . The position of the origin FCT, MAYA of the AdI, and IST-GREX (Contract No. 035223) of the of with respect to {I}can be represented by vector Commission of the European Communities. The work of the first author {B} {I} was supported by a PhD Scholarship from the FCT. p R3, and the relative orientation between and ∈ {B} {I} can be represented by a rotation matrix SO(3), where body beacons can suffer from highly correlated disturbances. SO(3) is the Special Orthogonal group definedR ∈ as This might be due to the fact that the observations originate from signals that have travelled almost through the same SO(3) = R3×3 : T = I , det( ) = 1 . (1) R ∈ R R 3 R propagation channel. In these cases the covariance matrix R is close to being block diagonal. In the above expression I3 stands for the 3 3 identity matrix and det( ) is the matrix determinant operator.× The In order to determine the attitude and the position of the configuration space· of the rigid body can then be identified rigid body from the pseudo-range measurements, the vector p R3 of unknown biases c R needs also to be estimated. It is with the Special Euclidean group SE(3) = SO(3) (as ∈ a set). Elements of SE(3) will be identified with× the pair common in the literature to avoid estimating the biases by T ( , p) or with the vector vec( )T pT (where vec( ) is subtracting pseudo-range equations among them to obtain theR operator that stacks the columnsR of a matrix from left· to range-differences. This approach will not be pursued in this right) depending on what representation is more suitable for paper. Instead, an augmented parameter space Θ will be the computations. considered containing the unknown bias terms. The final parameter space will be defined as the Cartesian product Suppose the rigid body has p beacons and assume that Rp R3 Rp the location of the beacons with respect to is known. Θ = SE(3) = SO(3) . {B} × × × The beacons could be GPS antennas, or underwater acoustic A. ML Estimator Formulation transponders, arranged with a certain known geometry in the The Maximum Likelihood (ML) Estimator determines rigid body. Let us further consider that there are m fixed the triad ( , p, c) Θ that maximizes the likelihood landmarks distributed in the ambient space with known posi- ML function, thatR is, the probability∈ p (y , p, c) of obtaining tions: for example, GPS satellites or surface buoys equipped |R the observationsb y given the parameters ( , p, c) [7] [8]. Ac- with hydrophones. Let b R3, i 1,...,p denote the b b i cording to the Gaussianity assumption onR the disturbances, positions of the p beacons in∈ the rigid∈ body { expressed} in the ML estimator can be found by solving the optimization and let p R3,j 1,...,m denote the positions of{B} the j problem m landmarks∈ expressed∈ { in }(see Fig. 1). {I} Let dij denote the distance between the i’th beacon and , p, c = arg min f( , p, c) (6) the j’th landmark. Let c R; i 1, ,p be an unknown R ML (R,p,c)∈ Θ R i beacon dependent bias term.∈ Then∈ { · the · · pseudo-ranges} are R where f :Θb b bis given by defined as → 1 f( , p, c) = (y ρ)T R−1(y ρ) (7) ρij = dij + ci = bi + p pj + ci (2) R 2 − − kR − k and, from (2), ρ = ρ( , p, c). Note that the cost function f with i 1,...,p , j 1,...,m , and stands is not differentiable whenR some of the ranges d vanish, that for the∈ Euclidean { norm.} The∈ { observations} y ,k defined · k by ij ij is, when the position of a beacon coincides with the position y = ρ + w consist of the pseudo-ranges ρ corrupted ij ij ij ij of a landmark. It is realistic to assume that this situation by an additive zero mean Gaussian disturbances w . Note ij never occurs in practice. that the terms beacon and landmark are used to illustrate the problem but they do not impose any particular role (in III. OPTIMIZATION ON RIEMANNIAN terms of emitter/receiver). They should be simply viewed as SUBMANIFOLDS pseudo-range measuring devices. In order to determine the ML Estimator, the function It is convenient to stack all the ij elements in a more f needs to be minimized over the parameter space Θ. At compact form by defining the vectors this point, it is not obvious how to continue and effectively T mp solve this constrained optimization problem. The problem d , [d dp ] [d m dpm] R , (3) 11 · · · 1 · · · 1 · · · ∈ does not admit a closed form solution so it is necessary to h i resort to an iterative scheme. There has been some work and ρ, y, w Rmp in a similar way. Define also a vector T on generalizing the classic gradient descent and Newton c = [c ∈c ] Rp containing all the bias terms. With 1 p methods to Riemannian manifolds [9] [10]. The key idea this arrangement,· · · the∈ observations can then be written in a involved in [9] is to generalize the classic gradient and more compact form as Newton-like directions and to perform line searches along ρ = d + (1m Ip)c (4) geodesics, as depicted in Fig.
Details
-
File Typepdf
-
Upload Time-
-
Content LanguagesEnglish
-
Upload UserAnonymous/Not logged-in
-
File Pages6 Page
-
File Size-